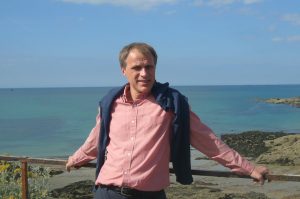
This post is devoted to a concentration inequality of Lipschitz functions for a class of projected probability distributions on the unit sphere of $\mathbb{R}^n$, $n\geq2$, \[ \mathbb{S}^{n-1}=\Bigr\{x\in\mathbb{R}^n:|x|:=\sqrt{x_1^2+\cdots+x_n^2}=1\Bigr\}. \] We take this opportunity to recall various aspects of concentration for Gaussians.
Concentration. Let us consider a random vector $X$ of $\mathbb{R}^n$, $n\geq2$, and its Euclidean norm $|X|:=\sqrt{X_1^2+\cdots+X_n^2}$. Suppose that for all $F:\mathbb{R}^n\to\mathbb{R}$ Lipschitz, the law of the random variable $F(X)$ has sub-Gaussian concentration, in the sense that for all $r\geq0$, \begin{equation*} \mathbb{P}(|F(X)-\mathbb{E}F(X)|\geq r) \leq c\exp\Bigr(-\frac{r^2}{2C\|F\|_{\mathrm{Lip.}}^2}\Bigr), \end{equation*} for some constants $c,C > 0$. Then for all $F:\mathbb{R}^n\to\mathbb{R}$ Lipschitz and $r\geq\sigma\|F\|_{\mathrm{Lip.}}$, \begin{align*} \mathbb{P}\Bigr( \Bigr|F\Bigr(\frac{X}{|X|}\Bigr)-\mathbb{E}F\Bigr(\frac{X}{|X|}\Bigr)\Bigr| \geq r \Bigr) &\leq2c\exp\Bigr(-\frac{\mu^2}{8C}\Bigr(\frac{r}{\|F\|_{\mathrm{Lip.}}}-\sigma\Bigr)^2\Bigr) \end{align*} where \[ \mu:=\mathbb{E}|X| \quad\text{and}\quad \sigma:=\mathbb{E}\Bigr|\frac{|X|}{\mu}-1\Bigr|. \] The quantity $\|F\|_{\mathrm{Lip.}}:=\sup_{x,y\in\mathbb{R}^n:x\neq y}\frac{|F(x)-F(y)|}{|x-y|}$ is the Lipschitz norm of $F$ with respect to the Euclidean norm on $\mathbb{R}^n$, and not with respect to the geodesic distance on $\mathbb{S}^{n-1}$.
The statement is dilation invariant, in the sense that if we replace $X$ by $\lambda X$ for some constant $\lambda > 0$, the left hand side is invariant, and the right hand side is also invariant, since $\mu$ and $C$ are replaced by $\lambda\mu$ and $\lambda C$ while $\sigma$ is invariant.
Since $X/|X|$ takes its values in the unit sphere $\mathbb{S}^{n-1}$, which has diameter $2$, we have $\bigr|F\bigr(\frac{X}{|X|}\bigr)-\mathbb{E}F\bigr(\frac{X}{|X|}\bigr)\bigr|\leq2\|F\|_{\mathrm{Lip.}}$, it follows that $\mathbb{P}\bigr( \bigr|F\bigr(\frac{X}{|X|}\bigr)-\mathbb{E}F\bigr(\frac{X}{|X|}\bigr)\bigr| \geq r \bigr)=0$ if $r > 2\|F\|_{\mathrm{Lip.}}$, as a consequence, the concentration inequality is useless when $r > 2\|F\|_{\mathrm{Lip.}}$.
Projected normal laws. Consider the Gaussian case $X\sim\mathcal{N}(m,\Sigma)$, $m\in\mathbb{R}^n$, $\Sigma\in\mathrm{Sym}^+_{n\times n}(\mathbb{R})$. Then the sub-Gaussian concentration of Lipschitz functions holds with \[ c=2 \quad\text{and}\quad C=\|\Sigma\|_{\mathrm{op.}}=\max_{|x|=1}\langle\Sigma x,x\rangle. \] The law of $X/|X|$ is known as the projected normal distribution on $\mathbb{S}^{n-1}$.
Let us further specialize to the isotropic case $(m,\Sigma)=(0,I_n)$. Then the law of $X/|X|$ is uniform, moreover $\|\Sigma\|_{\mathrm{op.}}=1$, $X_1,\ldots,X_n$ are i.i.d. $\mathcal{N}(0,1)$, and \[ \mu =\mathbb{E}(\chi(n)) =\sqrt{2}\frac{\Gamma(\frac{n+1}{2})}{\Gamma(\frac{n}{2})} \sim_{n\to\infty}\sqrt{n} \quad\text{while}\quad \sigma\sim_{n\to\infty}\frac{1}{\sqrt{\pi n}}, \] and these asymptotic estimates correspond to LLN and CLT for $X_1^2+\cdots+X_n^2$ and express the thin-shell phenomenon for the isotropic log-concave distribution $\mathcal{N}(0,I_n)$.
Proof. The map $x\mapsto x/|x|$ is $1$-Lipschitz outside the unit ball, but is not Lipschitz at the origin. Instead of trying to compose functions, the idea is that since the real random variable $|X|$ is a Lipschitz function of $X$, it concentrates around its mean $\mu$, making $X/|X|$ close to $X/\mu$, which is a dilation of $X$, and which concentrates in turn! Let us follow this idea.
The concentration inequality, used with the $1$-Lipschitz function $\left|\cdot\right|$, gives, for all $\rho > 0$, \[ \mathbb{P}(||X|-\mu|\geq\rho \mu) \leq c\exp\Bigr(-\frac{\rho^2\mu^2}{2C}\Bigr). \] Next, to concentrate $F(X/|X|)$, we note that by replacing $r$ with $r/\|F\|_{\mathrm{Lip.}}$, we can assume without loss of generality that $\|F\|_{\mathrm{Lip.}}=1$. Now, on the event $A_\rho=\{||X|-\mu| < \rho \mu\}$, \[ \Bigr|F\Bigr(\frac{X}{|X|}\Bigr)-F\Bigr(\frac{X}{\mu}\Bigr)\Bigr| \leq|X|\frac{||X|-\mu|}{|X|\mu} =\frac{||X|-\mu|}{\mu} \leq\rho. \] Moreover, we have \[ \Bigr|\mathbb{E}F\Bigr(\frac{X}{|X|}\Bigr)-\mathbb{E}F\Bigr(\frac{X}{\mu}\Bigr)\Bigr| \leq\mathbb{E}\Bigr|F\Bigr(\frac{X}{|X|}\Bigr)-F\Bigr(\frac{X}{\mu}\Bigr)\Bigr| \leq\sigma. \] Hence, on the event $A_\rho$, \[ \Bigr|F\Bigr(\frac{X}{|X|}\Bigr)-\mathbb{E}F\Bigr(\frac{X}{|X|}\Bigr)\Bigr| \leq \Bigr|F\Bigr(\frac{X}{\mu}\Bigr)-\mathbb{E}F\Bigr(\frac{X}{\mu}\Bigr)\Bigr| +\bigr(\rho+\sigma\bigr). \] Therefore, for all $r\geq(\rho+\sigma)$, using the concentration for $F(X/\mu)$, \begin{align*} \mathbb{P}\Bigr( \Bigr|F\Bigr(\frac{X}{|X|}\Bigr)-\mathbb{E}F\Bigr(\frac{X}{|X|}\Bigr)\Bigr| \geq r \Bigr) &\leq \mathbb{P}(A_\rho^c)+\mathbb{P}\Bigr( \Bigr|F\Bigr(\frac{X}{\mu}\Bigr)-\mathbb{E}F\Bigr(\frac{X}{\mu}\Bigr)\Bigr| \geq r-(\rho+\sigma) \Bigr)\\ &\leq c\exp\Bigr(-\frac{\rho^2}{2C}\mu^2\Bigr) +c\exp\Bigr(-\frac{(r-(\rho+\sigma))^2}{2C}\mu^2\Bigr). \end{align*} It remains to select or optimize over $\rho$. Let us take $\rho=r-(\rho+\sigma)$, which gives $\rho=\frac{1}{2}(r-\sigma)$, which satisfies $r\geq(\rho+\sigma)$. This gives, for all $r\geq\sigma$, \begin{align*} \mathbb{P}\Bigr( \Bigr|F\Bigr(\frac{X}{|X|}\Bigr)-\mathbb{E}F\Bigr(\frac{X}{|X|}\Bigr)\Bigr| \geq r \Bigr) &\leq2c\exp\Bigr(-\frac{\mu^2(r-\sigma)^2}{8C}\Bigr). \end{align*}
Uniform case. If the law of $X$ is rotationally invariant, for instance when $X\sim\mathcal{N}(0,I_n)$, then $U:=X/|X|$ follows the uniform distribution on $\mathbb{S}^{n-1}$, and the concentration inequality of Milman and Schechtman states that for all $F:\mathbb{S}^{n-1}\to\mathbb{R}$ and $r\geq0$, \[ \mathbb{P}(|F(U)-\mathbb{E}F(U)|\geq r) \leq2\exp\Bigr(-\frac{nr^2}{2\|F\|_{\mathrm{Lip.}}^2}\Bigr), \] where the Lipschitz constant is with respect to the geodesic distance on $\mathbb{S}^{n-1}$.
Covariance representation for the Gaussian. Let us consider $X\sim\mathcal{N}(0,I_n)$ in $\mathbb{R}^n$. Then, for all $F,G:\mathbb{R}^n\to\mathbb{R}$ such that $\||\nabla F|\|_\infty < \infty$ and $\||\nabla G|\|_\infty < \infty$, and all $\alpha\in[0,1]$, the following elementary covariance representation holds: \[ \mathbb{E}F(X)G(X)-\mathbb{E}F(X)\mathbb{E}G(X) =\int_0^1\mathbb{E}\langle(\nabla F)(X_\alpha),(\nabla G)(Y_\alpha)\rangle\mathrm{d}\alpha \] where \[ \begin{pmatrix}X_\alpha\\Y_\alpha\end{pmatrix} \sim\mathcal{N}\Bigr(0, \begin{pmatrix} I_n & \alpha I_n\\ \alpha I_n & I_n \end{pmatrix}\Bigr). \] Equivalently, we could use $(X_\alpha,Y_\alpha):=(X,\alpha X+\sqrt{1-\alpha^2}Y)$ with $Y$ independent copy of $X$ and $\alpha=\mathrm{e}^{-t}$, which is the Mehler formula for the Ornstein-Uhlenbeck process.
Following [H], this can be proved by interpolation as $\alpha$ runs over $[0,1]$. We could use the Ornstein-Uhlenbeck semigroup, but there is something simpler. Indeed, $X_1=Y_1$ has the law of $X$, while $X_0$ and $Y_0$ are independent and have both the law of $X$. Thus \begin{align*} \mathbb{E}F(X)G(X)-\mathbb{E}F(X)\mathbb{E}G(X) &=\mathbb{E}F(X_1)G(Y_1)-\mathbb{E}F(X_0)G(Y_0)\\ &=\int_0^1\partial_\alpha\mathbb{E}F(X_\alpha)G(Y_\alpha)\mathrm{d}\alpha. \end{align*} By approximation and bilinearity, it suffices to consider the case of trigonometric monomials, namely characteristic functions : $F(x)=\mathrm{e}^{\mathrm{i}\langle u,x\rangle}$ and $G(x)=\mathrm{e}^{\mathrm{i}\langle v,x\rangle}$, $u,v\in\mathbb{R}^n$. In this case \[ \mathbb{E}F(X_\alpha)G(Y_\alpha) =\exp\Bigr(\frac{1}{2}\Bigr(|u|^2+2\alpha\langle u,v\rangle+|v|^2\Bigr)\Bigr). \] Now it simply remains to note, using $\nabla F(x)=\mathrm{i}u\mathrm{e}^{\mathrm{i}\langle u,x\rangle}$ and $\nabla G(x)=\mathrm{i}v\mathrm{e}^{\mathrm{i}\langle v,x\rangle}$, that \[ \partial_\alpha\mathbb{E}F(X_\alpha)G(Y_\alpha) =\langle u,v\rangle\mathbb{E}F(X_\alpha)G(Y_\alpha) =\mathbb{E}\langle\nabla F(X_\alpha),\nabla G(Y_\alpha)\rangle. \] Denoting $f_\alpha(x,y)$ the density of $(X_\alpha,Y_\alpha)$, this writes, using integration by parts, \[ \partial_\alpha f_\alpha(x,y) =\sum_{k=1}^n\partial^2_{x_k,y_k}f_\alpha(x,y). \]
Concentration from covariance representation. Following [BGH, H], the covariance representation implies sub-Gaussian concentration of Lipschitz functions. Let $F:\mathbb{R}^n\to\mathbb{R}$ be such that $\||\nabla F|\|_\infty\leq1$ and $\mathbb{E}F(X)=0$. The covariance representation used for $F$ and $G=\mathrm{e}^{\theta F}$, $\theta\geq 0$, gives, using the fact that $Y_\alpha$ has the law of $X$ for all $\alpha$, \begin{align*} \mathbb{E}F(X)\mathrm{e}^{\theta F(X)} &=\theta\int_0^1\mathbb{E}\langle\nabla F(X_\alpha),\nabla F(Y_\alpha)\rangle\mathrm{e}^{\theta F(Y_\alpha)}\mathrm{d}\alpha\\ &\leq\theta\int_0^1\mathbb{E}|\nabla F(X_\alpha)||\nabla F(Y_\alpha)|\mathrm{e}^{\theta F(Y_\alpha)}\mathrm{d}\alpha\\ &\leq\theta\int_0^1\mathbb{E}\mathrm{e}^{\theta F(Y_\alpha)}\mathrm{d}\alpha\\ &=\theta\mathbb{E}\mathrm{e}^{\theta F(X)}. \end{align*} Introducing the Laplace transform $L(\theta):=\mathbb{E}\mathrm{e}^{\theta F(X)}$, this is the differential inequality \[ L'(\theta)\leq\theta L(\theta), \quad\text{hence}\quad \log\mathbb{E}\mathrm{e}^{\theta F} \leq\frac{\theta^2}{2}. \] By exponential Markov inequality, for all $r\geq0$, \[ \mathbb{P}(F(X)\geq r) \leq\inf_{\theta > 0}\mathrm{e}^{-\theta r}\mathbb{E}\mathrm{e}^{\theta F} \leq\exp\Bigr(-\frac{r^2}{2}\Bigr). \] Finally, by translation, dilation, and approximation with the Rademacher theorem on Lipschitz functions, if $X\sim\mathcal{N}(m,\Sigma)$ then for all $F:\mathbb{R}^n\to\mathbb{R}$ and all $r\geq0$, \begin{align*} \mathbb{P}(|F(X)-\mathbb{E}F(X)|\geq r) &\leq2\exp\Bigr(-\frac{r^2}{2\|F(\sqrt{\Sigma}\cdot)\|_{\mathrm{Lip.}}^2}\Bigr)\\ &\leq2\exp\Bigr(-\frac{r^2}{2\|\Sigma\|_{\mathrm{op.}}\|\nabla F\|_{\mathrm{Lip.}}^2}\Bigr). \end{align*} In the sequel, for simplicity, we formulate the results for $\mathcal{N}(0,I_n)$ only.
Finer tail from Pisier inequality. Recall the Mills bound for $Z\sim\mathcal{N}(0,1)$ and $r > 0$: \[ \mathbb{P}(Z\geq r) =\int_r^\infty\frac{\mathrm{e}^{-\frac{x^2}{2}}}{\sqrt{2\pi}}\mathrm{d}x \leq\int_r^\infty\frac{x}{r}\frac{\mathrm{e}^{-\frac{x^2}{2}}}{\sqrt{2\pi}}\mathrm{d}x \leq\frac{1}{\sqrt{2\pi}r}\int_r^\infty(-\mathrm{e}^{-\frac{x^2}{2}})'\mathrm{d}x =\frac{\mathrm{e}^{-\frac{r^2}{2}}}{\sqrt{2\pi}r}, \] a quantitative estimate that catches the erfc asymptotics $\mathbb{P}(Z\geq r)\sim\frac{\mathrm{e}^{-\frac{r^2}{2}}}{\sqrt{2\pi}r}$ as $r\to\infty$.
Following [BGH, H], if say $X\sim\mathcal{N}(0,I_n)$, then for all $F:\mathbb{R}^n\to\mathbb{R}$, $\|F\|_{\mathrm{Lip.}}\leq1$, $r > 0$, \[ \mathbb{P}(|F(X)-\mathbb{E}F(X)|\geq r) \leq\sqrt{\frac{\pi}{2}}\frac{\mathrm{e}^{-\frac{r^2}{2}}}{r}, \] generalizing the Mills bound with a worse constant.
Let us give a proof following [BGH]. Set $Y=F(X)-\mathbb{E}F(X)$. By smoothing, we can assume that $F$ is smooth and $Y$ has positive density $p$. The covariance representation above gives, for all bounded and piecewise differentiable $U:\mathbb{R}\to\mathbb{R}$, that \[ \mathbb{E}YU(Y)\leq\mathbb{E}U'(Y). \] Specializing to the piecewise step function $U(x)=\min(x-r)_+,\varepsilon)$, we get, \[ \mathbb{E}Y(Y-r)\mathbb{1}_{r\leq Y\leq r+\varepsilon} +\varepsilon\mathbb{E}Y\mathbb{1}_{Y\geq r+\varepsilon} \leq\mathbb{P}(Y\geq r+\varepsilon)-\mathbb{P}(Y\geq r). \] Dividing by $\varepsilon$ and sending $\varepsilon$ to $0$ gives, for all $r > 0$, \[ m(r):=\mathbb{E}Y\mathbb{1}_{Y\geq r}\leq p(r). \] Since $m(r)=\int_r^\infty xp(x)\mathrm{d}x$ and $m'(r)=-rp(r)$, we have obtained the differential inequality $m(r)\leq -m'(r)/r$. Therefore $r\mapsto\log m(r)+\frac{1}{2}r^2$ is non-increasing. It follows that $r\geq0\mapsto\mathrm{e}^{\frac{r^2}{2}}\mathbb{E}Y\mathbb{1}_{Y\geq r}=m(r)\mathrm{e}^{\frac{r^2}{2}}$ is non-increasing. Hence, for all $r > 0$, \[ \mathbb{P}(Y\geq r) \leq\mathbb{E}Y\mathbb{1}_{Y\geq0}\frac{\mathrm{e}^{-\frac{r^2}{2}}}{r}. \] It remains to use the Pisier concentration inequality to get $\mathbb{E}|Y|\leq\sqrt{\pi/2}\||\nabla F|\|_\infty$.
Pisier concentration inequality with Maurey version of the proof. Following [P, Theorem 2.2], if say $X\sim\mathcal{N}(0,I_n)$ then for all $\Phi:\mathbb{R}\to\mathbb{R}$ convex and $F:\mathbb{R}^n\to\mathbb{R}$, \[ \mathbb{E}\Phi(F(X)-\mathbb{E}F(X)) \leq \mathbb{E}\Phi(F(X)-F(X')) \leq\mathbb{E}\Phi(\tfrac{\pi}{2}\langle\nabla F(X),X'\rangle) \] where $X'$ is an independent copy of $X$. Examples include $\Phi(x)=\mathrm{e}^{\theta x}$ and $\Phi(x)=|x|$.
The first inequality comes from the Jensen inequality with respect to the expectation on $X'$. To prove the second inequality, let us follow Pisier and Maurey. Set $X_\alpha:=\alpha X+\sqrt{1-\alpha^2}X'$ and $X'_\alpha:=\sqrt{1-\alpha^2}X-\alpha X'$, $\alpha\in[0,1]$. Then $\partial_\alpha X_\alpha=\frac{1}{\sqrt{1-\alpha^2}}X'_\alpha$, and \[ F(X)-F(X')=F(X_1)-F(X_0) =\int_0^1\langle\nabla F(X_\alpha),X'_\alpha\rangle\frac{\mathrm{d}\alpha}{\sqrt{1-\alpha^2}}. \] The Jensen inequality for $\Phi$ and the arcsine law on $[0,1]$ of density $f(\alpha)=\frac{2}{\pi\sqrt{1-\alpha^2}}$ gives \[ \Phi(F(X)-F(X')) \leq\int_0^1\Phi\bigr(\tfrac{\pi}{2}\langle\nabla F(X_\alpha),X'_\alpha\rangle\bigr)f(\alpha)\mathrm{d}\alpha. \] By the Fubini-Tonelli theorem \[ \mathbb{E}\Phi(F(X)-F(X')) \leq\int_0^1\mathbb{E}\Phi\bigr(\tfrac{\pi}{2}\langle\nabla F(X_\alpha),X'_\alpha\rangle\bigr)f(\alpha)\mathrm{d}\alpha. \] Now $(X_\alpha,X'_\alpha)$ is the image of the standard Gaussian vector $(X',X)=(X_0,X'_0)$ by a rotation (of angle $\arccos\alpha$) and since the standard Gaussian law is rotationally invariant, the law of $(X_\alpha,X'_\alpha)$ does not depend on $\alpha$. Hence the expectation under the integral is a constant function of $\alpha$, and this gives finally the desired Pisier concentration inequality.
In the case $\Phi(x)=|x|$, we get, using Fubini-Tonelli or conditionning over $X$, \[ \mathbb{E}|F(X)-\mathbb{E}F(X)| \leq\frac{\pi}{2}\mathbb{E}|\langle\nabla F(X),X'\rangle| =\frac{\pi}{2}\mathbb{E}_X\mathbb{E}_{X'}|\langle\nabla F(X),X'\rangle|. \] Now, using the Cauchy-Schwarz inequality to upper bound the right hand side would be too rough. Instead, we note that at fixed $X$, by rotational invariance, $\langle\nabla F(X),X'\rangle$ has the law of $|\nabla F(X)|\langle e_1,X'\rangle$, namely the law of $|\nabla F(X)|Z$ with $Z\sim\mathcal{N}(0,1)$. Therefore, we get \[ \mathbb{E}|F(X)-\mathbb{E}F(X)| \leq\frac{\pi}{2}\||\nabla F(X)|\|_\infty\mathbb{E}(|Z|) =\sqrt{\frac{\pi}{2}}\||\nabla F(X)|\|_\infty. \]
Note that only the rotational invariance of the Gaussian is used in these Pisier-Maurey proofs. In particular, the results remain available for rotational invariant distributions such as multivariate Barenblatt/Student/Cauchy distributions, see for instance [BV].
It is natural to ask about the optimality of the constant $\pi/2$ in the Pisier concentration inequality. In the affine case $F(x)=\langle a,x\rangle+b$, $|a|=1$, we have $F(X)-F(X')\sim\mathcal{N}(0,2)$ and $\langle\nabla F(X),X'\rangle\sim\mathcal{N}(0,1)$, hence the optimal constant for affine $F$ is $\sqrt{2} < \pi/2$.
Finer tail via stochastic calculus, following Ibragimov, Tsirelson, and Sudakov. Maurey is known in probabilistic functional analysis for proofs using stochastic calculus. He was not the first. As a matter of fact, let us examine an argument giving finer concentration due to Ibragimov, Sudakov, and Tsirelson in [IST, Theorem 1 and Corollaries 1 and 2 p. 25-27] : if $X\sim\mathcal{N}(0,I_n)$ and $F:\mathbb{R}^n\to\mathbb{R}$, $\||\nabla F|\|_{\infty}\leq1$, then the random variable $F(X)-\mathbb{E}F(X)$ has the law of $B_T$ where $B$ is a standard real Brownian motion and $T$ a stopping time such that $T\leq 1$.
As a consequence, for all $r\geq0$, by the reflection principle, \[ \mathbb{P}(F(X)-\mathbb{E}F(X)\geq r) = \mathbb{P}(B_T\geq r) \leq \mathbb{P}\Bigr(\sup_{0\leq t\leq 1}B_t\geq r\Bigr) = \mathbb{P}(|B_1|\geq r)=2Q(r) \] where $Q$ is the tail of the standard Gaussian $\mathcal{N}(0,1)$. It follows that for all $r\geq0$, \[ \mathbb{P}(|F(X)-\mathbb{E}F(X)|\geq r) \leq4Q(r). \] Recall the Mills bound and the erfc asymptotics \[ Q(r)=\int_r^\infty\frac{\mathrm{e}^{-\frac{x^2}{2}}}{\sqrt{2\pi}}\mathrm{d}x \leq\frac{\mathrm{e}^{-\frac{r^2}{2}}}{r\sqrt{2\pi}} \quad\text{and}\quad Q(r)=\frac{1}{2}\mathrm{erfc}\Bigr(\frac{r}{\sqrt{2}}\Bigr)\sim_{r\to+\infty}\frac{\mathrm{e}^{-\frac{r^2}{2}}}{r\sqrt{2\pi}}\Bigr(1-\frac{1}{r^2}+\frac{3}{r^4}-\cdots\Bigr). \] Back to the construction of $T$ such that $F(X)-\mathbb{E}F(X)$ and $B_T$ are identical in law, it is not a surprise to get something of this kind, having in mind Skorokhod representation theorems, what is remarquable is to get it with $T\leq1$. Let ${(W_s)}_{s\in[0,1]}$ be an $n$-dimensional standard Brownian motion, and let us consider the martingale \[ M_s=\mathbb{E}(F(W_1)\mid\mathcal{F}_s), \quad s\in[0,1]. \] Then $M_0=\mathbb{E}F(X)$, while $M_1$ has the law of $F(X)$. By the Dubins-Schwarz theorem, there exists a real Brownian motion $B$ such that ${(B_{\langle M\rangle_s})}_{0\leq s\leq 1}$ and ${(M_s-M_0)}_{0\leq s\leq 1}$ have same law, in particular $M_1-M_0=F(X)-\mathbb{E}F(X)$ has the law of $B_T$ with $T:=\langle M\rangle_1$.
It remains to show that $\langle M\rangle_1\leq1$. Let ${(P_s)}_{s\in[0,1]}$ be the heat or Markov semigroup of $W$ defined by $P_s(f)(x)=\mathbb{E}(f(W_s)\mid W_0=x)$. Then, by the Markov property, we get $M_s=\mathbb{E}(F(W_1)\mid W_s)=P_{1-s}(F)(W_s)$. Next, the Itô formula gives \[ M_t=M_0+\int_0^t\nabla P_{1-s}(F)(W_s)\cdot\mathrm{d}W_s, \quad\text{hence}\quad \langle M\rangle_t=\int_0^t|\nabla P_{1-s}(F)|^2(W_s)\mathrm{d}s. \] Now $\nabla P_{1-s}(F)=P_{1-s}\nabla F$, thus $|\nabla P_{1-s}(F)|\leq P_{1-s}|\nabla F|$, hence $\||\nabla P_{1-s}(F)|\|_\infty\leq1$.
Note that $\mathbb{E}(|F(X)-\mathbb{E}F(X)|^2)=\mathbb{E}\langle M\rangle_1\leq1$, hence $\mathbb{E}|F(X)-\mathbb{E}F(X)|\leq1$, a better bound than the $\sqrt{\pi/2}$ obtained from Pisier inequality above. Moreover the affine case $F(x)=\langle a,x\rangle+b$, $|a|=1$, shows that this bound is in fact optimal.
Further comments. The concentration of Lipschitz functions for the uniform distribution on $\mathbb{S}^{n-1}$ dates back at least to Milman and Schechtman, as a consequence of the Lévy isoperimetric inequality for the uniform distribution on the sphere. In high-dimension, as $n\to\infty$, its gives the concentration of Lipschitz functions for the standard normal distribution, which can also be deduced from the Gaussian isoperimetric inequality of Sudakov and Tsirelson, or from the logarithmic Sobolev inequality of Gross via the Herbst argument, or from the Talagrand transportation inequality, or from the infimum convolution inequality of Maurey. As we have explained, finer versions can be deduced, following Ibragimov, Sudakov, and Tsirelson [IST], by using stochastic calculus, or by using the concentration inequality of Pisier [P]. Following Bobkov, Götze, and Houdré [BGH, H], it can also be deduced quickly from a covariance representation put forward by Houdré and Pérez-Abreu [HPA], which can be seen as the Ornstein-Uhlenbeck case of the Hörmander-Helffer-Sjöstrand covariance representation formula. A spherical version is considered by Bobkov and Duggal in [BD]. Finer tail bounds are also considered by Aubrun, Jenkinson, and Szarek in [AJS].
The concentration of measure phenomenon is related to the notion of observable diameter developed by Mikhaïl Gromov in metric geometry, see for instance [G,S].
The idea of writing this post came after a question asked by Anthony Nguyen, a PhD student in signal processing at INRIA and École normale supérieure Paris-Saclay.
Further reading.
- [Lé] Paul Lévy
Problèmes concrets d'analyse fonctionnelle
Gauthier-Villars, 1951 - [MS] Vitali Davidovich Milman, Gideon Schechtman, with an appendix by Mikhaïl Gromov
Asymptotic theory of finite dimensional normed spaces
Springer, 1986 - [G] Mikhaïl Gromov
Metric structures for Riemannian and non-Riemannian spaces
With appendices by Mikhaïl Katz, Pierre Pansu and Stephen Semmes
Translated from the 1981 French original by Sean Michael Bates
Reprint of the 2001 English edition. Birkhäuser 2007. xx+585pp
Including the famous chapter $3\frac{1}{2}$ on concentration of measure - [B] Christer Borell
The Brunn-minkowski inequality in Gauss space
Inventiones mathematicae, 1975 - [S] Takashi Shioya
Metric Measure Geometry: Gromov's Theory of Convergence and Concentration of Metrics and Measures
IRMA Lectures in Mathematics & Theoretical Physics, EMS 2016 - [IST] Ildar Abdulovich Ibragimov, Valdimir Nikolaevich Sudakov, and Boris Semyonovich Tsirelson
Norms of Gaussian sample functions
Proceedings of the Third Japan-USSR Symposiumon Probability Theory
Lecture Notes in Math. 550, pp. 20-41. Springer (1976) - [ST] Vladimir Nikolaevich Sudakov, Boris Semyonovich Tsirelson
Extremal properties of half-spaces for spherically invariant measures
Journal of Soviet Mathematics, 1978 - [P] Gilles Pisier
Probabilistic methods in the geometry of Banach spaces
Probability and Analysis, Lecture Notes in Math. 1206, pp. 167-241. Springer (1986) - [M] Bernard Maurey
Some deviations inequalities
Geometric & Functional Analysis (GAFA), 1(2), 188-197 (1991) - [T1] Michel Talagrand
A new isoperimetric theorem and its application to concentration of measure phenomena
Geometric & Functional Analysis (GAFA), 1(2), 211–223 (1991) - [T2] Michel Talagrand
Transportation cost for Gaussian and other product measures
Geometric & Functional Analysis (GAFA), 6(3), 587–600 (1995) - [HPA] Christian Houdré and Victor Pérez-Abreu
Covariance identities and inequalities for functionals on Wiener and Poisson spaces
Ann. Probab., 23, 400-419 (1995) - [BG] Sergey Germanovich Bobkov, Frederich Götze
Exponential integrability and transportation cost related to logarithmic Sobolev inequalities
Journal of Functional Analysis, 163(1), 1–28 (1999) - [BGH] Sergey G. Bobkov, Frederich Götze, and Christian Houdré
On Gaussian and Bernoulli covariance representations
Bernoulli 7 (2001), 439-451 - [Le] Michel Ledoux
The concentration of measure phenomenon
AMS, 2001 - [BV] Serget G. Bobkov and Bruno Volzone
On Gilles Pisier's approach to Gaussian concentration, isoperimetry, and Poincaré-type inequalities
http://arxiv.org/abs/2311.03506 - [H] Christian Houdré
Covariance representation and an elementary proof of the Gaussian concentration inequality
https://arXiv.org/abs/2410.06937 - [AJS] Guillaume Aubrun, Justin Jenkinson, Stanislaw J. Szarek
Optimal constants in concentration inequalities on the sphere and in the Gauss space
https://arXiv.org/abs/2406.13581 - [BD] Sergey G. Bobkov and Devraj Duggal
Spherical covariance representations
https://arXiv.org/abs/2403.19089
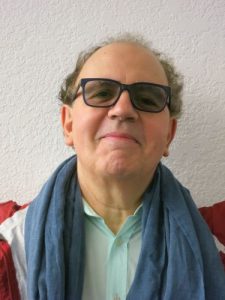