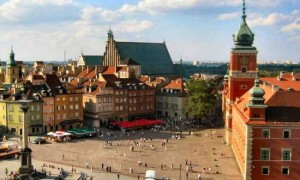
Recently I had the great pleasure to upload on arXiv a joint work arXiv:1303.5838 with Radek Adamczak in which we explore the validity of the circular law for random matrices with non i.i.d. entries. Recall that among the basic results of random matrix theory, the circular law is the simplest to state and also the hardest to prove. It states that the empirical distribution of the eigenvalues of an \( {n\times n} \) random matrix with i.i.d. entries of variance \( {1} \), when scaled by \( {\sqrt{n}} \), tends as \( {n\rightarrow\infty} \) to the uniform distribution on the unit disc of the complex plane.
Our main result is as follows. Let \( {A} \) be a random \( {n\times n} \) real matrix having as a random vector in \( {\mathbb{R}^{n\times n}} \) a log-concave isotropic unconditional law. Then with probability one, as \( {n\rightarrow\infty} \), the empirical spectral distribution of \( {\frac{1}{\sqrt{n}}A} \) tends to the uniform distribution on the unit disc.
About the assumptions. The entries of \( {A} \) are uncorrelated and have a symmetric law of zero mean and unit variance. This allows for some dependence and non equidistribution among the entries, while keeping the special case of i.i.d. standard Gaussian (or exponential) entries. If one drops the unconditionality assumption then the limiting spectral measure needs not be the circular law (there are examples in a paper by Feinberg and Zee (eq. (5.8) p. 654) and Guionnet-Krishnapur-Zeitouni. This is in contrast with the model of random matrices with i.i.d. log-concave rows (see Adamczak) for which it turned out that the circular law holds without assuming unconditionality.
Method of proof. We prove our main result using the Tao and Vu version of the Hermitization method of Girko, presented in a recent survey on the circular law. This boils down the problem to define the Hermitian matrix
\[ B_n(z):=\sqrt{\left(\frac{1}{\sqrt{n}}A_n-zI_n\right)\left(\frac{1}{\sqrt{n}}A_n-zI_n\right)^\ast} \]
for every \( {z\in\mathbb{C}} \), and to check the following couple of properties:
- (i) For all \( {z \in \mathbb{C}} \) the spectral measure \( {\nu_{z,n}} \) of \( {B_n(z)} \) converges almost surely to some deterministic probability measure \( {\nu_z} \) on \( {\mathbb{R}_+} \) which depends only on \( {z} \) (universality). Moreover, for almost all \( {z\in \mathbb{C}} \),
\[ U(z) := -\int_{\mathbb{R}_+} \log (s) \nu_z(ds) = -(\log|z|)\mathbf{1}_{|z|>1}+\frac{1-|z|^2}{2}\mathbf{1}_{|z|\leq1}. \]
- (ii) For all \( {z \in \mathbb{C}} \), with probability one the function \( {s \mapsto \log(s)} \) is uniformly integrable with respect to the family of measures \( {\{\nu_{z,n}\}_{n\ge 1}} \).
The link between \( {A_n} \) and \( {B_n(z)} \) is given by the Girko Hermitization identity:
\[ U_n(z) :=-\log|\det(A_n)| =-\log\det(B_n(z)) =-\int_0^\infty\!\log(s)\,\nu_{z,n}(ds). \]
The quantity \( {U_n(z)} \) is the logarithmic potential at point \( {z} \) of the empirical spectral distribution formed with the eigenvalues of \( {A_n} \). To prove (i), we use concentration of measure for the Cauchy-Stieltjes transform of \( {B_n(z)} \) to get the convergence to a universal limit, and the well known Gaussian case with i.i.d. entries to get the formula. To prove (ii), we need to control the largest and smallest and a bunch of small eigenvalues of \( {B_n(z)} \). This is done by using remarkable properties of unconditional log-concave distributions regarding tails and densities.
About log-concavity. We say that a probability measure \( {\mu} \) on \( {\mathbb{R}^d} \) is log-concave when for all nonempty compact sets \( {A,B} \) and \( {\theta \in (0,1)} \),
\[ \mu(\theta A + (1-\theta)B) \ge \mu(A)^\theta\mu(B)^{1-\theta}. \]
A measure \( {\mu} \) not supported on a proper affine subspace of \( {\mathbb{R}^d} \) is log-concave iff it has density \( {e^{-V}} \) where \( {V \colon \mathbb{R}^d \rightarrow \mathbb{R}\cup\{+\infty\}} \) is a convex function, see for instance the lecture notes by Giannopoulos. We say that \( {\mu} \) is isotropic if it is centered and its covariance matrix is the identity, in other words if the coordinates are uncorrelated, centered, and have unit variance. Recall finally that \( {\mu} \) is called unconditional if \( {X=(X_1,\ldots,X_d)} \) and \( {(\varepsilon_1 X_1,\ldots,\varepsilon_d X_d)} \) have the same law when \( {X} \) has law \( {\mu} \) and \( {\varepsilon_1,\ldots,\varepsilon_d} \) are i.i.d. Rademacher random variables (signs) independent of \( {X} \). This means that the law of \( {X} \) has the symmetries of the cube. The isotropy and the unconditionality are related to the canonical basis of \( {\mathbb{R}^d} \), while the log-concavity is not. Note that unconditionality together with unit variances imply automatically isotropy.
Log-concave distributions play an essential role in high dimensional and asymptotic geometric analysis. The uniform probability distribution on a convex and compact set (such as \( {\ell_p(\mathbb{R}^d)} \) balls) is always log-concave. Beyond convex bodies, the most basic examples of log-concave measures are products of Gaussian measures \( {\exp(-\|x\|_2^2-\frac{d}{2}\log(\pi))} \) and products of symmetric exponential measures \( {\exp(-\|x\|_1-d\log(2))} \). The dogma is that most high dimensional properties of isotropic log-concave measures are similar to the ones of these two basic examples. A good way to understand this phenomenon is to take a look at the central limit theorem for convex bodies say for simplicity when the body is an \( {\ell_p} \) ball.
The log-concave property is stable by many common transformations such as by tensor product, by convolution product, by taking marginals, projections, affine images, conditional distributions, etc. The geometric and probabilistic analysis of log-concave measures is a well developed area of research. What we will need for our analysis is properties related to the behavior of densities and concentration of measure results. Many of such questions are difficult and related to famous open problems in asymptotic geometric analysis. Fortunately, unconditional log-concave measures behave in a much more rigid way than general ones, in particular some of the conjectures have been answered either completely or up to terms which are logarithmic in the dimension and as such do not cause difficulties in the problems we are about to deal with. Below we present the ingredients we will use throughout the proof.
- Sub-exponential tails. If \( {X} \) is mean zero and variance one log-concave real random variable then for all \( {t \ge 0} \),
\[ \mathbb{P}(|X| \ge t) \le 2\exp(-ct). \]
A proof can be found in an old paper by Christer Borell (not Émile Borel);
- Comparison of tails. If \( {X} \) is an isotropic unconditional log-concave random vector in \( {\mathbb{R}^n} \) and if \( {\mathcal{E}} \) is a standard \( {n} \)-dimensional symmetric exponential vector (i.e. with i.i.d. components following a symmetric exponential distribution with variance one), then for any semi-norm \( {\|\cdot\|} \) on \( {\mathbb{R}^n} \) and any \( {t > 0} \),
\[ \mathbb{P}(\|X\| \ge Ct) \le C\mathbb{P}(\|\mathcal{E}\| \ge t) \]
where \( {C} \) is a universal constant. A proof can be found in a paper by Rafal Latala. We use it in order to control the largest eigenvalue of \( {B_n(z)} \);
- Density bound. If \( {X} \) is an isotropic unconditional log-concave random vector in \( {\mathbb{R}^n} \) then its density is bounded from above by \( {C^n} \), where \( {C} \) is a universal constant. A proof can be found in Bobkov and Nazarov. We use this property to control small ball probabilities. The famous slicing conjecture states that such a bound is valid even without unconditionality.
- Poincaré inequality. If \( {X} \) is an isotropic unconditional log-concave random vector in \( {\mathbb{R}^n} \), then for every smooth \( {f\colon \mathbb{R}^n \rightarrow \mathbb{R}} \),
\[ \mathrm{Var}(f(X)) \le C\log^2(n+1)\mathbb{E}(|\nabla f(X)|^2) \]
where \( {C} \) is a universal constant. A proof can be found in a paper by Klartag. The famous Kannan-Lovasz-Simonovits (KLS) conjecture states that this holds even without unconditionality and without the logarithm! We use the Poincaré inequality above in order to get concentration of measure for Lipschitz functions, via upper bounds on the Laplace transform (Herbst argument). Namely, this gives that for any 1-Lipschitz function \( {g\colon \mathbb{R}^n \rightarrow \mathbb{R}} \) and all \( {t > 0} \),
\[ \mathbb{P}(|g(X)-\mathbb{E}(g(X))| \ge t) \le 2\exp(-ct/\log(n+1)) \]
where \( {c} \) is a constant. We will use it with the random version \( {X=A} \) in \( {\mathbb{R}^{n^2}} \), and with \( {g(X):=m_A(\xi)} \), the Cauchy-Stieltjes transform of the empirical spectral distribution of \( {\sqrt{AA^*}} \) at point \( {\xi\in\mathbb{C}_+} \).
About unconditionality again, in order to control a bunch of small eigenvalues of \( {B_n(z)} \), we need a bound for the distance of a row of \( {A_n} \) to the span of a bunch of rows of \( {A_n} \). Here unconditionality enters the scene via a very well known trick: it allows to introduce artificially Rademacher random variables (i.e. random signs) and then to use for instance the Khintchine-Kahane inequality and Talagrand concentration inequality on the discrete cube.
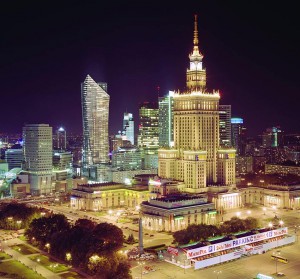