Star-fish. My next door colleague Olivier Guédon is an expert in high dimensional convex geometry. He likes to picture convex bodies as some sort of starfishs. This habit is motivated by the fact that in high dimension, the extremal points of a convex body can be really far away from the center, further than most of the other points of the boundary. For instance, one may think about the cube \( {\frac{1}{2}[-1,1]^n} \) in \( {\mathbb{R}^n} \) of unit volume, for which the extremal points \( {\frac{1}{2}(\pm 1,\ldots,\pm 1)} \) have length \( {\frac{1}{2}\sqrt{n}} \), which becomes huge in high dimension, while the cube edge length remains equal to \( {1} \). This leads to the natural question of the location of most of the mass of the convex body: is it close to the center or close to the extremal points? An answer is provided by the central limit theorem (probabilistic asymptotic geometric analysis!).
Thin-shell. A random vector \( {X} \) of \( {\mathbb{R}^n} \) follows the uniform law on \( {\frac{1}{2}[-1,1]^n} \) iff its coordinates \( {X_1,\ldots,X_n} \) are i.i.d. and follow the uniform law on \( {\frac{1}{2}[-1,1]} \), for which
\[ m_2:=\mathbb{E}(X_i^2)=\frac{1}{12}, \quad m_4:=\mathbb{E}(X_i^4)=\frac{1}{80}. \]
In particular, \( {X_1^2,\ldots,X_n^2} \) have mean \( {m_2} \) and variance \( {\sigma^2=m_4-m_2^2=\frac{1}{180}} \). For every \( {r>0} \), let us consider the thin shell (or ring)
\[ \mathcal{R}_n(r) :=\left\{x\in\mathbb{R}^n:-r\leq\frac{\left\Vert x\right\Vert_2^2-nm_2}{\sqrt{n\sigma^2}}\leq r\right\}. \]
Then, thanks to the central limit theorem, we have
\[ \mathrm{Vol}\left(\frac{1}{2}[-1,1]^n\cap\mathcal{R}_n(r)\right) =\mathbb{P}((X_1,\ldots,X_n)\in\mathcal{R}_n(r)) \underset{n\rightarrow\infty}{\longrightarrow} \int_{-r}^r\!\frac{e^{-\frac{1}{2}t^2}}{\sqrt{2\pi}}\,dt. \]
Taking \( {r} \) large enough such that the right hand side is close to \( {1} \) (every statistician knows that we get \( {0.95} \) for \( {r=1.96} \)), this indicates that when \( {n\gg1} \) most of the volume of the cube is contained in a thin shell at radial position \( {\sqrt{nm_2}} \). It turns out that this high dimensional phenomenon is not specific to the cube (\( {\ell^\infty} \) ball) and remains valid for any convex body (exercise: consider the \( {\ell^2} \) ball and the \( {\ell^1} \) ball). This is also at the heart of the central limit theorem for convex bodies (references on the subject are given in this previous post).
Law of Large Numbers. In some sense log-concave distributions are geometric generalizations of product distributions with sub-exponential tails. A quick way to have in mind the thin-shell phenomenon is to think about a random vector \( {X} \) with i.i.d. coordinates. The Law of Large Numbers states that almost surely the norm \( {\left\Vert X\right\Vert=(X_1^2+\cdots+X_n^2)^{1/2}} \) is close to \( {\sqrt{nm}} \) where \( {m:=\mathbb{E}(X_1^2)} \) when \( {n\gg1} \). Also most of the mass of \( {X} \) is in a thin-shell at radius \( {nm} \), a nice high dimensional phenomenon.
Further reading. You may read the survey Concentration phenomena in high dimensional geometry, by Olivier Guédon (2014).
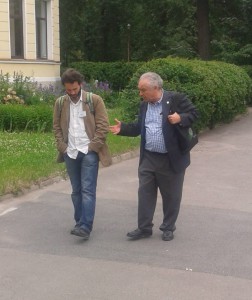