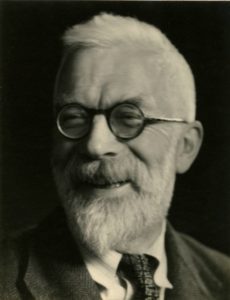
Fisher information. The Fisher information or divergence of a positive Borel measure measure \( {\nu} \) with respect to another one \( {\mu} \) on the same space is
\[ \mathrm{Fisher}(\nu\mid\mu) =\int\left|\nabla\log\textstyle\frac{\mathrm{d}\nu}{\mathrm{d}\mu}\right|^2\mathrm{d}\nu =\int\frac{|\nabla\frac{\mathrm{d}\nu}{\mathrm{d}\mu}|^2}{\frac{\mathrm{d}\nu}{\mathrm{d}\mu}}\mathrm{d}\mu =4\int\left|\nabla\sqrt{\textstyle\frac{\mathrm{d}\nu}{\mathrm{d}\mu}}\right|^2\mathrm{d}\mu %=4\int\left|\nabla\sqrt{\varphi}\right|^2\mathrm{d}\mu %=\int_{\{\varphi>0\}}\frac{\left|\nabla \varphi\right|^2}{\varphi}\mathrm{d}\mu \in[0,+\infty] \]
if \( {\nu} \) is absolutey continuous with respect to \( {\mu} \), and \( {\mathrm{Fisher}(\nu\mid\mu)=+\infty} \) otherwise.
It plays a role in the analysis and geometry of statistics, information, partial differential equations, and Markov diffusion stochastic processes. It is named after Ronald Aylmer Fisher (1890 – 1962), a British scientist who is also the Fisher of many other objects and concepts including for instance:
- the Fisher information of a statistical model and the Fisher information metric,
- the Fisher exact statistical test,
- the Fisher F probability distribution,
- the Fisher–Tippett extreme value probability distribution,
- the Fisher–Kolmogorov–Petrovsky–Piskunov (FKPP) partial differential equation,
- the Wright-Fisher model for genetic drift,
- the Fisher principle in evolutionary biology.
However, he should not be confused with for instance:
- Irving Fisher (1867 — 1947) and the Fisher equation in mathematical finance,
- Michael Fisher (1931 — ) who has contributions to equilibrium statistical mechanics,
- Ernst Sigismund Fischer (1875 — 1954) related to the Courant–Fischer–Weyl minimax variational formulas for eigenvalues and to the Riesz-Fischer theorem
Let us denote \( {|x|=\sqrt{x_1^2+\cdots+x_n^2}} \) and \( {x\cdot y=x_1y_1\cdots+x_ny_n} \) for all \( {x,y\in\mathbb{R}^n} \).
Explicit formula for Gaussians. For all \( {n\geq1} \), all vectors \( {m_1,m_2\in\mathbb{R}^n} \), and all \( {n\times n} \) covariance matrices \( {\Sigma_1} \) and \( {\Sigma_2} \), we have
\[ \mathrm{Fisher}(\mathcal{N}(m_1,\Sigma_1)\mid\mathcal{N}(m_2,\Sigma_2)) =|\Sigma_2^{-1}(m_1-m_2)|^2+\mathrm{Tr}(\Sigma_2^{-2}\Sigma_1-2\Sigma_2^{-1}+\Sigma_1^{-1}). \]
When \( {\Sigma_1} \) and \( {\Sigma_2} \) commute, this reduces to the following, closer to the univariate case,
\[ \mathrm{Fisher}(\mathcal{N}(m_1,\Sigma_1)\mid\mathcal{N}(m_2,\Sigma_2)) =|\Sigma_2^{-1}(m_1-m_2)|^2+\mathrm{Tr}(\Sigma_2^{-2}(\Sigma_2-\Sigma_1)^2\Sigma_1^{-1}). \]
In the univariate case, this reads, for all \( {m_1,m_2\in\mathbb{R}} \) and \( {\sigma_1^2,\sigma_2^2\in(0,\infty)} \),
\[ \mathrm{Fisher}(\mathcal{N}(m_1,\sigma_1^2)\mid\mathcal{N}(m_2,\sigma_2^2)) =\frac{(m_1-m_2)^2}{\sigma_2^4}+\frac{(\sigma_2^2-\sigma_1^2)^2}{\sigma_1^2\sigma_2^4}. \]
A proof. If \( {X\sim\mathcal{N}(m,\Sigma)} \) then, for all \( {1\leq i,j\leq n} \),
\[ \mathbb{E}(X_iX_j)=\Sigma_{ij}+m_im_j, \]
hence, for all \( {n\times n} \) symmetric matrices \( {A} \) and \( {B} \),
\[ \begin{array}{rcl} \mathbb{E}(AX\cdot BX) &=&\mathbb{E}\sum_{i,j,k=1}^nA_{ij}X_jB_{ik}X_k\\ &=&\sum_{i,j,k=1}^nA_{ij}B_{ik}\mathbb{E}(X_jX_k)\\ &=&\sum_{i,j,k=1}^nA_{ij}B_{ik}(\Sigma_{jk}+m_jm_k)\\ &=&\mathrm{Trace}(A\Sigma B)+Am\cdot Bm, \end{array} \]
and thus for all \( {n} \)-dimensional vectors \( {a} \) and \( {b} \),
\[ \begin{array}{rcl} \mathbb{E}(A(X-a)\cdot B(X-b)) &=&\mathbb{E}(AX\cdot BX)+A(m-a)\cdot B(m-b)-Am\cdot Bm\\ &=&\mathrm{Trace}(A\Sigma B)+A(m-a)\cdot B(m-b). \end{array} \]
Now, using the notation \( {q_i(x)=\Sigma_i^{-1}(x-m_i)\cdot(x-m_i)} \) and \( {|\Sigma_i|=\det(\Sigma_i)} \),
\[ \begin{array}{rcl} \mathrm{Fisher}(\Gamma_1\mid\Gamma_2) &=&\displaystyle4\frac{\sqrt{|\Sigma_2|}}{\sqrt{|\Sigma_1|}}\int\Bigr|\nabla\mathrm{e}^{-\frac{q_1(x)}{4}+\frac{q_2(x)}{4}}\Bigr|^2\frac{\mathrm{e}^{-\frac{q_2(x)}{2}}}{\sqrt{2\pi|\Sigma_2|}}\mathrm{d}x\\ &=&\displaystyle\int|\Sigma_2^{-1}(x-m_2)-\Sigma_1^{-1}(x-m_1)|^2\frac{\mathrm{e}^{-\frac{q_1(x)}{2}}}{\sqrt{2\pi|\Sigma_1|}}\mathrm{d}x\\ &=&\displaystyle\int(|\Sigma_2^{-1}(x-m_2)|^2\\ &&\qquad-2\Sigma_2^{-1}(x-m_2)\cdot\Sigma_1^{-1}(x-m_1)\\ &&\qquad+|\Sigma_1^{-1}(x-m_1)|^2)\frac{\mathrm{e}^{-\frac{q_1(x)}{2}}}{\sqrt{2\pi|\Sigma_1|}}\mathrm{d}x\\ &=&\mathrm{Trace}(\Sigma_2^{-1}\Sigma_1\Sigma_2^{-1})+|\Sigma_2^{-1}(m_1-m_2)|^2-2\mathrm{Trace}(\Sigma_2^{-1})+\mathrm{Trace}(\Sigma_1^{-1})\\ &=&\mathrm{Trace}(\Sigma_2^{-2}\Sigma_1-2\Sigma_2^{-1}+\Sigma_1^{-1})+|\Sigma_2^{-1}(m_1-m_2)|^2. \end{array} \]
The formula when \( {\Sigma_1\Sigma_2=\Sigma_2\Sigma_1} \) follows immediately.
Other distances. Recall that the Hellinger distance between probability measures \( {\mu} \) and \( {\nu} \) with densities \( {f_\mu} \) and \( {f_\nu} \) with respect to the same reference measure \( {\lambda} \) is
\[ \mathrm{Hellinger}(\mu,\nu) =\Bigr(\int(\sqrt{f_\mu}-\sqrt{f_\nu})^2\mathrm{d}\lambda\Bigr)^{1/2} =\Bigr(2-2\int\sqrt{f_\mu f_\nu}\mathrm{d}\lambda\Bigr)^{1/2} \in[0,\sqrt{2}]. \]
This quantity does not depend on the choice of \( {\lambda} \).
The \( {\chi^2} \) divergence (inappropriately called distance) is defined by
\[ \chi^2(\nu\mid\mu) =\mathrm{Var}_\mu\Bigr(\frac{\mathrm{d}\nu}{\mathrm{d}\mu}\Bigr) =\Bigr\|\frac{\mathrm{d}\nu}{\mathrm{d}\mu}-1\Bigr\|_{\mathrm{L}^2(\mu)} =\Bigr\|\frac{\mathrm{d}\nu}{\mathrm{d}\mu}\Bigr\|_{\mathrm{L}^2(\mu)}-1 \in[0,+\infty]. \]
The Kullback—Leibler divergence or relative entropy is defined by
\[ \mathrm{Kullback}(\nu\mid\mu) =\int\log{\textstyle\frac{\mathrm{d}\nu}{\mathrm{d}\mu}}\mathrm{d}\nu =\int{\textstyle\frac{\mathrm{d}\nu}{\mathrm{d}\mu}} \log{\textstyle\frac{\mathrm{d}\nu}{\mathrm{d}\mu}}\mathrm{d}\mu \in[0,+\infty] \]
if \( {\nu} \) is absolutey continuous with respect to \( {\mu} \), and \( {\mathrm{Kullback}(\nu\mid\mu)=+\infty} \) otherwise.
The Wasserstein—Kantorovich—Monge transportation distance of order \( {2} \) and with respect to the underlying Euclidean distance is defined for all probability measures \( {\mu} \) and \( {\nu} \) on \( {\mathbb{R}^n} \) by
\[ \mathrm{Wasserstein}(\mu,\nu)=\Bigr(\inf_{(X,Y)}\mathbb{E}(\left|X-Y\right|^2)\Bigr)^{1/2} \in[0,+\infty] \ \ \ \ \ (1) \]
where the inf runs over all couples \( {(X,Y)} \) with \( {X\sim\mu} \) and \( {Y\sim\nu} \).
Now, for all \( {n\geq1} \), \( {m_1,m_2\in\mathbb{R}^n} \), and all \( {n\times n} \) covariance matices \( {\Sigma_1,\Sigma_2} \), denoting
\[ \Gamma_1=\mathcal{N}(\mu_1,\Sigma_1) \quad\mbox{and}\quad \Gamma_2=\mathcal{N}(\mu_2,\Sigma_2), \]
we have, with \( {m=m_1-m_2} \),
\[ \begin{array}{rcl} \mathrm{Hellinger}^2(\Gamma_1,\Gamma_2) &=&2-2\frac{\det(\Sigma_1\Sigma_2)^{1/4}}{\det(\frac{\Sigma_1+\Sigma_2}{2})^{1/2}}\mathrm{exp}\Bigr(-\frac{1}{4}(\Sigma_1+\Sigma_2)^{-1}m\cdot m\Bigr)\\ \chi^2(\Gamma_1\mid\Gamma_2) &=&\sqrt{\frac{|\Sigma_2|}{|2\Sigma_1-\Sigma_1^2\Sigma_2^{-1}|}}\exp\Bigr(\frac{1}{2}\Sigma_2^{-1}(\mathrm{I}_n+(2\Sigma_1^{-1}\Sigma_2^{-1}-\Sigma_2^{-2}))m\cdot m\Bigr)-1\\ 2\mathrm{Kullback}(\Gamma_1\mid\Gamma_2) &=&\Sigma_2^{-1}m\cdot m+\mathrm{Tr}(\Sigma_2^{-1}\Sigma_1-\mathrm{I}_n)+\log\det(\Sigma_2\Sigma_1^{-1})\\ \mathrm{Fisher}(\Gamma_1\mid\Gamma_2) &=&|\Sigma_2^{-1}m|^2+\mathrm{Tr}(\Sigma_2^{-2}\Sigma_1-2\Sigma_2^{-1}+\Sigma_1^{-1})\\ \mathrm{Wasserstein}^2(\Gamma_1,\Gamma_2) &=&|m|^2+\mathrm{Tr}\Bigr(\Sigma_1+\Sigma_2-2\sqrt{\sqrt{\Sigma_1}\Sigma_2\sqrt{\Sigma_1}}\Bigr), \end{array} \]
and if \( {\Sigma_1} \) and \( {\Sigma_2} \) commute, \( {\Sigma_1\Sigma_2=\Sigma_2\Sigma_1} \), then we find the simpler formulas
\[ \begin{array}{rcl} \mathrm{Fisher}(\Gamma_1\mid\Gamma_2) &=&|\Sigma_2^{-1}(m_1-m_2)|^2+\mathrm{Tr}(\Sigma_2^{-2}(\Sigma_2-\Sigma_1)^2\Sigma_1^{-1})\\ \mathrm{Wasserstein}^2(\Gamma_1,\Gamma_2) &=&|m_1-m_2|^2+\mathrm{Tr}((\sqrt{\Sigma_1}-\sqrt{\Sigma_2})^2). \end{array} \]
Fisher as an infinitesimal Kullback. The Boltzmann—Shannon entropy is in a sense the opposite of the Kullback divergence with respect to the Lebesgue measure \( {\lambda} \), namely
\[ \mathrm{Entropy}(\mu) =-\int\frac{\mathrm{d}\mu}{\mathrm{d}\lambda} \log\frac{\mathrm{d}\mu}{\mathrm{d}\lambda}\mathrm{d}\lambda =\mathrm{Kullback}(\mu\mid\lambda). \]
It was discovered by Nicolaas Govert de Bruijn (1918 — 2012) that the Fisher information appears as the differential version of the entropy under Gaussian noise. More precisely, it states that if \( {X} \) is a random vector of \( {\mathbb{R}^n} \) with finite entropy and if \( {Z\sim\mathcal{N}(0,I_n)} \) then
\[ \frac{\mathrm{d}}{\mathrm{d}t}\Bigr\vert_{t=0} \mathrm{Entropy}(\mathrm{Law}(X+\sqrt{t}Z)\mid\lambda) =-\mathrm{Fisher}(\mathrm{Law}(X)\mid\lambda). \]
In other words, if \( {\mu_t} \) is the law at time \( {t} \) of an \( {n} \)-dimensional Brownian motion started from a random initial condition \( {X} \) then
\[ \frac{\mathrm{d}}{\mathrm{d}t}\Bigr\vert_{t=0} \mathrm{Entropy}(\mu_t\mid\lambda) =-\mathrm{Fisher}(\mu_0\mid\lambda). \]
The Lebesgue measure is the invariant (and reversible) measure of Brownian motion. More generally, let us consider the stochastic differential equation
\[ \mathrm{d}X_t=\sqrt{2}\mathrm{d}B_t-\nabla V(X_t)\mathrm{d}t \]
on \( {\mathbb{R}^n} \), where \( {V:\mathbb{R}^n\mapsto\mathbb{R}} \) is \( {\mathcal{C}^2} \) and where \( {{(B_t)}_{t\geq0}} \) is a standard Brownian motion. If we assume that \( {V-\frac{\rho}{2}\left|\cdot\right|^2} \) is convex for some \( {\rho\in\mathbb{R}} \) then it admits a solution \( {{(X_t)}_{t\geq0}} \) known as the overdamped Langevin process, which is a Markov diffusion process. If we further assume that \( {\mathrm{e}^{-V}} \) is integrable with respect to the Lebesgue measure, then the probability measure \( {\mu} \) with density proportional to \( {\mathrm{e}^{-V}} \) is invariant and reversible. Now, denoting \( {\mu_t=\mathrm{Law}(X_t)} \), the analogue of the De Bruijn identity reads, for all \( {t\geq0} \),
\[ \frac{\mathrm{d}}{\mathrm{d}t} \mathrm{Kullback}(\mu_t\mid\mu) =-\mathrm{Fisher}(\mu_t\mid\mu) \]
but this requires that \( {\mu_0} \) is chosen in such a way that \( {t\mapsto\mathrm{Kullback}(\mu_t\mid\mu)} \) is well defined and differentiable. This condition is easily checked in the example of the Ornstein—Uhlenbeck process which corresponds to \( {V=\frac{1}{2}\left|\cdot\right|^2} \) and for which \( {\mu=\mathcal{N}(0,I_n)} \).
Ornstein–Uhlenbeck. If \( {{(X_t^x)}_{t\geq0}} \) is an \( {n} \)-dimensional Ornstein–Uhlenbeck process solution of the stochastic differential equation
\[ X_0^x=x\in\mathbb{R}^n, \quad\mathrm{d}X^x_t=\sqrt{2}\mathrm{d}B_t-X^x_t\mathrm{d}t \]
where \( {{(B_t)}_{t\geq0}} \) is a standard \( {n} \)-dimensional Brownian motion, then the invariant law is \( {\gamma=\mathcal{N}(0,I_n)} \) and the Mehler formula reads
\[ X^x_t=x\mathrm{e}^{-t}+\int_0^t\mathrm{e}^{s-t}\mathrm{d}B_s\sim\mathcal{N}(x\mathrm{e}^{-t},(1-\mathrm{e}^{-2t})I_n), \]
and the explicit formula for the Fisher information for Gaussians gives
\[ \mathrm{Fisher}(\mathrm{Law}(X^x_t)\mid\gamma) =\mathrm{Fisher}(\mathcal{N}(x\mathrm{e}^{-t},(1-\mathrm{e}^{-2t})I_n)\mid\gamma) =|x|^2\mathrm{e}^{-2t}+n\frac{\mathrm{e}^{-4t}}{1-\mathrm{e}^{-2t}}. \]
Log-Sobolev inequality. The optimal log-Sobolev inequality for \( {\mu=\mathcal{N}(0,\mathrm{I}_n)} \) writes
\[ \mathrm{Kullback}(\nu\mid\mu) \leq\frac{1}{2}\mathrm{Fisher}(\nu\mid\mu) \]
for all probability measure \( {\nu} \) on \( {\mathbb{R}^n} \), and equality is achieved when \( {\log\frac{\mathrm{d}\nu}{\mathrm{d}\mu}} \) is linear, namely when \( {\nu=\mathcal{N}(m,\mathrm{I}_n)} \) for some \( {m\in\mathbb{R}^n} \). By using the Gaussian formulas above for Kullback and Fisher, this log-Sobolev inequality boils down when \( {\nu=\mathcal{N}(m,\Sigma_1)} \) to
\[ \log\det(\Sigma_1^{-1})\leq\mathrm{Tr}(\Sigma_1^{-1}-\mathrm{I}_n). \]
Taking \( {K=\Sigma_1^{-1}} \) shows that this is a matrix version of \( {\log(x)\leq x-1} \), nothing else.
Note. This post was written while working on
- Universal cutoff for Dyson Ornstein Uhlenbeck process
By Boursier, Chafaï, and Labbé (2021)
Further reading.
- About the Hellinger distance
(on this blog) - Wasserstein distance between two Gaussians
(on this blog) - Aspects of the Ornstein-Uhlenbeck process
(on this blog) - Probability metrics and the stability of stochastic models
by Svetlozar T. Rachev (1991) - On choosing and bounding probability metrics
by Alison L. Gibbs and Francis Edward Su (2002) - Statistical inference based on divergence measures
by Leandro Pardo (2006)
Super!!
on en a souvent besoin et la flemme de les recalculer! Merci Djalil!!
un truc qui aurait ete sympa, c’est que nous avons de belles inegalites a partir des gaussiennes : log-Sobolev, transport-information (soit Kullback, soit Fisher)… et qui marche donc ente deux gaussiennes… ca donne alors des comparaisons entre ces expressions! en dim 1, ca doit pas etre trop dur de les comparer a la main. mais en dim plus grande..???
Salut Arnaud ! C’est drôle, je me suis fait la même réflexion en rédigeant ce billet (LSI, TI, HWI gaussiennes multivariées restreintes aux gaussiennes), et je vais peut-être y consacrer un futur billet ou de mettre à jour celui-ci 😉 J’ai rajouté à la fin du billet un commentaire pour LSI gaussien. Cela fait aussi penser par ailleurs au papier de Elliott Lieb intitulé Gaussian kernels have only Gaussian maximizers.