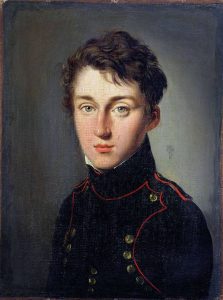
Why and how entropy emerges in basic mathematics? This tiny post aims to provide some answers. We have already tried something in this spirit in a previous post almost ten years ago.
Combinatorics. Asymptotic analysis of the multinomial coefficient $\binom{n}{n_1,\ldots,n_r}:=\frac{n!}{n_1!\cdots n_r!}$ :
\[
\frac{1}{n}\log\binom{n}{n_1,\ldots,n_r}
\xrightarrow[n=n_1+\cdots+n_r\to\infty]{\nu_i=\frac{n_i}{n}\to p_i}
\mathrm{S}(p):=-\sum_{i=1}^rp_i\log(p_i).
\] Recall that if $A=\{a_1,\ldots,a_r\}$ is a finite set of cardinal $r$ and $n=n_1+\cdots+n_r$ then
\[
\mathrm{Card}\Bigr\{(x_1,\ldots,x_n)\in A^n:\forall 1\leq i\leq r,\sum_{k=1}^n\mathbf{1}_{x_k=a_i}=n_i\Bigr\}=\binom{n}{n_1,\ldots,n_r}.
\] The multinomial coefficient can be interpreted as the number of microstates $(x_1,\ldots,x_n)$ compatible with the macrostate $(n_1,\ldots,n_r)$, while the quantity $\mathrm{S}(p)$ appears as a normalized asymptotic measure of additive degrees of freedom or disorder. This is already in the work of Ludwig Eduard Boltzmann (1844 — 1906) in kinetic gas theory at the origins of statistical physics. The quantity $\mathrm{S}(p)$ is also the one used by Claude Elwood Shannon (1916 — 2001) in information and communication theory as the average length of optimal lossless coding. It is characterized by the following three natural axioms or properties, denoting $\mathrm{S}^{(n)}$ to remember $n$ :
- for all $n\geq1$, $p\mapsto\mathrm{S}^{(n)}(p)$ is continuous
- for all $n\geq1$, $\mathrm{S}^{(n)}(\frac{1}{n},\ldots,\frac{1}{n})<\mathrm{S}^{(n+1)}(\frac{1}{n+1},\ldots,\frac{1}{n+1})$
- for all $n=n_1+\cdots+n_r\geq1$, $\mathrm{S}^{(n)}(\frac{1}{n},\ldots,\frac{1}{n})=\mathrm{S}^{(r)}(\frac{n_1}{n},\ldots,\frac{n_r}{n})+\sum_{i=1}^r\frac{n_i}{n}\mathrm{S}^{(n_i)}(\frac{1}{n_i},\ldots,\frac{1}{n_i}).$
Probability. If $X_1,\ldots,X_n$ are independent and identically distributed random variables of law $\mu$ on a finite set or alphabet $A=\{a_1,\ldots,a_r\}$, then for all $x_1,\ldots,x_n\in A$,
\begin{align*}
\mathbb{P}((X_1,\ldots,X_n)=(x_1,\ldots,x_n))
&=\prod_{i=1}^r\mu_i^{\sum_{k=1}^n\mathbb{1}_{x_k=a_i}}
=\prod_{i=1}^r\mu_i^{n\nu_i}
=\mathrm{e}^{n\sum_{i=1}^n\nu_i\log\mu_i}\\
&=\mathrm{e}^{-n(\mathrm{S}(\nu)+\mathrm{H}(\nu\mid\mu))},
\end{align*} a remarkable identity where $\mathrm{S}(\nu)$ is the Boltzmann-Shannon entropy considered before, and where $\mathrm{H}(\nu\mid\mu)$ is a new quantity known as the Kullback-Leibler divergence or relative entropy :
\[
\mathrm{S}(\nu):=-\sum_{i=1}^r\nu_i\log\nu_i=-\int f(x)\log f(x)\mathrm{d}x
\] where $f$ is the density of $\nu$ with respect to the counting measure $\mathrm{d}x$, and
\[
\mathrm{H}(\nu\mid\mu):=\sum_{i=1}^r\nu_i\log\frac{\nu_i}{\mu_i}
=\sum_{i=1}^r\frac{\nu_i}{\mu_i}\log\frac{\nu_i}{\mu_i}\mu_i
=\int\frac{\mathrm{d}\nu}{\mathrm{d}\mu}\log\frac{\mathrm{d}\nu}{\mathrm{d}\mu}\mathrm{d}\mu.
\] This comes from information theory after Solomon Kullback (1907 – 1994) and Richard Leibler (1914 — 2003). Here $\mathrm{S}(\nu)$ measures the combinatorics on $x_1,\ldots,x_n$ at prescribed frequencies $\nu$, while $\mathrm{H}(\nu\mid\mu)$ measures the cost or energy of deviation from the actual distribution $\mu$. This is a Boltzmann–Gibbsfication of the probability $\mathbb{P}((X_1,\ldots,X_n)=(x_1,\ldots,x_n))$, see below, leading via the Laplace method to the large deviations principle of Ivan Nikolaevich Sanov (1919 — 1968). The Jensen inequality for the strictly convex function $u\mapsto u\log(u)$ gives
\[
\mathrm{H}(\nu\mid\mu)\geq0\quad\text{with equality iff}\quad\nu=\mu.
\]
Statistics. If $Y_1,\ldots,Y_n$ are independent and identically distributed random variables of law $\mu^{(\theta)}$ in parametric family parametrized by $\theta$, on a finite set $A$, then, following Ronald Aylmer Fisher (1890 – 1962), the likelihood of data $(x_1,\ldots,x_n)\in A^n$ is
\[
\ell_{x_1,\ldots,x_n}(\theta):=\mathbb{P}(Y_1=x_1,\ldots,Y_n=x_n)
=\prod_{i=1}^n\mu^{(\theta)}_{x_i}.
\] It can also be seen as the likelihood of $\theta$ with respect to $x_1,\ldots,x_n$. This dual point of view leads to the following : if $X_1,\ldots,X_n$ is an observed sample of $\mu^{(\theta_*)}$ with $\theta_*$ unknown then the maximum likelihood estimator of $\theta_*$ is
\[
\widehat{\theta}_n:=\arg\max_{\theta\in\Theta}\ell_{X_1,\ldots,X_n}(\theta)
=\arg\max_{\theta\in\Theta}\Bigr(\frac{1}{n}\log\ell_{X_1,\ldots,X_n}(\theta)\Bigr).
\] The asymptotic analysis via the law of large numbers reveals entropy as asymptotic contrast
\begin{align*}
\frac{1}{n}\log\ell_{X_1,\ldots,X_n}(\theta)
&=\frac{1}{n}\sum_{i=1}^n\log\mu^{(\theta)}_{X_i}\\&\xrightarrow[n\to\infty]{\mathrm{a.s.}}
\sum_{k=1}^r\mu^{(\theta_*)}_k\log\mu^{(\theta)}_k
=\underbrace{-\mathrm{S}(\mu^{(\theta_*)})}_{\text{const}}-\mathrm{H}(\mu^{(\theta_*)}\mid\mu^{(\theta)}).
\end{align*}
Analysis. The entropy appears naturally as a derivative of the $L^p$ norm of $f\geq0$ as follows:
\[
\partial_p\|f\|_p^p
=\partial_p\int f^p\mathrm{d}\mu
=\partial_p\int \mathrm{e}^{-p\log(f)}\mathrm{d}\mu
=\int f^p\log(f)\mathrm{d}\mu
=\frac{1}{p}\int f^p\log(f^p)\mathrm{d}\mu.
\] This is at the heart of the Leonard Gross (1931 — ) theorem relating the hypercontractivity of Markov semigroups with the logarithmic Sobolev inequality for the invariant measure. This can also be used to extract from the William Henry Young (1843 – 1942) convolution inequalities certain entropic uncertainty principles.
Boltzmann-Gibbs measures, variational characterizations, and Helmholtz free energy. We take $V:A\to\mathbb{R}$, interpreted as an energy. Maximizing $\mu\mapsto\mathrm{S}(\mu)$ over the constraint of average energy $\int V\mathrm{d}\mu=v$ gives the maximizer \[
\mu_\beta
:=\frac{1}{Z_\beta}\mathrm{e}^{-\beta V}\mathrm{d}x
\quad\text{where}\quad
Z_\beta:=\int\mathrm{e}^{-\beta V}\mathrm{d}x.
\] We use integrals instead of sums to lightnen notation. The notation $\mathrm{d}x$ stands for the counting measure on $A$. The parameter $\beta>0$, interpreted as inverse temperature, is dictated by $v$. Such a probability distribution $\mu_\beta$ is known as a Boltzmann-Gibbs distribution, after Ludwig Eduard Boltzmann (1844 – 1906) and Josiah Willard Gibbs (1839 – 1903). We have a variational characterization as a maximum entropy at fixed average energy :
\[
\int V\mathrm{d}\mu=\int V\mathrm{d}\mu_\beta
\quad\Rightarrow\quad
\mathrm{S}(\mu_\beta)-\mathrm{S}(\mu)
=\mathrm{H}(\mu\mid\mu_\beta).
\] There is a dual point of view in which instead of fixing the average energy, we fix the inverse temperature $\beta$ and we introduce the Hermann von Helmholtz (1821 – 1894) free energy
\[
\mathrm{F}(\mu):=\int V\mathrm{d}\mu-\frac{1}{\beta}\mathrm{S}(\mu)
\] This can be seen as a Joseph-Louis Lagrange (1736 – 1813) point of view in which the constraint is added to the functional. We have
\[
\mathrm{F}(\mu_\beta)=-\frac{1}{\beta}\log(Z_\beta)
\quad\text{since}\quad
\mathrm{S}(\mu_\beta)=\beta\int V\mathrm{d}\mu_\beta+\log Z_\beta.
\] We have then a new variational characterization as a minimum free energy at fixed temperature :
\[
\mathrm{F}(\mu)-\mathrm{F}(\mu_\beta)=\frac{1}{\beta}\mathrm{H}(\mu\mid\mu_\beta).
\] This explains why $\mathrm{H}$ is often called free energy.
Legrendre transform. The relative entropy $\nu\mapsto\mathrm{H}(\nu\mid\mu)$ is the Legendre transform of the log-Laplace transform, in the sense that
\[
\sup_g\Bigr\{\int g\mathrm{d}\nu-\log\int\mathrm{e}^g\mathrm{d}\mu\Bigr\}=\mathrm{H}(\nu\mid\mu).
\] Indeed, for all $h$ such that $\int\mathrm{e}^h\mathrm{d}\mu=1$, by the Jensen inequality, with $f:=\frac{\mathrm{d}\nu}{\mathrm{d}\mu}$,
\begin{align*}
\int h\mathrm{d}\nu
&=\int f\log(f)\mathrm{d}\mu+\int\log\frac{\mathrm{e}^h}{f}f\mathrm{d}\mu\\
&\leq\int f\log(f)\mathrm{d}\mu+\log\int\mathrm{e}^h\mathrm{d}\mu
=\int f\log(f)\mathrm{d}\mu=\mathrm{H}(\nu\mid\mu),
\end{align*} and equality is achieved for $h=\log f$. It remains to reparametrize with $h=g-\log\int\mathrm{e}^g\mathrm{d}\mu$. Conversely, the Legendre transform of the relative entropy is the log-Laplace transform :
\[
\sup_{\nu}
\Bigr\{\int g\mathrm{d}\nu-\mathrm{H}(\nu\mid\mu)\Bigr\}=\log\int\mathrm{e}^g\mathrm{d}\mu.
\] This is an instance of the convex duality for the convex functional $\nu\mapsto\mathrm{H}(\nu\mid\mu)$.
Same story for $-\mathrm{S}$ which is convex as a function of the Lebesgue density of its argument.
Heat equation. The heat equation $\partial_tf_t=\Delta f_t$ is the gradient flow of entropy :$$\partial_t\int f_t\log(f_t)\mathrm{d}x=-\int\frac{\|\nabla f_t\|^2}{f_t}\mathrm{d}x$$where we used integration by parts, the right hand side is the Fisher information. In other words, the entropy is a Lyapunov function for the heat equation seen as an infinite dimensional ODE.
Further reading.
- Boltzmann-Gibbs entropic variational principle
On this blog (2022) - Entropy ubiquity
On this blog (2015) - Bosons and fermions
On this blog (2012)