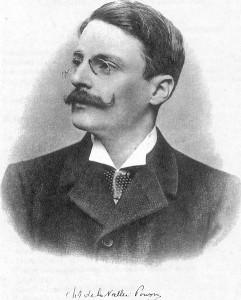
« La Belgique, victime de cruelles violences, a rencontré, dans ses épreuves, de grands et de nombreux témoignages de sympathie. L’Université de Louvain en a recueilli sa part. Ses professeurs, dispersés après le désastre de la ville, ont été invités dans des Universités étrangères. Beaucoup y ont trouvé un asile, quelques-uns même la possibilité de poursuivre leur enseignement. Ainsi, j’ai été appelé a faire des conférences à l’Université Harvard en Amérique l’année dernière, puis cette année au Collège de France. Le présent volume contient la matière des Leçons que j’ai faites au Collège de France entre décembre 1915 et mars 1916. Il est, après bien d’autres, un modeste souvenir de ces événements. … Une partie des résultats établis dans cet article se trouvaient dans la troisième édition du Tome II de mon Cours d’Analyse, qui était sous presse lors de l’incendie de Louvain. Les Allemands ont brulé cet ouvrage : toutes les installations de mon éditeur ont, en effet, partagé le sort de la bibliothèque de l’Université. … » Charles de la Vallée Poussin in Intégrales de Lebesgue, fonctions d’ensemble, classes de Baire, Leçons professées au Collège de France (1916). Excerpt from the introduction.
This post is devoted to some probabilistic aspects of uniform integrability, a basic concept that I like very much. Let \( {\Phi} \) be the class of non-decreasing functions \( {\varphi:\mathbb{R}_+\rightarrow\mathbb{R}_+} \) such that
\[ \lim_{x\rightarrow+\infty}\frac{\varphi(x)}{x}=+\infty. \]
This class contains for instance the convex functions \( {x\mapsto x^p} \) with \( {p>1} \) and \( {x\mapsto x\log(x)} \). Let us fix a probability space \( {(\Omega,\mathcal{A},\mathbb{P})} \), and denote by \( {L^\varphi} \) the set of random variables \( {X} \) such that \( {\varphi(|X|)\in L^1=L^1((\Omega,\mathcal{F},\mathbb{P}),\mathbb{R})} \). We have \( {L^\varphi\subsetneq L^1} \). Clearly, if \( {{(X_i)}_{i\in I}\subset L^1} \) is bounded in \( {L^\varphi} \) with \( {\varphi\in\Phi} \) then \( {{(X_i)}_{i\in I}} \) is bounded in \( {L^1} \).
Uniform integrability. For any family \( {{(X_i)}_{i\in I}\subsetneq L^1} \), the following three properties are equivalent. When one (and thus all) of these properties holds true, we say that the family \( {{(X_i)}_{i\in I}} \) is uniformly integrable (UI). The first property can be seen as a natural definition of uniform integrability.
- (definition of UI) \( {\lim_{x\rightarrow+\infty}\sup_{i\in I}\mathbb{E}(|X_i|\mathbf{1}_{|X_i|\geq x})=0} \);
- (epsilon-delta) the family is bounded in \( {L^1} \): \( {\sup_{i\in I}\mathbb{E}(|X_i|)<\infty} \), and moreover \( {\forall\varepsilon>0} \), \( {\exists\delta>0} \), \( {\forall A\in\mathcal{F}} \), \( {\mathbb{P}(A)\leq\delta\Rightarrow\sup_{i\in I}\mathbb{E}(|X_i|\mathbf{1}_A)\leq\varepsilon} \);
- (de la Vallée Poussin) there exists \( {\varphi\in\Phi} \) such that \( {\sup_{i\in I}\mathbb{E}(\varphi(|X_i|))<\infty} \).
The second property is often referred to as the “epsilon-delta” criterion. The third and last property is a boundedness in \( {L^\varphi\subsetneq L^{1}} \) and is due to Charles-Jean Étienne Gustave Nicolas de la Vallée Poussin (1866 – 1962), a famous Belgian mathematician, also well known for his proof of the prime number theorem.
Proof of \( {1\Rightarrow2} \). For the boundedness in \( {L^1} \), we write, for any \( {i\in I} \), and some \( {x\geq0} \) large enough,
\[ \mathbb{E}(|X_i|) \leq \mathbb{E}(|X_i|\mathbf{1}_{|X_i|<x})+\mathbb{E}(|X_i|\mathbf{1}_{|X_i|\geq x}) \leq x+\sup_{i\in I}\mathbb{E}(|X_i|\mathbf{1}_{|X_i|\geq x})<\infty. \]
Next, by assumption, for any \( {\varepsilon>0} \), there exists \( {x_\varepsilon} \) such that \( {\sup_{i\in I}\mathbb{E}(|X_i|\mathbf{1}_{|X_i|\geq x})\leq\varepsilon} \) for any \( {x\geq x_\varepsilon} \). If \( {\mathbb{P}(A)\leq \delta_\varepsilon:=\varepsilon/x_\varepsilon} \) then for any \( {i\in I} \),
\[ \mathbb{E}(|X_i|\mathbf{1}_A) =\mathbb{E}(|X_i|\mathbf{1}_{|X_i|<x_\varepsilon}\mathbf{1}_A) +\mathbb{E}(|X_i|\mathbf{1}_{|X_i|\geq x_\varepsilon}\mathbf{1}_A) \leq x_\varepsilon\mathbb{P}(A)+\varepsilon\leq 2\varepsilon. \]
Proof of \( {2\Rightarrow1} \). Since \( {{(X_i)}_{i\in I}} \) is bounded in \( {L^1} \), for every \( {j\in I} \), if \( {A:=A_j:=\{|X_j|\geq x\}} \), then, by Markov inequality, \( {\mathbb{P}(A)\leq x^{-1}\sup_{i\in I}\mathbb{E}(|X_i|)\leq \delta} \) for \( {x} \) large enough, uniformly in \( {j\in I} \), and the assumption gives \( {\lim_{x\rightarrow+\infty}\sup_{i,j\in I}\mathbb{E}(|X_i|\mathbf{1}_{|X_j|\geq x})=0} \).
Proof of \( {3\Rightarrow1} \). For any \( {\varepsilon>0} \), since \( {\varphi\in\Phi} \), by definition of \( {\Phi} \) there exists \( {x_\varepsilon\geq0} \) such that \( {x\leq\varepsilon \varphi(x)} \) for every \( {x\geq x_\varepsilon} \), and therefore
\[ \sup_{i\in I}\mathbb{E}(|X_i|\mathbf{1}_{|X_i|\geq x_\varepsilon}) \leq \varepsilon\sup_{i\in I}\mathbb{E}(\varphi(|X_i|)\mathbf{1}_{|X_i|\geq x_\varepsilon}) \leq \varepsilon\sup_{i\in I}\mathbb{E}(\varphi(|X_i|)). \]
Proof of \( {1\Rightarrow 3} \). Let us seek for a piecewise linear function \( {\varphi} \) of the form
\[ \varphi(x)=\int_0^x\varphi'(t)dt \quad\mbox{with}\quad \varphi’=\sum_{n=1}^\infty u_n\mathbf{1}_{[n,n+1[} \quad\mbox{and}\quad u_n=\sum_{m\geq1}\mathbf{1}_{x_m\leq n} \]
for a sequence \( {{(x_m)}_{m\geq1}\nearrow+\infty} \) to be constructed. We have \( {\varphi\in\Phi} \) since \( {\lim_{n\rightarrow\infty}u_n=+\infty} \). Moreover, since \( {\varphi\leq\sum_{n=1}^\infty(u_1+\cdots+u_n)\mathbf{1}_{[n,n+1[}} \), we get, for any \( {i\in I} \),
\[ \begin{array}{rcl} \mathbb{E}(\varphi(|X_i|)) &\leq& \sum_{n=1}^\infty (u_1+\cdots+u_n)\mathbb{P}(n\leq |X_i|<n+1) \\ &=& \sum_{n=1}^\infty u_n\mathbb{P}(|X_i|\geq n)\\ &=& \sum_{m\geq1}\sum_{n\geq x_m}\mathbb{P}(|X_i|\geq n)\\ &\leq & \sum_{m\geq1}\sum_{n\geq x_m}n\mathbb{P}(n\leq |X_i|<n+1)\\ &\leq & \sum_{m\geq1}\mathbb{E}(|X_i|\mathbf{1}_{|X_i|\geq x_m})\\ &\overset{*}{\leq} & \sum_{m\geq1}2^{-m}<\infty \end{array} \]
where \( {\overset{*}{\leq}} \) holds if for every \( {m} \) we select \( {x_m} \) such that \( {\sup_{i\in I}\mathbb{E}(|X_i|\mathbf{1}_{|X_i|\geq x_m})\leq 2^{-m}} \), which is allowed by assumption (we may replace \( {2^{-m}} \) by anything sumable in \( {m} \)).
This achieves the proof of the equivalence of the three properties: \( {1\Leftrightarrow 2\Leftrightarrow 3} \).
Alternative proof of \( {1\Rightarrow 3} \). Following a suggestion made by Nicolas Fournier on an earlier version of this post, one can simply construct \( {\varphi} \) as follows:
\[ \varphi(x)=\sum_{m\geq 1} (x-x_m)_+. \]
This function is non-decreasing and convex, and
\[ \frac{\varphi(x)}{x} = \sum_{m\geq1} \left(1-\frac{x_m}{x}\right)_+ \underset{x\rightarrow\infty}{\nearrow} \sum_{m\geq1} 1=\infty. \]
It remains to note that
\[ \mathbb{E}(\varphi(|X_i|)) \leq \sum_{m\geq1}\mathbb{E}(|X_i|\mathbf{1}_{|X_i|\geq x_m}) \leq 1. \]
Convexity and moderate growth. In the de la Vallée Poussin criterion, one can always assume that the function \( {\varphi} \) is convex (in particular continuous), with moderate growth in the sense that for every \( {x\geq0} \),
\[ \varphi(x)\leq x^2. \]
Indeed, the construction of \( {\varphi} \) that we gave above provides a function \( {\varphi} \) with piecewise constant and non-decreasing derivative, thus the function is convex (it is a Young function). Following Paul-André Meyer, to derive the moderate growth property, we may first observe that thanks to the way we constructed \( {\varphi} \), for every \( {x\geq0} \),
\[ \varphi'(2x)\leq c\varphi'(x) \]
where one can take \( {c=2} \). This follows from the fact that we have the freedom to take the \( {x_m} \)’s as large as we want, for instance in such a way that for every \( {n\geq1} \),
\[ u_{2n}\leq u_n+\sum_{m\geq1}\mathbf{1}_{n<x_m\leq 2n}\leq 2u_n. \]
Consequently, the function \( {\varphi} \) itself has also moderate growth since, denoting \( {C:=2c} \),
\[ \varphi(2x)=\int_0^{2x}\!g(u)\,du=\int_0^x\!g(2t)\,2dt\leq 2c\varphi(x)=C\varphi(x). \]
Now \( {\varphi(x)\leq C^{1+k}\varphi(2^{-k}x)} \) for any \( {k\geq1} \), and taking \( {k=k_x=\lceil\log_2(x)\rceil} \) we obtain
\[ \varphi(x)\leq C^2C^{\log_2(x)}\varphi(1)=C^2\varphi(1)2^{\log_2(C)\log_2(x)}=C^2\varphi(1)x^{\log_2(C)}. \]
Since one can take \( {c=2} \) we get \( {C=4} \), which allows \( {\varphi(x)\leq x^2} \) by scaling.
Examples of UI families.
- Any finite subset of \( {L^1} \) is UI;
- More generally, if \( {\sup_{i\in I}|X_i|\in L^1} \) (domination: \( {|X_i|\leq X} \) for every \( {i\in I} \), with \( {X\in L^1} \)) then \( {{(X_i)}_{i\in I}} \) is UI. To see it, we may first observe that the singleton family \( {\{\sup_{i\in I}|X_i|\}} \) is UI, and thus, by the de la Vallée Poussin criterion, there exists \( {\varphi\in\Phi} \) such that \( {\varphi(\sup_{i\in I}|X_i|)\in L^1} \), and therefore
\[ \sup_{i\in I}\mathbb{E}(\varphi(|X_i|))\leq\mathbb{E}(\sup_{i\in I}\varphi(|X_i|))\leq\mathbb{E}(\varphi(\sup_{i\in I}|X_i|))<\infty, \]
which implies, by the de la Vallée Poussin criterion again, that \( {{(X_i)}_{i\in I}} \) is UI;
- If \( {\mathcal{U}_1\subset L^1,\ldots,\mathcal{U}_n\subset L^1} \) is a finite collection of UI families then the union \( {\mathcal{U}_1\cup\cdots\cup\mathcal{U}_n} \) is UI.
- If \( {\mathcal{U}} \) is UI then its convex envelope (or convex hull) is UI. Beware however that the vector span of \( {\mathcal{U}} \) is not UI in general.
- If \( {{(X_n)}_{n\geq1},X\in L^1} \) and \( {X_n\overset{L^1}{\rightarrow} X} \) then \( {{(X_n)}_{n\geq1}\cup\{X\}} \) is UI and \( {{(X_n-X)}_{n\geq1}} \) is UI. To see it, for any \( {\varepsilon>0} \), we first select \( {n} \) large enough such that \( {\sup_{k\geq n}\mathbb{E}(|X_k-X|)\leq\varepsilon} \), and then \( {\delta>0} \) with the epsilon-delta criterion for the finite family \( {\{X_1,\ldots,X_n\}} \), which gives, for any \( {A\in\mathcal{F}} \) such that \( {\mathbb{P}(A)\leq\delta} \),
\[ \sup_{n\geq1}\mathbb{E}(|X_n-X|\mathbf{1}_A) \leq \max\left(\max_{1\leq k\leq n}\mathbb{E}(|X_k-X|\mathbf{1}_A) ; \sup_{k\geq n}\mathbb{E}(|X_k-X|) \right) \leq \varepsilon. \]
- The de la Vallée Poussin criterion is often used with \( {\varphi(x)=x^2} \), and means in this case that every bounded subset of \( {L^2} \) is UI.
Integrability. The de la Vallée Poussin criterion, when used with a singleton UI family \( {\{X\}} \), states that \( {X\in L^1} \) implies that \( {\varphi(|X|)\in L^1} \) for some \( {\varphi\in\Phi} \). In other words, for every random variable, integrability can always be improved in a sense. There is not any paradox here since \( {\varphi} \) depends actually on \( {X} \). Topologically, integrability is in a sense an open statement rather than a closed statement. An elementary instance of this phenomenon is visible for Riemann series, in the sense that if \( {\sum_{n\geq1}n^{-s}<\infty} \) for some \( {s>0} \) then \( {\sum_{n\geq1}n^{-s’}<\infty} \) for some \( {s'<s} \), because the convergence condition of the series is “\( {s>1} \)”, which is an open condition.
Integrability of the limit. If \( {{(X_n)}_{n\geq1}} \) is UI and \( {X_n\rightarrow X} \) almost surely as \( {n\rightarrow\infty} \) then \( {X\in L^1} \). Indeed, by the Fatou Lemma,
\[ \mathbb{E}(|X|) =\mathbb{E}(\varliminf_{n\rightarrow\infty}|X_n|) \leq\varliminf_{n\rightarrow\infty}\mathbb{E}(|X_n|) \leq\sup_{n\geq1}\mathbb{E}(|X_n|)<\infty. \]
Dominated convergence. For any \( {{(X_n)}_{n\geq1},X\in L^1} \), we have
\[ X_n\overset{ L^1}{\rightarrow}X \quad\text{if and only if}\quad X_n\overset{\mathbb{P}}{\rightarrow}X \text{ and } {(X_n)}_{n\geq1}\text{ is UI} \]
and in fact, for any \( {{(X_n)}_{n\geq1}\in L^1} \) and any random variable \( {X} \),
\[ X\in L^1\text{ and }X_n\overset{ L^1}{\rightarrow}X \quad\text{if and only if}\quad X_n\overset{\mathbb{P}}{\rightarrow}X \text{ and } {(X_n)}_{n\geq1}\text{ is UI}. \]
This can be seen as an improved dominated convergence theorem (since \( {{(X_n)}_{n\geq1}} \) is UI when \( {\sup_n|X_n|\in L^1} \)). The proof may go as follows. We already know that if \( {X_n\rightarrow X} \) in \( {L^1} \) then \( {X_n\rightarrow X} \) in probability (Markov inequality) and \( {{(X_n)}_{n\geq1}\cup\{X\}} \) is UI (see above). Conversely, if \( {X_n\rightarrow X} \) in probability and \( {{(X_n)}_{n\geq1}} \) is UI, then \( {{(X_n-X)}_{n\geq1}} \) is UI since \( {X\in L^1} \), and thus, using the convergence in probability and the epsilon-delta criterion, we obtain that for any \( {\varepsilon>0} \) and large enough \( {n} \),
\[ \mathbb{E}(|X_n-X|) =\mathbb{E}(|X_n-X|\mathbf{1}_{|X_n-X|\geq\varepsilon}) +\mathbb{E}(|X_n-X|\mathbf{1}_{|X_n-X|<\varepsilon}) \leq 2\varepsilon. \]
Martingales. The American mathematician Joseph Leo Doob (1910 – 2004) has shown that if a sub-martingale \( {{(M_n)}_{n\geq1}} \) is bounded in \( {L^1} \) then there exists \( {M_\infty\in L^1} \) such that \( {M_n\rightarrow M_\infty} \) almost surely. Moreover, in the case of martingales, this convergence holds also in \( {L^1} \) if and only if \( {{(M_n)}_{n\geq1}} \) is UI. In the same spirit, and a bit more precisely, if \( {{(M_n)}_{n\geq1}} \) is a martingale for a filtration \( {{(\mathcal{F}_n)}_{n\geq1}} \), then the following two properties are equivalent:
- \( {{(M_n)}_{n\geq1}} \) is UI;
- \( {{(M_n)}_{n\geq1}} \) is closed, meaning that there exists \( {M_\infty\in L^1} \) such that \( {M_n=\mathbb{E}(M_\infty|\mathcal{F}_n)} \) for all \( {n\geq1} \), and moreover \( {M_n\rightarrow M_\infty} \) almost surely and in \( {L^1} \).
Are there non UI martingales? Yes, but they are necessarily unbounded: \( {\sup_{n\geq0}|M_n|\not\in L^1} \), otherwise we may apply dominated convergence. A nice counter example is given by critical Galton-Watson branching process, defined recursively by \( {M_0=1} \) and
\[ M_{n+1}=X_{n+1,1}+\cdots+X_{n+1,M_n}, \]
where \( {X_{n+1,j}} \) is the number of offspring of individual \( {j} \) in generation \( {n} \), and \( {{(X_{j,k})}_{j,k\geq1}} \) are i.i.d. random variables on \( {\mathbb{N}} \) with law \( {\mu} \) of mean \( {1} \) and such that \( {\mu(0)>0} \). The sequence \( {{(M_n)}_{n\geq1}} \) is a non-negative martingale, and thus it converges almost surely to some \( {M_\infty\in L^1} \). It is also a Markov chain with state space \( {\mathbb{N}} \). The state \( {0} \) is absorbing and all the remaining states can lead to \( {0} \) and are thus transient. It follows then that almost surely, either \( {{(M_n)}_{n\geq0}} \) converges to \( {0} \) or to \( {+\infty} \), and since \( {M_\infty \in L^1} \), it follows that \( {M_\infty=0} \). However, the convergence cannot hold in \( {L^1} \) since this leads to the contradiction \( {1=\mathbb{E}(M_n)\rightarrow\mathbb{E}(M_\infty)=0} \) (note that \( {{(M_n)}_{n\geq1}} \) is bounded in \( {L^1} \)).
Topology. The Dunford-Pettis theorem, due to the American mathematicians Nelson James Dunford (1906 – 1986) and Billy James Pettis (1913 – 1979), states that for every family \( {{(X_i)}_{i\in I}\in L^1} \), the following propositions are equivalent.
- \( {{(X_i)}_{i\in I}} \) is UI;
- \( {{(X_i)}_{i\in I}} \) is relatively compact for the weak \( {\sigma(L^1,L^\infty)} \) topology;
- \( {{(X_i)}_{i\in I}} \) is relatively sequentially compact for the weak \( {\sigma(L^1,L^\infty)} \) topology.
The proof, which is not given in this post, can be found for instance in Delacherie and Meyer or in Diestel. We just recall that a sequence \( {{(X_n)}_{n\geq1}} \) in \( {L^1} \) converges to \( {X\in L^1} \) for the weak \( {\sigma(L^1,L^\infty)} \) topology when \( {\lim_{n\rightarrow\infty}\ell(X_n)=\ell(X)} \) for every \( {\ell\in (L^1)’=L^\infty} \), in other words when \( {\lim_{n\rightarrow\infty}\mathbb{E}(YX_n)=\mathbb{E}(YX)} \) for every \( {Y\in L^\infty} \).
The Dunford-Pettis theorem opens the door for the fine analysis of closed (possibly linear) subsets of \( {L^1} \), a deep subject in functional analysis and Banach spaces.
Tightness. If \( {{(X_i)}_{i\in I}\subset L^1} \) is bounded in \( {L^\varphi} \) for \( {\varphi:\mathbb{R}_+\rightarrow\mathbb{R}_+} \) non-decreasing and such that \( {\lim_{x\rightarrow\infty}\varphi(x)=+\infty} \), then the Markov inequality gives
\[ \sup_{i\in I}\mathbb{P}(|X_i|\geq R)\leq \frac{\sup_{i\in I}\mathbb{E}(\varphi(|X_i|))}{\varphi(R)}\underset{R\rightarrow\infty}{\longrightarrow0} \]
and thus the family of distributions \( {{(\mathbb{P}_{X_i})}_{i \in I}} \) is tight, in the sense that for every \( {\varepsilon>0} \), there exists a compact subset \( {K_\varepsilon} \) of \( {\mathbb{R}} \) such that \( {\sup_{i\in I}\mathbb{P}(|X_i|\in K_\varepsilon)\geq 1-\varepsilon} \). The Prohorov theorem states that tightness is equivalent to being relatively compact for the narrow topology (which is by the way metrizable by the bounded-Lipschitz Fortet-Mourier distance). The Prohorov and the Dunford-Pettis theorems correspond to different topologies on different objects (distributions or random variables). The tightness of \( {{(\mathbb{P}_{X_i})}_{i\in I}} \) is strictly weaker than the UI of \( {{(X_i)}_{i\in I}} \).
UI functions with respect to a family of laws. The UI property for a family \( {{(X_i)}_{i\in I}\subset L^1} \) depends actually only on the marginal distributions \( {{(\mathbb{P}_{X_i})}_{i\in I}} \) and does not feel the dependence between the \( {X_i} \)’s. In this spirit, if \( {(\eta_i)_{i\in I}} \) is a family of probability measures on a Borel space \( {(E,\mathcal{E})} \) and if \( {f:E\rightarrow\mathbb{R}} \) is a Borel function then we say that \( {f} \) is UI for \( {(\eta_i)_{i\in I}} \) on \( {E} \) when
\[ \lim_{t\rightarrow\infty}\sup_{i\in I}\int_{\{|f|>t\}}\!|f|\,d\eta_i=0. \]
This means that \( {{(f(X_i))}_{i\in I}} \) is UI, where \( {X_i\sim\eta_i} \) for every \( {i\in I} \). This property is often used in applications as follows: if \( {\eta_n\rightarrow\eta} \) narrowly as \( {n\rightarrow\infty} \) for some probability measures \( {{(\eta_n)}_{n\geq1}} \) and \( {\eta} \) and if \( {f} \) is continuous and UI for \( {(\eta_n)_{n\geq1}} \) then
\[ \int\!|f|\,d\eta<\infty \quad\text{and}\quad \lim_{n\rightarrow\infty}\int\!f\,d\eta_n=\int\!f\,d\eta. \]
Logarithmic potential. What follows is extracted from the survey Around the circular law (random matrices). We have already devoted a previous post to the logarithmic potential. Let \( {\mathcal{P}(\mathbb{C})} \) be the set of probability measures on \( {\mathbb{C}} \) which integrate \( {\log\left|\cdot\right|} \) in a neighborhood of infinity. The logarithmic potential \( {U_\mu} \) of \( {\mu\in\mathcal{P}(\mathbb{C})} \) is the function \( {U_\mu:\mathbb{C}\rightarrow(-\infty,+\infty]} \) defined for all \( {z\in\mathbb{C}} \) by
\[ U_\mu(z)=-\int_{\mathbb{C}}\!\log|z-w|\,d\mu(w) =-(\log\left|\cdot\right|*\mu)(z). \]
Let \( {\mathcal{D}'(\mathbb{C})} \) be the set of Schwartz-Sobolev distributions on \( {\mathbb{C}} \). We have \( {\mathcal{P}(\mathbb{C})\subset\mathcal{D}'(\mathbb{C})} \). Since \( {\log\left|\cdot\right|} \) is Lebesgue locally integrable on \( {\mathbb{C}} \), the Fubini-Tonelli theorem implies that \( {U_\mu} \) is a Lebesgue locally integrable function on \( {\mathbb{C}} \). In particular, we have \( {U_\mu<\infty} \) almost everywhere and \( {U_\mu\in\mathcal{D}'(\mathbb{C})} \). By using Green’s or Stockes’ theorems, one may show, for instance via the Cauchy-Pompeiu formula, that for any smooth and compactly supported function \( {\varphi:\mathbb{C}\rightarrow\mathbb{R}} \),
\[ -\int_{\mathbb{C}}\!\Delta\varphi(z)\log|z|\,dxdy=2\pi\varphi(0) \]
where \( {z=x+iy} \). Now can be written, in \( {\mathcal{D}'(\mathbb{C})} \),
\[ \Delta\log\left|\cdot\right| = 2\pi\delta_0 \]
In other words, \( {\frac{1}{2\pi}\log\left|\cdot\right|} \) is the fundamental solution of the Laplace equation on \( {\mathbb{R}^2} \). Note that \( {\log\left|\cdot\right|} \) is harmonic on \( {\mathbb{C}\setminus\{0\}} \). It follows that in \( {\mathcal{D}'(\mathbb{C})} \),
\[ \Delta U_\mu=-2\pi\mu, \]
i.e. for every smooth and compactly supported “test function” \( {\varphi:\mathbb{C}\rightarrow\mathbb{R}} \),
\[ \langle\Delta U_\mu,\varphi\rangle_{\mathcal{D}’} =-\!\int_{\mathbb{C}}\!\Delta\varphi(z)U_\mu(z)\,dxdy =-2\pi\int_{\mathbb{C}}\!\varphi(z)\,d\mu(z) =-\langle2\pi\mu,\varphi\rangle_{\mathcal{D}’} \]
where \( {z=x+iy} \). Also \( {-\frac{1}{2\pi}U_\cdot} \) is the Green operator on \( {\mathbb{R}^2} \) (Laplacian inverse). For every \( {\mu,\nu\in\mathcal{P}(\mathbb{C})} \), we have
\[ U_\mu=U_\nu\text{ almost everywhere }\Rightarrow \mu=\nu. \]
To see it, since \( {U_\mu=U_\nu} \) in \( {\mathcal{D}'(\mathbb{C})} \), we get \( {\Delta U_\mu=\Delta U_\nu} \) in \( {\mathcal{D}'(\mathbb{C})} \), and thus \( {\mu=\nu} \) in \( {\mathcal{D}'(\mathbb{C})} \), and finally \( {\mu=\nu} \) as measures since \( {\mu} \) and \( {\nu} \) are Radon measures. (Note that this remains valid if \( {U_\mu=U_\nu+h} \) for some harmonic \( {h\in\mathcal{D}'(\mathbb{C})} \)). As for the Fourier transform, the pointwise convergence of logarithmic potentials along a sequence of probability measures implies the narrow convergence of the sequence to a probability measure. We need however some strong tightness. More precisely, if \( {{(\mu_n)}_{n\geq1}} \) is a sequence in \( {\mathcal{P}(\mathbb{C})} \) and if \( {U:\mathbb{C}\rightarrow(-\infty,+\infty]} \) is such that
- (i) for a.a. \( {z\in\mathbb{C}} \), \( { \lim_{n\rightarrow\infty}U_{\mu_n}(z)=U(z)} \);
- (ii) \( {\log(1+\left|\cdot\right|)} \) is UI for \( {(\mu_n)_{n \geq 1}} \);
then there exists \( {\mu \in \mathcal{P}(\mathbb{C})} \) such that
- (j) \( {U_\mu=U} \) almost everywhere;
- (jj) \( {\mu = -\frac{1}{2\pi}\Delta U} \) in \( {\mathcal{D}'(\mathbb{C})} \);
- (jjj) \( {\mu_n\rightarrow\mu} \) narrowly.
Let us give a proof inspired from an article by Goldsheid and Khoruzhenko on random tridiagonal matrices. From the de la Vallée Poussin criterion, assumption (ii) implies that for every real number \( {r\geq1} \), there exists \( {\varphi\in\Phi} \), which may depend on \( {r} \), which is moreover convex and has moderate growth \( {\varphi(x)\leq 1+x^2} \), and
\[ \sup_n \int\!\varphi(\log(r+|w|))\,d\mu_{n}(w)<\infty. \]
Let \( {K\subset \mathbb{C}} \) be an arbitrary compact set. Take \( {r = r(K) \geq 1} \) large enough so that the ball of radius \( {r-1 } \) contains \( {K} \). Therefore for every \( {z\in K} \) and \( {w\in\mathbb{C}} \),
\[ \varphi(|\log|z-w||) \leq (1 + |\log|z-w||^2)\mathbf{1}_{\{|w|\leq r\}} +\varphi(\log(r+|w|))\mathbf{1}_{\{|w|>r\}}. \]
The couple of inequalities above, together with the local Lebesgue integrability of \( {(\log\left|\cdot\right|)^2} \) on \( {\mathbb{C}} \), imply, by using Jensen and Fubini-Tonelli theorems,
\[ \sup_n\int_K\!\varphi(|U_n(z)|)\,dxdy \leq \sup_n\iint\!\mathbf{1}_K(z)\varphi(|\log|z-w||)\,d\mu_n(w)\,dxdy<\infty, \]
where \( {z=x+iy} \) as usual. Since the de la Vallée Poussin criterion is necessary and sufficient for UI, this means that the sequence \( {{(U_{\mu_n})}_{n\geq1}} \) is locally Lebesgue UI. Consequently, from (i) it follows that \( {U} \) is locally Lebesgue integrable and that \( {U_{\mu_n}\rightarrow U} \) in \( {\mathcal{D}'(\mathbb{C})} \). Since the differential operator \( {\Delta} \) is continuous in \( {\mathcal{D}'(\mathbb{C})} \), we find that \( {\Delta U_{\mu_n}\rightarrow\Delta U} \) in \( {\mathcal{D}'(\mathbb{C})} \). Since \( {\Delta U\leq0} \), it follows that \( {\mu:=-\frac{1}{2\pi}\Delta U} \) is a measure (see e.g. Hormander). Since for a sequence of measures, convergence in \( {\mathcal{D}'(\mathbb{C})} \) implies narrow convergence, we get \( {\mu_n=-\frac{1}{2\pi}\Delta U_{\mu_n}\rightarrow\mu=-\frac{1}{2\pi}\Delta U} \) narrowly, which is (jj) and (jjj). Moreover, by assumptions (ii) we get additionally that \( {\mu\in\mathcal{P}(\mathbb{C})} \). It remains to show that \( {U_\mu=U} \) almost everywhere Indeed, for any smooth and compactly supported \( {\varphi:\mathbb{C}\rightarrow\mathbb{R}} \), since the function \( {\log\left|\cdot\right|} \) is locally Lebesgue integrable, the Fubini-Tonelli theorem gives
\[ \int\!\varphi(z)U_{\mu_n}(z)\,dz =-\int\!\left(\int\!\varphi(z)\log|z-w|\,dz\right)\,d\mu_n(w). \]
Now \( {\varphi*\log\left|\cdot\right|:w\in\mathbb{C}\mapsto\int\!\varphi(z)\log|z-w|\,dz} \) is continuous and is \( {\mathcal{O}(\log|1+\cdot|)} \). Therefore, by (i-ii), \( {U_{\mu_n}\rightarrow U_\mu} \) in \( {\mathcal{D}'(\mathbb{C})} \), thus \( {U_\mu=U} \) in \( {\mathcal{D}'(\mathbb{C})} \) and then almost everywhere, giving (j).
Bonjour,
In the proof of 2==>1 , you argued that” …. if \Omega = B_1 …. \cup B_n is a cover, then ….. ” But in general does such a cover exists ?
Sincerely,
Luc.
you are right, something was missing: either one has to ask for the epsilon-delta for any probability space realizing (X_i), or one has to add the boundedness in L^1 to the epsilon-delta criterion. This is fixed now. Thanks.
Bonjour,
C’est moi encore.
In the proof of 1 => 3, you select a strictly increasng sequecne $\{ x_m \}$, then construct $u_n$ and claimed that $\varphi\in\Phi$, since $u_n$ increases to infinity. I think here we need to clarify further about the choice of $x_m$. For example, the following construction fails:
for m, choose $x_m$, an integer greater than $2^m$ such that $\sup_{i\in I} \mathbb{E}\Big[ \vert X_i \vert \cdot 1_{(\vert X_i \vert \geq x_m)} < 2^{-m}$,
then pick another integer $x_{m+1} \geq (x_m + 1)\vee 2^{m+1}$, then
$u_{2^n} \leq n$ so that
$$\lim_{n\to+\infty} \frac{ u_1 + u_2 + \ldots + u_{2^n} }{2^n} = 0 $$
and thus
$$ \frac{\varphi(x)}{x} \xrightarrow{x\to+\infty} 0 \,\, . $$
sorry, the counterexample is wrong!@Luc
To be more explicit, since $\lim_{n\to\infty}u_n=+\infty$, we know that for every $A>0$ there exists $N=N_A$ such that $u_n\geq A$ for all $n\geq N$. Now, by definition of $\varphi$ we have $\varphi'(x)\geq A$ for all $x\geq N$, which gives $\varphi(x)/x\geq (1/x)\int_N^x\!\varphi'(t)\,dt\geq (1-N/x)A$ for any $x\geq N$. In particular $\varphi(x)/x\geq A/2$ for any $x\geq 2N$, and therefore $\lim_{x\to\infty}\varphi(x)/x=+\infty$.
1.
sorry again , the arithmetic mean $\dfrac{1}{n+1}\sum_{k=1}^n u_k \xrightarrow{n\to+\infty} +\infty $. Here is an elementary proof:
For any $M > 0$, there exists $N_1\in\mathbb{N}^\ast$ such that $u_n > M $ as $n\geq N_1$
and there exists $N_2\in\mathbb{N}^\ast$ such that $N_2 > N_1$ and $u_n > 2M$, as $n \geq N_2$,
then for $n > N_1 + N_2 + 1$, we have
\begin{align*}
\frac{u_1 + u_2 + \ldots + u_n }{n+1} &= \frac{u_1 + u_2 + \ldots + u_{N_1} }{n+1} + \frac{u_{N_1 + 1} + \ldots + u_{N_2} }{n+1} + \frac{u_{N_2 + 1} + \ldots + u_n }{n+1} \\
& \geq \frac{u_{N_1 + 1} + \ldots + u_{N_2} }{n+1} + \frac{u_{N_2 + 1} + \ldots + u_n }{n+1} \\
& \geq \frac{(N_2 – N_1)\times M}{n+1} + \frac{(n – N_2)\times (2M)}{n+1} \\
& = 2M – \frac{2 + N_2 + N_1}{n+1}\cdot M > M \,\, .
\end{align*}
This implies $\dfrac{\varphi(n+1)}{n+1} \xrightarrow{n\to+\infty} +\infty$ hence $\dfrac{\varphi(x)}{x} \geq \dfrac{\varphi( \lfloor x \rfloor)}{ \lfloor x \rfloor } \cdot \dfrac{ x- 1 }{x} \xrightarrow{x\to+\infty} +\infty$.
2.
In the proof of $\Big( 1\Longrightarrow 3 \Big)$ , the very last equality should be changed to the inequality $\leq$ ? see the following ?
\begin{align*}
&\ldots\ldots = \sum_{m\geq 1} \sum_{n\geq x_m} \mathbb{P} \big( \vert X_i \vert \geq n \big) \\
& = \sum_{m=1}^\infty \left[ \,\, \sum_{n \geq x_m} \sum_{k=n}^\infty \mathbb{P} \big( k \leq \vert X_i \vert < k+1 \big) \,\, \right] \\
& = \sum_{m=1}^\infty \left[ \,\, \sum_{k \geq x_m} \sum_{n = x_m}^k \mathbb{P} \big( k \leq \vert X_i \vert < k+1 \big) \,\, \right] \\
& = \sum_{m=1}^\infty \sum_{k \geq x_m} (k – x_m) \mathbb{P} \big( k \leq \vert X_i \vert < k+1 \big) \\
& \leq \sum_{m=1}^\infty \sum_{k \geq x_m} k \cdot \mathbb{P} \big( k \leq \vert X_i \vert < k+1 \big)
\end{align*}
1. All right. 2. Youare right, and this glitch is now fixed, thanks.
For French readers, the concept of uniform integrability, under the name équi-intégrabilité, is considered in the book « Bases mathématiques du calcul des probabilités » by Jacques Neveu. In particular the de la Vallée Poussin criterion is considered, sligltly and without the name and the equivalence, in Exercice II-5-2 page 52. This precise reference was communicated by Laurent Miclo. For a more substantial treatment, the best remains the book by Claude Delacherie and Paul-André Meyer.
Bonjour,
It is not true that the span of a family of UI is UI, the span of 1 (i.e. R) is not UI. Is it correct?
Merci
Thank you very much, you are perfectly right. It does not work with the span. Actually it is a matter of tightness, because it works nicely with the convex envelope. I have updated the post accordingly.