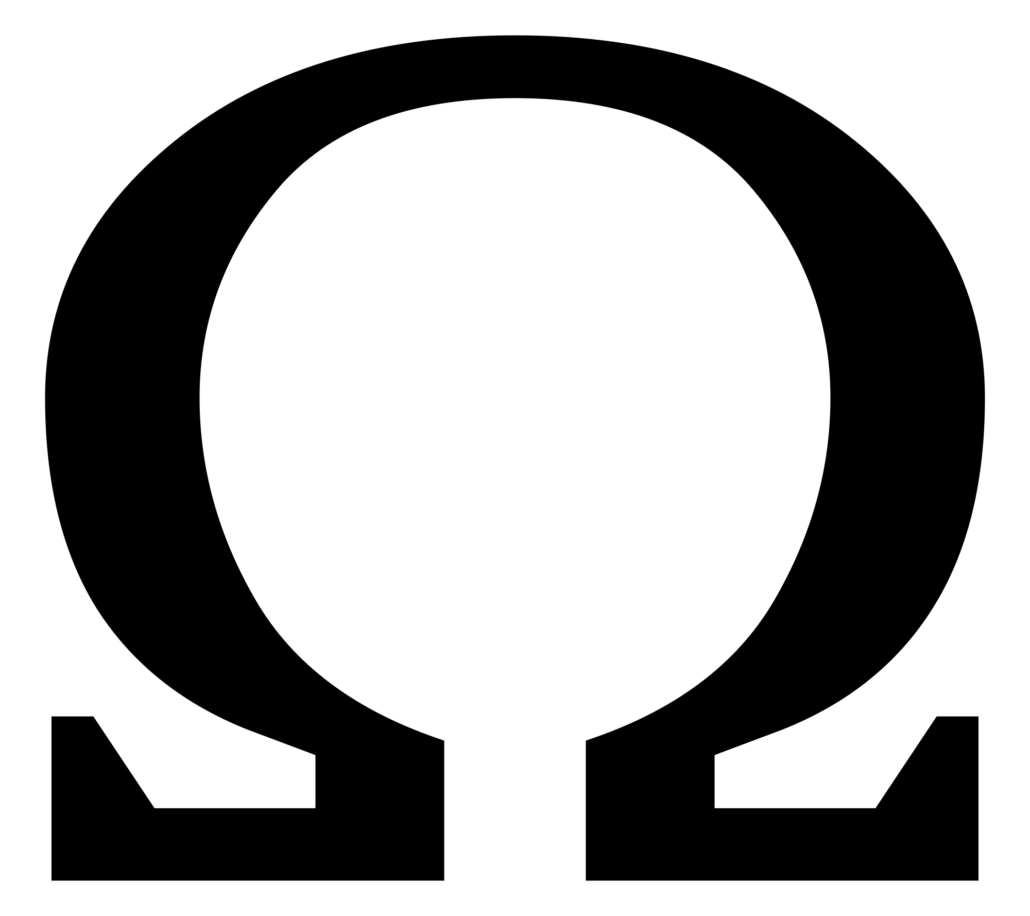
This post is devoted to a quick proof of a version of the law of large numbers for non-negative random variables in $\mathrm{L}^2$. It does not require independence or same distribution. It is a variant of the famous one for independent random variables bounded in $\mathrm{L}^4$.
The statement. If $X_1,X_2,\ldots$ are non-negative $\mathrm{L}^2$ random variables on $(\Omega,\mathcal{A},\mathbb{P})$ such that $\lim_{n\to\infty}\frac{1}{n}\mathbb{E}(S_n)=\ell\in\mathbb{R}$, and $\mathrm{Var}(S_n)=\mathcal{O}(n)$ where $S_n:=X_1+\cdots+X_n$, then $$\lim_{n\to\infty}\frac{S_n}{n}=\ell\text{ almost surely}.$$
A proof. We have $$\mathbb{E}\Bigr(\sum_n\Bigr(\frac{S_{n^2}-\mathbb{E}(S_{n^2})}{n^2}\Bigr)^2\Bigr)=\sum_n\frac{\mathrm{Var}(S_{n^2})}{n^4}=\mathcal{O}\Bigr(\sum_n\frac{1}{n^2}\Bigr)<\infty.$$ It follows that $\sum_n\Bigr(\frac{S_{n^2}-\mathbb{E}(S_{n^2})}{n^2}\Bigr)^2<\infty$ almost surely, which implies that $\frac{S_{n^2}-\mathbb{E}(S_{n^2})}{n^2}\to0$ almost surely. This gives that $\frac{1}{n^2}S_{n^2}\to\ell$ almost surely. It remains to use the sandwich$$\frac{S_{n^2}}{n^2}\frac{n^2}{(n+1)^2}\leq\frac{S_k}{k}\leq\frac{S_{(n+1)^2}}{(n+1)^2}\frac{(n+1)^2}{n^2}$$ valid if $k$ is such that $n^2\leq k\leq (n+1)^2$ (here we use the non-negative nature of the $X$'s).
Note and further reading. I have learnt this proof recently from my friend Arnaud Guyader who found it in a paper (Theorem 5) by Bernard Delyon and François Portier. It is probably a classic however. It is possible to drop the assumption of being non-negative by using $X=X_+-X_-$, but this would require to modify the remaining assumptions, increasing the complexity, see for instance Theorem 6.2 in Chapter 3 of Erhan Çinlar book (and the blog post comments below). The proof above has the advantage of being quick and beautiful.
Yes this type of subsequence argument can be found in e.g. Theorem 6.2 of E. Cinlar "Probability and Stochastics"
https://www.springer.com/us/book/9780387878584
Therein it was pointed out that for iid sequence one can apply the argument to X_i^+ and X_i^- separately so positivity can be assumed without loss of generality.
Thanks for the $X_{\pm}$, I have just updated the post accordingly. However the proof in this book is less elegant.
Then you need something more if you do not suppose nonnegativity, right ? iid ?
Because the assumptions on $S_{n,+}$ and $Var(S_{n,+}$ may not be satisfied…
Yes, and in fact I prefer the initial version. So I reverted the post to its initial version!
I did not see any essential difference. The "Borel-Cantelli" part is the same. Lemma 1.7 in the book is the sandwich bound of the ratios.
The difference is essentially in aesthetics and kind of generality, I would say. Nevertheless, I have just added precise references to better suit readers sharing your way of thinking.
To complement yihong's answer, it's Theorem 6.2 of *Chapter III* of Çinlar's book (the theorems numbers are reset at eat chapter).
I don't understand the Sandwich take X_1 X_2 .. to be deterministic
2, 2, 2, 2, -3, -4, 0, 0, 1
and case n=2, S_4/9=8/9 > S_6/6=1/6.
Maybe you mean X_1 X_2 .. positive, but then the theorem is less interesting.
Yes, thanks, I've fixed the post. Still it remains remarkably beautiful.