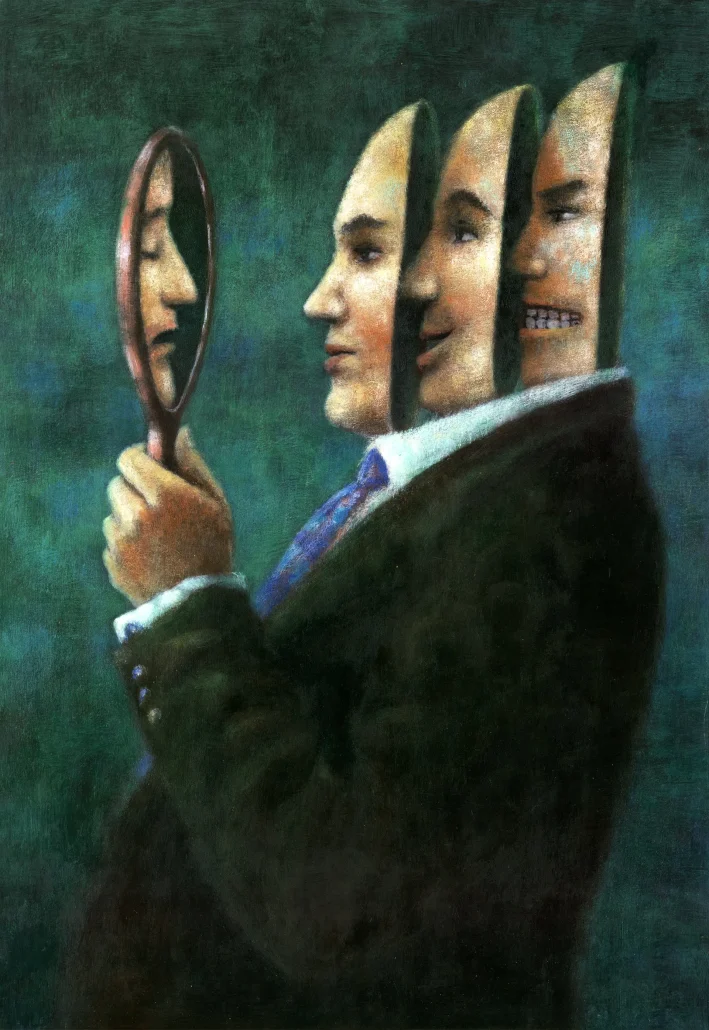
En France comme dans bien d’autres pays développés, les temples de l’élitisme sont avant tout ceux de l’auto-reproduction de dominants socio-culturels. Ceci explique peut-être l’effacement relatif de la question sociale dans certains de ces établissements, au profit de questions sociétales qui préoccupent les dominants et leur progéniture. Il faut dire que ces bourgeois, petits ou grands, bohèmes ou pas, d’extrême gauche ou pas, font des enfants, mais pas des enfants d’ouvriers, et sont pratiquement les seuls à pouvoir optimiser le parcours scolaire.
L’establishment, travaillé par ses propres convictions et une certaine militance parmi ses gouvernés, peut même aller jusqu’à tenter d’imposer à tous un point de vue dogmatique bien-pensant sur des sujets de société ou d’actualité. C’est qu’il faut faire en sorte que tout le monde réfléchisse correctement, éclairer les déviants, intimider les dissidents. Totalitarisme d’opérette ? Maccarthysme, inquisition, chasse aux sorcières qui ne disent pas leur nom ? Ces termes sont excessifs ? Nombreux sont ceux qui haussent les épaules, courbent l’échine, préfèrent se taire et attendre des jours meilleurs. Après tout, staliniens et autres maoïstes n’ont fait que passer.
L’Histoire suggère que la vérité tient plus d’une quête permanente que d’un aboutissement définitif. Il va sans dire que toutes les certitudes sont revisitées ou revisitables, mais toutes ne sont pas à mettre sur le même plan, certaines sont plus étayées que d’autres. Et il ne suffit pas de se nourrir de déconstruction ou de confiance pour avoir raison. La subversion et la nouveauté, pas plus que le conformisme et la tradition, ne garantissent justesse et pertinence, même s’ils exercent un fort pouvoir de séduction sur les esprits. Curieusement, les dominants socio-culturels, héritiers et pratiquants de la liberté de pensée et d’expression, sont parfois les premiers à vouloir la contrôler, au nom d’une orthodoxie morale de nature religieuse, convaincus de détenir la vérité, et d’avoir le devoir de faire taire ceux qui pensent différemment. L’Histoire nous enseigne qu’un totalitarisme peut se bâtir sur une absence de doute et d’esprit critique parmi les puissants, une médiocrité intellectuelle vécue comme juste, visionnaire, et à l’avant-garde.
1 Comment