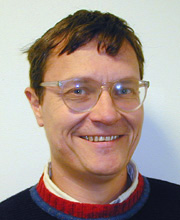
Laurent Saloff-Coste was one of my professors during my second year of Master. His teaching style was truly original, not at all academic, difficult to reproduce. I greatly appreciated his course on random walks on groups, between probability and algebra, involving analysis thru Sobolev inequalities, duality, and interpolation, and geometry thru isoperimetry and volume growth.
This post is about the cutoff phenomenon for ergodic Markov processes, more precisely the analysis conducted by Laurent Saloff-Coste and his student Guan-Yu Chen in 2008 for \( {\max L^p} \) cutoff, \( {1<p<\infty} \) under the product condition put forward by Yuval Peres. This phenomenon is about the abrupt convergence to the equilibrium. The cutoff phenomenon was put forward by David Aldous and Persi Diaconis for the study of random walks on groups.
Setting. Let \( {X={(X_t)}_{t\geq0}} \) be a continuous time Markov process with state space \( {S} \). For all \( {t\geq0} \), all bounded measurable \( {f:S\rightarrow\mathbb{R}} \), and all \( {x\in S} \), we set
\[ P_t(f)(x):=\mathbb{E}(f(X_t)\mid X_0=x). \]
The family \( {{(P_t)}_{t\geq0}} \) is a Markov semigroup of linear operators on bounded measurable functions : \( {P_0=\mathrm{id}} \), \( {P_s\circ P_t=P_{t+s}} \) (Markov property), \( {P_t(1)=1} \), \( {P_t(f)\geq0} \) for \( {f\geq0} \), \( {s,t\geq0} \). It acts on probability measures (left) and on bounded measurable functions (right) :
\[ \mu(P_tf) =(\mu P_t)(f) =\mu P_tf=\int P_t(f)(x)\mathrm{d}\mu(x). \]
For all \( {t\geq0} \), we have \( {X_t\sim\mu_t} \) where
\[ \mu_t:=\mu_0P_t. \]
In particular if \( {\mu_0=\delta_x} \) then \( {\mu_t=P_t(\cdot)(x)} \). We suppose that there exists a unique invariant probability measure \( {\pi} \) : if \( {\mu_0=\pi} \) then \( {\mu_t=\pi} \) for all \( {t\geq0} \).
Contraction. We denote by \( {\left\|\cdot\right\|_p} \) the \( {L^p(\pi)} \) norm, \( {p\in[1,\infty]} \). For all \( {t\geq0} \) and \( {f\in L^p} \), since \( {P_t} \) is an expectation, the function \( {P_t(|f|)} \) is well defined, and by the Jensen inequality \( {\|P_t(|f|)\|_p\leq\|f\|_p} \). Hence the function \( {P_t(f)} \) is well defined, is in \( {L^p(\pi)} \), and \( {P_t} \) is a linear contraction of \( {L^p(\pi)} \). In terms of operator norm, and recalling that \( {P_t(1)=1} \),
\[ \|P_t\|_{p\rightarrow p}=1. \]
The \( {L^p} \) distance to equilibrium and duality. For all \( {p\in[1,\infty]} \) and \( {t\geq0} \), we set
\[ \|\mu_t-\pi\|_p :=\begin{cases} \|h_t-1\|_p & \text{if }\mu_t\ll\pi\text{ with }\mathrm{d}\mu_t=h_t\mathrm{d}\pi\\ \infty & \text{if }\mu_t\not\ll\pi\text{ and }p>1\\ 2 & \text{if }\mu_t\not\ll\pi\text{ and }p=1 \end{cases}. \]
The \( {L^p-L^q} \) duality gives
\[ \|h_t-1\|_p =\sup_{\substack{g\in L^q(\pi)\\\|g\|_q=1}} \int g(h_t-1)\mathrm{d}\pi =\sup_{\substack{g\in L^q(\pi)\\\|g\|_q=1}}(\mu_t-\pi)(g) \]
where \( {q:=1/(1-1/p)} \) is the Hölder conjugate of \( {p} \). Hence, if \( {\mu_t\ll\pi} \), then
\[ \|\mu_t-\pi\|_p =\sup_{\substack{g\in L^q(\pi)\\\|g\|_q=1}}(\mu_t-\pi)(g). \]
The cases \( {p\in\{1,2\}} \) correspond to chi-square divergence and total variation distance :
\[ \begin{array}{rcl} \|h_t-1\|_2^2 &=&\int(h_t^2-2h_t+1)\mathrm{d}\pi =\|h_t\|_2^2-1 =\mathrm{Var}_\pi(h_t)=:\chi^2(\mu_t\mid\pi)\\ \|h_t-1\|_1 &=&\sup_{\substack{f\in L^\infty(\pi)\\\|f\|_\infty\leq1}}\int(h_t-1)f\mathrm{d}\pi =2\sup_A|\mu_t(A)-\pi(A)|=:2\|\mu_t-\pi\|_{\mathrm{TV}}. \end{array} \]
Monotonicity. For all \( {E\subset\mathcal{P}(S)} \), the following function is non-increasing :
\[ t\geq0\mapsto\sup_{\mu_0\in E}\|\mu_t-\pi\|_p. \]
Indeed, is suffices to consider the case when \( {E} \) is a singleton, the general case follows by taking the sup. Now, by invarince, for all \( {s,t\geq0} \), \( {p\in[1,\infty]} \), with \( {q:=1/(1-1/p)} \),
\[ (\mu_{t+s}-\pi)(f)=(\mu_t-\pi)P_s(f). \]
Hence if \( {\mu_t\ll\pi} \) with \( {h_t:=\frac{\mathrm{d}\mu_t}{\mathrm{d}\pi}\in L^p(\pi)} \), then the Hölder inequality and the contraction property of \( {P_t} \) in \( {L^q(\pi)} \) give
\[ |(\mu_{t+s}-\pi)(f)| \leq\|h_t-1\|_p\|P_t(f)\|_q \leq\|h_t-1\|_p\|f\|_q. \]
Therefore \( {\mu_{t+s}\ll\pi} \) with \( {h_{t+s}\in L^p(\pi)} \) and \( {\|h_{t+s}-1\|_p\leq \|h_t-1\|_p} \) in other words
\[ \|\mu_{t+s}-\pi\|_p\leq\|\mu_t-\pi\|_p. \]
Mixing time. For any \( {E\subset\mathcal{P}(S)} \), \( {p\in[1,\infty]} \), and threshold \( {\eta>0} \), it is defined as
\[ T_{\mathrm{mix}}:= T_{\mathrm{mix}}(E,p,\eta) := \inf\Bigr\{t>0:\sup_{\mu_0\in E}\|\mu_t-\pi\|_p\leq\eta\Bigr\}. \]
In particular
\[ \sup_{\mu_0\in E}\|\mu_t-\pi\|_p \begin{cases} >\eta & \text{if }t<T_{\mathrm{mix}}\\ \leq\eta & \text{if }t\geq T_{\mathrm{mix}} \end{cases}. \]
Spectral gap. It is the largest constant \( {\lambda\geq0} \) such that for all \( {t\geq0} \) and \( {f\in L^2(\pi)} \),
\[ \|P_t(f)-\pi(f)\|_2\leq\mathrm{e}^{-\lambda t}\|f\|_2 \quad\text{where}\quad \pi(f):=\int f\mathrm{d}\pi. \]
A positive spectral gap \( {\lambda>0} \) means that the process is ergodic : \( {\mu_t\rightarrow\pi} \) as \( {t\rightarrow\infty} \), actually exponentially ergodic in \( {L^2} \). For any threshold \( {\eta\in(0,1)} \), the relaxation time is
\[ T_{\mathrm{rel}} :=T_{\mathrm{rel}}(\eta) :=\frac{-\log(\eta)}{\lambda}. \]
In terms of operator norm : \( {\|P_t-\pi\|_{2\rightarrow2}\leq\mathrm{e}^{-\lambda t}} \). Since \( {\|P_t-\pi\|_{p\rightarrow p}\leq2} \) for \( {p\in\{1,\infty\}} \), the Riesz-Thorin interpolation theorem yields, for all \( {p\in(1,\infty)} \) and \( {t\geq0} \),
\[ \|P_t-\pi\|_{p\rightarrow p}\leq C_p\mathrm{e}^{-\lambda c_pt} \]
where \( {C_p:=2^{|1-2/p|}} \) and \( {c_p:=1-|1-2/p|>0} \). In a sense \( {c_p=0} \) if \( {p\in\{1,\infty\}} \).
Cutoff in \( {L^p} \), \( {1<p<\infty} \). Suppose that the process \( {X} \) depends on an integer parameter \( {n} \). In particular the state space \( {S} \), the set of initial distributions \( {E\subset\mathcal{P}(S)} \), the invariant probability measure \( {\pi} \), the spectral gap \( {\lambda} \), the relaxation time \( {T_{\mathrm{rel}}} \), and the mixing time \( {T_{\mathrm{mix}}} \) depend on \( {n} \). The parameter \( {n} \) encodes typically a dimension, or size. Basic examples are given by Brownian motion on the sphere, and by the random transposition walk on the symmetric group. In other words, we consider a sequence of processes indexed by \( {n} \). We fix the parameter \( {\eta} \) in the definition of \( {T_{\mathrm{rel}}} \) and \( {T_{\mathrm{mix}}} \) to some value that does not depend on \( {n} \). We display the dependence on \( {n} \) with a subscript or superscript. Now, if the product condition is satisfied :
\[ \lim_{n\rightarrow\infty}\lambda_nT_{\mathrm{mix}}^{(n)}=\infty \quad\text{in other words}\quad T_{\mathrm{rel}}^{(n)}\underset{n\rightarrow\infty}{\ll} T_{\mathrm{mix}}^{(n)} \]
then we have a cutoff phenomenon at the mixing time : for all \( {\varepsilon\in(0,1)} \) and \( {t\geq0} \),
\[ \lim_{n\rightarrow\infty}\sup_{\mu_0^{(n)}\in E_n}\|\mu_t^{(n)}-\pi_n\|_p =\begin{cases} 0 & \text{if }t<(1-\varepsilon)T_{\mathrm{mix}}^{(n)}\\ \infty & \text{if }t>(1+\varepsilon)T_{\mathrm{mix}}^{(n)} \end{cases}. \]
The condition \( {\lim_{n\rightarrow\infty}\lambda_nT_{\mathrm{mix}}^{(n)}=\infty} \) implies in particular that \( {T_{\mathrm{mix}}^{(n)}>0} \) for \( {n} \) large enough.
Proof. We drop the \( {n} \) sub/superscripts for simplicity. For all \( {u,v\geq0} \), all \( {f\in L^q(\pi)} \), \( {q=1/(1-1/p)} \), \( {p\in(1,\infty)} \), the semigroup property for \( {P} \) and the invariance of \( {\pi} \) give
\[ (\mu_{u+v}-\pi)(f) =(\mu_uP_v-\pi P_v)(f) =(\mu_u-\pi)(P_v-\pi)(f). \]
Therefore
\[ |(\mu_{u+v}-\pi)(f)| \leq\|\mu_u-\pi\|_p\|(P_v-\pi)(f)\|_q \leq\|\mu_u-\pi\|_pC_p\mathrm{e}^{-\lambda c_p v}\|f\|_q, \]
and taking the supremum over \( {f\in L^q(\pi)} \) such that \( {\|f\|_q=1} \) yields \begin{align*} \|\mu_{u+v}-\pi\|_p \leq \|\mu_u-\pi\|_pC_p\mathrm{e}^{-\lambda c_p v}, \end{align*} in other words, the function \( {d(t):=\|\mu_t-\pi\|_p} \) satisfies
\[ d(u+v)\leq d(u)C_p\mathrm{e}^{-\lambda c_p v}, \]
which gives, with \( {d_E(t):=\sup_{\mu_0\in E}d(t)} \),
\[ d_E(u+v)\leq d_E(u)C_p\mathrm{e}^{-\lambda c_p v}. \]
Now, with \( {u=T_{\mathrm{mix}}} \), \( {v=\varepsilon T_{\mathrm{mix}}} \), \( {\varepsilon\in(0,1)} \), we get, by monotonicity,
\[ \sup_{t>(1+\varepsilon)T_{\mathrm{mix}}}d(t)_E\leq d_E(u+v) \leq\eta C_p\mathrm{e}^{-\lambda T_{\mathrm{mix}} c_p\varepsilon} \underset{n\rightarrow\infty}{\longrightarrow}0, \]
while with \( {u=(1-\varepsilon)T_{\mathrm{mix}}} \), \( {v=\frac{\varepsilon}{2}T_{\mathrm{mix}}} \), \( {\varepsilon\in(0,1)} \), we get, by monotonicity,
\[ \inf_{t<(1-\varepsilon)T_{\mathrm{mix}}}d_E(t)\geq d_E(u) \geq d_E((1-\tfrac{\varepsilon}{2})T_{\mathrm{mix}}) C_p^{-1}\mathrm{e}^{\lambda T_{\mathrm{mix}}c_p\frac{\varepsilon}{2}} \geq \eta C_p^{-1}\mathrm{e}^{\lambda T_{\mathrm{mix}}c_p\frac{\varepsilon}{2}} \underset{n\rightarrow\infty}{\longrightarrow}+\infty. \]
For both the upper bound and the lower bound, we have used the assumption \( {\lim_{n\rightarrow\infty}\lambda T_{\mathrm{mix}}=\infty} \) (product condition on spectral gap and mixing time), as well as the fact that \( {c_p>0} \), which is the case if \( {p\in(1,\infty)} \), from Riesz-Thorin, while \( {c_p=0} \) if \( {p\in\{1,\infty\}} \).
Corollary in the \( {L^2} \) case. When \( {p=2} \), the product condition above is satisfied when \( {E_n=\{\delta_{x_n}\}} \) for some \( {x_n\in S_n} \) and there exists \( {\varphi_n\in L^2(\pi_n)} \) such that
\[ \lim_{n\rightarrow\infty}\frac{|\varphi_n(x_n)|}{\|\varphi_n\|_2}=+\infty \quad\text{and}\quad |(P_t^{(n)}-\pi_n)(\varphi_n)(x_n)| \geq\mathrm{e}^{-c\lambda_n t}|\varphi_n(x_n)| \]
for some constant \( {c>0} \) and for all \( {t\geq0} \) and \( {n} \). Indeed, at \( {t=T_{\mathrm{mix}}} \), by duality,
\[ \eta\geq\|\mu_t^{(n)}-\pi_n\|_2=\sup_{\|g\|_2=1}|(P_t^{(n)}-\pi_n)(g)| \geq\mathrm{e}^{-c\lambda_nT_{\mathrm{mix}}^{(n)}} \frac{|\varphi_n(x_n)|}{\|\varphi_n\|_2} \]
which imposes the product condition \( {\lim_{n\rightarrow\infty}\lambda_nT_{\mathrm{mix}}^{(n)}=+\infty} \). This is particularly appealing when we know an eigenfunction associated to the spectral gap.
Let us check now this \( {L^2} \) criterion or sufficient condition on the explicit Ornstein-Uhlenbeck process on \( {\mathbb{R}^n} \), generated by the differential operator \( {\frac{1}{n}\Delta-\langle x,\nabla\rangle} \). The Mehler formula reads \( {P_t(\cdot)(x)=\mathcal{N}(x\mathrm{e}^{-t},\frac{1-\mathrm{e}^{-2t}}{n}\mathrm{Id}_n)} \), and we have \( {\pi_n=\mathcal{N}(0,\frac{1}{n}\mathrm{Id}_n)} \) and \( {\lambda_n=1} \). The condition above is satisfied by the eigenfunction \( {\varphi_n(x)=\langle x,(1,\ldots,1)\rangle} \) associated to \( {\lambda_n} \), for which \( {\|\varphi_n\|_2=1} \), as soon as \( {\lim_{n\rightarrow\infty}|\langle x_n,(1,\ldots,1)\rangle|=+\infty} \). On the other hand, the explicit Gaussian computation of the \( {L^2} \) norm yields
\[ \|\mu_t^{(n)}-\pi_n\|_2^2 =-1+\frac{1}{(1-\mathrm{e}^{-4t})^{n/2}} \exp\Bigr(n|x_n|^2\frac{\mathrm{e}^{-2t}}{1+\mathrm{e}^{-2t}}\Bigr) \quad\text{thus}\quad T_{\mathrm{mix}}=\log\sqrt{n|x_n|^2}. \]
By the Cauchy-Schwarz inequality, \( {|\langle x,(1,\ldots,1)\rangle|^2\leq n|x_n|^2} \), hence \( {\lim_{n\rightarrow\infty}T_{\mathrm{mix}}=+\infty} \).
More generally, for the Dyson-Ornstein-Uhlenbeck process on \( {\{x\in\mathbb{R}^d:x_1\leq\cdots\leq x_n\}} \) generated by the differential operator
\[ Lf:=\frac{1}{n}\sum_{i=1}^n\partial_i^2-\sum_{i=1}^nx_i\partial_i +\frac{\beta}{2n}\sum_{j\neq i}\frac{\partial_i-\partial_j}{x_i-x_j}, \]
we have \( {\lambda_n=1} \),
\[ \pi_n= \frac{1}{Z_{n,\beta}}\prod_{i=1}^n\mathrm{e}^{-\frac{n}{2}x_i^2} \prod_{i>j}(x_i-x_j)^\beta\mathrm{1}_{x_1\leq\cdots\leq x_n} \mathrm{d}x_1\cdots\mathrm{d}x_n, \]
and for the same \( {\varphi_n} \) as above, we can check by integration by parts that \( {\|\varphi_n\|_2=1} \).
Further comments.
- We do not need that he process is reversible or that the state space is compact.
- The analysis can be easily refined using a notion of cutoff window.
- The conclusion does not hold for \( {p=1} \), even in the reversible case. However, the conclusion holds for \( {p=\infty} \) in the reversible case. An analysis can be conducted in the normal case, which is more general than the self-adjointness of reversibility.
- The product condition is sufficient but not necessary for the cutoff.
- From the \( {L^p} \) point of view, the case \( {p=1} \) (total variation) appears as a degenerate case, and is indeed more delicate and sensitive. The cutoff phenomenon can be considered for various other distances or divergences, such as the separation distance, the Hellinger distance (equivalent to total variation), the Kullback-Leibler relative entropy, the Kantorovich-Wasserstein coupling distance, etc.
- The relaxation time (spectral gap) as well as the mixing time can be related to curvature and dimension via Sobolev type functional inequalities.
Further reading.
- Guan-Yu Chen and Laurent Saloff-Coste
The cutoff phenomenon for ergodic Markov processes
Electronic Journal of Probability 13(3) 26-78 (2008)
Mostly Theorem 3.3 and Corollary 3.4