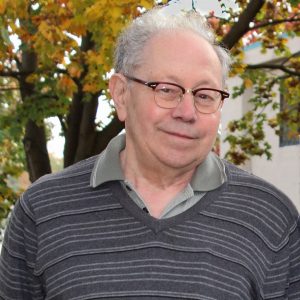
This post is formed with the rough notes that I have prepared for a long informal talk given on January 3, 2023, at Paris-Dauphine, around log-Sobolev inequalities and the Bakry-Émery criterion. Many aspects are already in Master 2 Lecture Notes written with Joseph Lehec.
The logarithmic Sobolev inequality (LSI) concept was forged by Leonard Gross (1931 -) in 1975, as a reformulation of the hypercontractivity of a Markov semigroup. More precisely, if $(P_t)_{t\geq0}=(\mathrm{e}^{tL})_{t\geq}$ is a Markov semigroup with infinitesimal generator $L$ and invariant probability measure $\mu$, and if $L$ is a diffusion or if $\mu$ is reversible, then for all constant $c>0$,
\[
\|P_t(f)\|_{1+(p-1)\mathrm{e}^{4t/c}}\leq\|f\|_p,\quad \forall t\geq0,\forall p\geq1,\forall f,
\] if and only if
\[
\int f^2\log(f^2)\mathrm{d}\mu
\leq -c\int fLf\mathrm{d}\mu+\int f^2\mathrm{d}\mu\log\int f^2\mathrm{d}\mu\,\quad\forall f.
\] The name hypercontractivity comes from the fact that $1+(p-1)\mathrm{e}^{4t/c}>p$ if $t>0$. The name LSI comes from an analogy with classical Sobolev inequalities. The logarithm in the LSI comes from hypercontractivity as a derivative of the $L^p$ norm with respect to $p$.
\[
\partial_p\int |f|^p\mathrm{d}\mu=\int|f|^p\log|f|\mathrm{d}\mu.
\] The assumption of being a diffusion or reversible allows to transform the LSI into a $p$-homogeneous statement by a simple power change of function. The hypercontractivity inequality is an equality at time $t=0$, and the LSI is its infinitesimal version, via $L=\partial_{t=0}P_t$. The same holds for all $t$ due to the Markov nature of the semigroup and the invariance of $\mu$.
Still in the reversible Markovian context, the LSI is also equivalent to the sub-exponential decay in time of relative entropy along the dynamics, namely, with $f_t:=P_t(f)$, $f\geq0$, $\int f\mathrm{d}\mu=1$ :
$$
\int f_t\log(f_t)\mathrm{d}\mu
\leq\mathrm{e}^{-4t/c}\int f_0\log(f_0)\mathrm{d}\mu,\quad\forall f\geq0,\forall t\geq0.
$$ The Boltzmannian H-theorem for the (linear) evolution equation $\partial_tf_t=Lf_t$ reads
$$\partial_t\int f_t\log(f_t)\mathrm{d}\mu=\int\log(f_t)Lf_t\mathrm{d}t\leq0$$ while the sub-exponential decay above is the corresponding Cercignany theorem. In this context, and beyond the monotonicity, the Bakry-Émery criterion provides a convexity in time, as well as the exponential decay via a Grönwall lemma. It is this connection with kinetic theory and the Boltzmann PDE that brought Cédric Villani to the domain in the late 1990s.
We know nowadays that the LSI is linked with information theory, functional analysis, analysis on manifolds, statistical mechanics, harmonic analysis, analysis of PDE, stochastic processes, free probability, high dimensional probability, high dimensional statistics, among other fields. Inspired by Cédric Villani, a historical note by Michel Ledoux gathers 15 proofs of the Gaussian LSI.
Leonard Gross should not be confused with the physicist David J. Gross (1941 — ), the theoretical physicist Eugene P. Gross (1926 — 1991), the mathematicians Benedict Hyman Gross (1950 — ) and Mark Gross (1965 – his son!), among other big Gross.
The Gaussian LSI was already studied, in its Lebesgue form in 1959 by the information theorist Aart Johannes Stam (1929 — 2020), in 1969 by the mathematical physicist Paul Gerard Federbush (1934 — ), an academic grandson of Enrico Fermi, and the academic grandfather of Roland Bauerschmidt, and in 1975 by William G. Faris (1939 — ).
In the 1980s, the LSI and related functional inequalities were also studied by Paul-André Meyer and Dominique Bakry, Michel Émery, as well as Michel Ledoux, Daniel Stroock, Oscar Rothaus, … In the 1990s, came Laurent Miclo, William Beckner, Laurent Saloff-Coste, Persi Diaconis, …, but also, for statistical mechanics, Horng-Tzer Yau, Boguslaw Zegarlinski, Fabio Martinelli, Thierry Bodineau, Bernard Helffer, … In the 2000s, came Sergey Bobkov, Cédric Villani, Liming Wu, Patrick Cattiaux, Arnaud Guillin,…
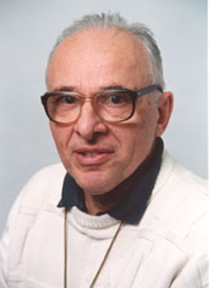
Variance, entropies, Fisher.
\begin{align*}
\mathrm{Var}_{\mu}(f)&=\int f^2\mathrm{d}\mu-\left(\int f\mathrm{d}\mu\right)^2=\mathrm{Var}(f(X))\quad\text{where $X\sim\mu$}\\
\mathrm{Ent}_{\mu} (f)&=\int f \log f\mathrm{d}\mu – \left( \int f\mathrm{d}\mu \right)\log \left( \int f\mathrm{d}\mu \right),\quad f\geq0\\
\mathrm{Ent}_{\mu}^\Phi(f)&=\int\Phi(f)\mathrm{d}\mu-\Phi\Bigr(\int f\mathrm{d}\mu\Bigr) =\mathbb{E}(\Phi(f(X)))-\Phi(\mathbb{E}(f(X))\\
\Phi(u)&=u^2, u\in\mathbb{R}\\
\Phi(u)&=u\log(u), u\geq0
\end{align*} To make it $2$-homogeneous like the variance : $\mathrm{Ent}_{\mu}(f^2)$
Kullback-Leibler divergence (relative entropy) : $\mathrm{H}(\nu\mid\mu)\geq0$, $\mathrm{d}\nu:=f\mathrm{d}\mu$
On $\mathbb{R}^n$ relation to statistical physics/mechanics :
- Boltzmann (or Shannon) entropy and Boltzmann-Gibbs probability measure
\[
\mathrm{S}(\nu):=-\int\frac{\mathrm{d}\nu}{\mathrm{d}x}\log\frac{\mathrm{d}\nu}{\mathrm{d}x}\mathrm{d}x
\quad\text{and}\quad
\mu_\beta:=\frac{1}{Z_\beta}\mathrm{e}^{-\beta
V}\mathrm{d}x
\] - Maximum entropy at fixed average energy
\[
\mathrm{S}(\mu_\beta)-\mathrm{S}(\nu)=\mathrm{H}(\nu\mid\mu_\beta)\geq0 \quad\text{if}\quad \int V\mathrm{d}\mu_\beta=\int V\mathrm{d}\nu
\] - Minimum Helmholtz free energy via penalization
\begin{align*}
\mathrm{F}(\nu)&:=\int V\mathrm{d}\nu-\frac{1}{\beta}\mathrm{S}(\nu)\\
\mathrm{F}(\nu)-\mathrm{F}(\mu_\beta) &= \frac{1}{\beta}\mathrm{H}(\nu\mid\mu_\beta)\geq0\\
\mathrm{F}(\mu_\beta)&=-\frac{1}{\beta}\log Z_\beta.
\end{align*} - Fisher information (statistics, information theory) $\mathrm{d}\nu=f\mathrm{d}\mu$,
\[
\mathrm{I}(\nu\mid\mu)
=\int\frac{|\nabla f|^2}{f}\mathrm{d}\mu
=\int|\nabla\log f|^2\mathrm{d}\nu.
\]
Poincaré and log-Sobolev inequalities.
Here on $\mathbb{R}^n$. For a class $\mathrm{I}$ of test functions $\mathbb{R}^n\to\mathbb{R}$, $\exists c<\infty$, $\forall f\in\mathcal{F}$,
\begin{align*}
\mathrm{Var}_\mu (f)
&\leq c_{\mathrm{PI}}\int|\nabla f|^2\mathrm{d}\mu\\
\mathrm{Ent}_\mu(f^2)
&\leq c_{\mathrm{LSI}}\int|\nabla f|^2\mathrm{d}\mu\\
\mathrm{Ent}_\mu(f)
&\leq \frac{c_{\mathrm{LSI}}}{4}\int\frac{|\nabla f|^2}{f}\mathrm{d}\mu=\frac{c_{\mathrm{LSI}}}{4}\int|\nabla \log(f)|^2f\mathrm{d}\mu=c_{\mathrm{LSI}}\int|\nabla\sqrt{f}|^2\mathrm{d}\mu\\
\mathrm{H}(\nu\mid\mu)
&\leq\frac{c_{\mathrm{LSI}}}{4}\mathrm{I}(\nu\mid\mu)
\quad\text{(Villani notation, above is Bakry-Ledoux)}
\end{align*}
- Best (optimal) constant is smallest, infinite if impossible, depends on $\mu$ and $\mathcal{F}$
- Righ-hand side. The term $\int|\nabla f|^2\mathrm{d}\mu$ is often called energy by Bakry-Ledoux. This matches potential theory (Coulomb energy, via carré du champ électrique), Riemanian geometry (geodesics), and quantum mechanics. Bakry, Émery, and Ledoux, not specially versed in kinetic theory, information theory, or quantum mechanics, were however interested in geometric functional analysis, statistical mechanics, and some aspects of non-kinetic statistical physics.
- Beyond $\mathbb{R}^n$ : replace $\nabla$ by analogues: discrete gradient, Malliavin, etc.
- Discrete space. No chain rule, no equivalence between $1$-homogeneous and $2$-homogeneous forms of LSI, leads to several modified LSI, which can be compared possibly by restricting the class $\mathcal{F}$ of test functions.
- Markov. If $\mu$ invariant law of Markov process with generator $L$ then replace $\int|\nabla f|^2\mathrm{d}\mu$ by
\[
-\int fLf\mathrm{d}\mu\quad(\text{Dirichlet form})
\] Conversely, if one can interpret the right hand side of LSI as a Dirichlet form (quadratic form analogue of unbounded linear operators), then this leads to an operator that we can try to interpret as a Markov generator. For instance
\[
\int|\nabla f|^2\mathrm{d}x=-\int f\Delta f\mathrm{d}x
\] is typically associated via integration by parts to Laplacian, hence to Brownian motion, with boundary conditions related to the class of test functions $\mathcal{F}$ used for LSI (or PI). - Linearisation. $\mathrm{Ent}_\mu((1+\varepsilon f)^2)=\frac{\varepsilon^2}{2}\mathrm{Var}_\mu(f)+o(\varepsilon^2)$ gives
\[
c_{\mathrm{PI}}\leq\frac{1}{2}c_{\mathrm{LSI}}
\] Since PI is simpler than LSI, always try to prove PI, which is necessary for LSI. - PI and LSI not always achievable by an $f$, Rothaus alternative
- PI $\Leftrightarrow$ spectral gap of Laplacian type operator, eigenvalues
- Functional inequalities. PI and LSI, Sobolev functional spaces embeddings.
$$\begin{align*}\mathbb{E}_\mu(\Phi(f))&\leq \Phi(\mathbb{E}_\mu(f))+c\mathbb{E}_\mu(|\nabla f|^2)\\
\Phi(f)\in L^1(\mu)&\Leftarrow f\in L^1(\mu)\text{ and }|\nabla f|^2\in L^1(\mu)\end{align*}$$ - Perturbation. If $\mathrm{d}\mu_B=\mathrm{e}^B\mathrm{d}\mu$ then $c_{\mathrm{LSI}}(\mu_B)\leq\mathrm{e}^{\|B\|_{\mathrm{osc}}}c_{\mathrm{LSI}}(\mu)$ (Holley-Stroock)
- One dimensional case. Characterization by Hardy type inequalities (Muckenhoupt) : probability on $\mathbb{R}$ with density $\propto\mathrm{e}^{-c|x|^\alpha}$ satisfies PI if $\alpha\geq1$ and LSI if $\alpha\geq2$.
- Disconnected support. $c_{\mathrm{PI}}(\mu)=c_{\mathrm{LSI}}(\mu)=\infty$ if support of $\mu$ not connected
Take a non-constant $f$ which is constant on each connected component - Probabilistic functional analysis, analysis and geometry of Markov operators, geometric functional analysis. Not always related to Markov/PDE/Dynamics.
Concentration of measure for Lipschitz functions. LSI is a (sub-)gaussian statement, in which $c_{\mathrm{LSI}}$ plays the role of the norm of covariance matrix.
- Laplace transform of $F:\mathbb{R}^n\to\mathbb{R}$ for $\mu$, sub-Gaussian bound from LSI:
\[
L(\theta):=\int\mathrm{e}^{\theta F}\mathbb{d}\mu, \quad \log
L(\theta)\leq\theta^2\frac{c_{\mathrm{LSI}}\Vert F\Vert_{\mathrm{Lip}}^2}{4}+\theta\int
F\mathrm{d}\mu,\quad\forall \theta\in\mathbb{R}
\] - Proof (Herbst): $f=\mathrm{e}^{\theta F}$ in LSI for $\mu$ gives
\[
\theta L'(\theta)-L(\theta)\log
L(\theta)\leq\frac{c_{\mathrm{LSI}}}{2}\theta^2L(\theta),
\quad L(0)=1.
\] - By Markov, for all $Z\sim\mu$, $r\geq0$,
\[
\mathbb{P}(|F(Z)-\mathbb{E}F(Z)|\geq r)\leq2\exp\left(-\frac{r^2}{c_{\mathrm{LSI}}\Vert F\Vert_{\mathrm{Lip}}^2}\right).
\] - $\mathrm{Ent}_\mu$ is the Legendre transform of the log-Laplace transform in the sense that
\[
\mathrm{Ent}_\mu(f)
=\sup_g\Bigr\{\int\!fg\,\mathrm{d}\mu
-\log\int\mathrm{e}^g\,\mathrm{d}\mu\Bigr\}.
\] and conversely (convex duality)
\[
\sup_{g\geq0\atop\int g\,\mathrm{d}\mu=1}\Bigr\{\int fg\,\mathrm{d}\mu-\mathrm{Ent}_\mu(g)\Bigr\}
=\log\int\mathrm{e}^f\,\mathrm{d}\mu.
\] $\to$ Concentration of measure, transportation of measure, large deviations,
$\to$ Hopf-Lax infimum convolution solution of Hamilton-Jacobi equations. - Consequence : no LSI for exponential law and Poisson law, $\to$ modified inequalities.
- Roughly LSI = sub-gaussian at $\infty$, smoothness, and connected support.
Sub-Gaussian concentration implies LSI if curvature lower bounded (Wang) - If $X_1,\dots,X_N$ iid $\sim\mu$, $f:\mathbb{R}^n\to\mathbb{R}$, then, for all $r\geq0$,
\[
\mathbb{P}\left(
\left|\frac{f(X_1)+\cdots+f(X_N)}{N}-\mathbb{E}f(X_1)\right|\geq r\right)
\leq 2\exp\left(-\frac{Nr^2}{c_{\mathrm{LSI}}(\mu^{\otimes N})\Vert
f\Vert_{\mathrm{Lip}}^2}\right).
\] Actually, tensorization below gives: $c_{\mathrm{LSI}}(\mu^{\otimes N})\leq c_{\mathrm{LSI}}(\mu)$.
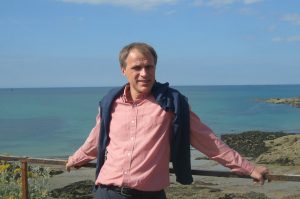
Tensorization of PI/LSI via sub-additivity of entropies and additivity of gradient.
- All these are equivalent for a convex $\Phi$, and valid for $\Phi(u)=u^2$ and $\Phi(u)=u\log(u)$ :
- Convexity : $(u,v)\mapsto\Phi”(u)v^2$ is convex
- Jensen sub-commutation : $\forall\mu_1,\mu_2$, $\forall f$, (just Cauchy-Schwarz for PI!)
\[
\mathrm{Ent}^\Phi_{\mu_2}(\mathbb{E}_{\mu_1}(f))\leq\mathbb{E}_{\mu_1}(\mathrm{Ent}^\Phi_{\mu_2}(f))
\] - Sub-additivity : $\forall n$, $\forall\mu_1,\ldots,\mu_n$, $\forall f$,
\[
\mathrm{Ent}^\Phi_{\mu_1\otimes\cdots\otimes\mu_n}(f)
\leq\sum_{i=1}^n\mathbb{E}_{\mu_1\otimes\cdots\otimes\mu_n}(\mathrm{Ent}_{\mu_i}(f))
\] - Functional convexity : $\forall\mu$,
\[
f\mapsto\mathrm{Ent}^\Phi_\mu(f)
\quad\text{is convex}
\] - Variational formula : $\forall \mu$, $\forall f$,
\[
\mathrm{Ent}^\Phi_\mu(f)=\sup_g\{\mathbb{E}_\mu((\Phi'(g)-\Phi'(\mathbb{E}_\mu
g))(f-g))-\mathrm{Ent}^\Phi_\mu(g)\}
\]
Heritage of Boltzmann, Shannon, Bobkov, Latała-Oleszkiewicz, among others.
- PI/LSI tensorization (dimension free) via $\mathrm{Ent}^\Phi$ sub-additivity and $|\nabla f|^2$ additivity
\begin{align*}
c_{\mathrm{PI}}(\mu_1\otimes\cdots\otimes\mu_n)
&\leq\max_{1\leq i\leq n}c_{\mathrm{PI}}(\mu_i)
\quad\text{and}\quad
c_{\mathrm{LSI}}(\mu_1\otimes\cdots\otimes\mu_n)
\leq\max_{1\leq i\leq n}c_{\mathrm{LSI}}(\mu_i)\\
c_{\mathrm{PI}}(\mu^{\otimes n})
&\leq c_{\mathrm{PI}}(\mu)
\quad\text{and}\quad
c_{\mathrm{LSI}}(\mu^{\otimes n})
\leq c_{\mathrm{LSI}}(\mu)
\end{align*} $x^{1\leq p\leq 2}$, Beckner, Latała-Oleszkiewicz, Arnold-Markowich-Toscani-Uerreiter, Dolbeault, etc.
From $\{-1,1\}$ to Gaussians via tensorization and the CLT.
- On two point space $\{\pm1\}$ with uniform measure and $\nabla$ replaced by $(f(1)-f(0))^2$, elementary, $a=f(-1),b=f(1)$. Poincaré is an equality :
\[
\frac{a^2\log(a^2)+b^2\log(b^2)}{2}-\frac{a^2+b^2}{2}\log\frac{a^2+b^2}{2}
\leq\frac{(a-b)^2}{2}.
\] By homogeneity $(a^2+b^2=2$, $u=a^2$), this reduces to the even simpler inequality
\[
u\log(u)+(2-u)\log(2-u)\leq (\sqrt{u}-\sqrt{2-u})^2,\quad 0\leq u\leq 2.
\] - From uniform on cube $\{-1,1\}^n$ to Gaussian on $\mathbb{R}$ via CLT
and tensorization $\frac{x_1+\cdots+x_n}{\sqrt{n}}$
\begin{align*}
c_{\mathrm{LSI}}(\mathcal{N}(0,1))
&=2\quad\text{achieved by $f(x)=\mathrm{e}^{\lambda x}$}\\
c_{\mathrm{PI}}(\mathcal{N}(0,1))
&=1\quad \text{achieved by $f(x)=\lambda
x$, also via Hermite $\perp$
polys}\\
c_{\mathrm{PI}}(\mathcal{N}(0,1))
&=\frac{1}{2}c_{\mathrm{LSI}}(\mathcal{N}(0,1))
\end{align*} - By tensorization again: for all $n\geq1$,
\begin{align*}
c_{\mathrm{LSI}}(\mathcal{N}(0,I_n)
&=\mathcal{N}(0,1)^{\otimes n}=2\quad\text{achieved by
$f(x)=\mathrm{e}^{\lambda\cdot x}$}\\
c_{\mathrm{PI}}(\mathcal{N}(0,I_n))
&=2\quad\text{achieved by
$f(x)=\lambda\cdot x$}
\end{align*} - Dimension free : Wiener measure, Loop space, Lie groups.
- Brascamp-Lieb and log-concavity
\begin{align*}
\mathrm{Ent}_{\mathcal{N}(m,K)}(f^2)
&\leq2\mathbb{E}_{\mathcal{N}(m,K)}(\langle
K\nabla f,\nabla
f\rangle^2)\leq2\lambda_{\max}(K)\mathbb{E}_{\mathcal{N}(m,K)}(|\nabla
f|^2)\\
\mathrm{Var}_{\mathrm{e}^{-V}}(f^2)
&\leq\mathbb{E}_{\mathrm{e}^{-V}}(\langle
(\mathrm{Hess}V)^{-1}\nabla f,\nabla
f\rangle)\leq\frac{1}{\rho}\mathbb{E}_{\mathrm{e}^{-V}}(|\nabla
f|^2)\quad\text{if $\mathrm{Hess}V(x)\geq\rho I_n$ $\forall x$}\\
c_{\mathrm{LSI}}
&\approx\|\text{Cov}\|\quad(\text{gaussian analogy})
\end{align*} See also Hörmander and Helffer-Sjöstrand, see also KLS conjecture.
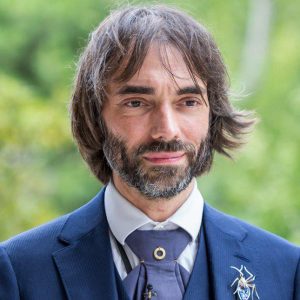
Markov and Langevin.
- ${(X_t)}_{t\geq0}$, state space $E$
- Markov semi-group $P_t f(x)=\mathbb{E}(f(X_t)\mid X_0=x)$
\[
P_0=\mathrm{id},\quad
P_{s+t}=P_s\circ P_t,\quad
P_t \mathbf 1= \mathbf 1,\quad
f\geq 0\Rightarrow P_t f \geq 0.
\] - Infinitesimal generator (BM: $L=\Delta$, OU: $L=\Delta-x\cdot\nabla$)
\[
P_t=\mathrm{e}^{tL},\quad \partial_tP_t=LP_t=P_tL
\] - Left operator on $\mu$ and right operator on $f$ :
\[
\mu P_t f=\mathbb{E}(f(X_t)),\quad X_0\sim\mu.
\] - Invariance : if $X_0\sim\mu\Rightarrow X_t\sim\mu$ $\forall t$,
\[
\mu P_t=\mu\quad\forall t,\quad \mu L=0
\] - Resersibility : $X_0\sim\mu\Rightarrow
{(X_s)}_{s\in[0,t]}\overset{\mathrm{d}}{=}{(X_{t-s})}_{s\in[0,t]}$ $\forall
t$.
\begin{eqnarray*}
\mu_t
&=&\mathrm{Law}(X_t)=\mu_0P_t,\quad
f_t=\frac{\mathrm{d}\mu_t}{\mathrm{d}\mu}=P_tf_0\\
\partial_tf_t
&=&Lf_t\quad\text{(Fokker-Planck, Chapman-Kolmogorov)}
\end{eqnarray*} - Reversibility & integration by parts via $P_t=\mathrm{id}+tL+o(t)$ ($L$ selfajoint in $L^2(\mu)$)
\[
\int fLg\mathrm{d}\mu=\int gLf\mathrm{d}\mu, \quad \forall f,g
\] - Diffusion property (replaces IBP for $P_t$ and implies IBP under $\mu$)
\[
L\phi(f)=\phi'(f)Lf+\phi”(f)|\nabla f|^2
\] Integration by parts when diffusion
\[
-\int fLg\mathrm{d}\mu
=\int\nabla f\cdot\nabla g\mathrm{d}\mu\quad\text{(integration by parts)}
\] - (Overdamped) Langevin reversible diffusion process for potential $V:\mathbb{R}^d\to\mathbb{R}$
\begin{align*}
X_t&=X_0-\int_0^t\nabla V(X_s)\mathrm{d}X_s+\sqrt{2}B_t\quad(\text{ODE
with noise})\\
L&=\Delta-\nabla V\cdot\nabla,\quad \mu\propto\mathrm{e}^{-V}
\end{align*} We focus on Langevin for simplicity in the sequel.
Gaussian case (Ornstein-Uhlenbeck): $V=\rho\frac{\left|\cdot\right|^2}{2}$, $\rho\geq0$, $\rho=0$ for BM
Many things work for general Markov, some aspects are specific to diffusions.
Entropy decay and Markov LSI.
- Monotonicity (second part with diffusion IBP since Langevin)
\[
\partial_t\mathrm{Ent}^\Phi_\mu(P_tf)
=\int\Phi'(P_tf)LP_tf\mathrm{d}\mu\leq0
\quad\Bigr(=-\int\Phi”(P_tf)|\nabla f|^2\mathrm{d}\mu\Bigr).
\] Jensen $\Phi(P_tf)-P_t(\Phi(f))\leq0$, $=$ at $t=0$ hence $\Phi'(f)Lf-L\Phi(f)\leq0$. Invariance. - Markov LSI (second requires Markov diffusion)
\[
\mathrm{Ent}^\Phi_\mu(f)
\leq-c_{\mathrm{LSI}}\int\Phi'(f)Lf\mathrm{d}\mu
\quad\Bigr(=c_{\mathrm{LSI}}\int\Phi”(f)|\nabla f|^2\mathrm{d}\mu\Bigr).
\] - Sub-exponential decay (à la Cercignani) via deBruijn and Grönwall
\[
c_{\mathrm{LSI}}\leq c
\quad\text{iif}\quad
\mathrm{Ent}^\Phi_\mu(P_tf)
\leq\mathrm{e}^{-4t/c}\mathrm{Ent}^\Phi_\mu(f),\ \forall t,\forall f
\]
Gross hypercontractivity.
- Contractivity : $\forall p\in[0,\infty]$, $\forall t\geq0$, $\forall
f$, $\|P_t(f)\|_p\leq\|f\|_p$. - Hypercontractivity (Gross theorem on characterization via LSI)
If $\mu$ is reversible or $\mu$ invariant and Markov is diffusion:
\[
c_{\mathrm{LSI}}(\mu)\leq c
\quad\Leftrightarrow\quad
\|P_t\|_{1+(p-1)\mathrm{e}^{4t/c}}\leq\|f\|_p\quad\forall f,\forall t.
\] Note : Markov version of LSI. - Proof : $\displaystyle\partial_p\|f\|_p^p=\int f^p\log(f)\mathrm{d}\mu$,
$\partial_tP_t=LP_t=P_tf$, $L^p$-$L^1$ via reversibility or diffusion.
Note : Gross forged the LSI concept here
Note : LSI is linearization of hypercontractivity
Note : historically Nelson showed hypercontractivity for OU without LSI
Note : hypercontractivity for Rademacher $\{\pm1\}$ and discrete LSI : Bonami-Beckner.
Note : combinatorial aspects of entropy rarely play a role in this universe
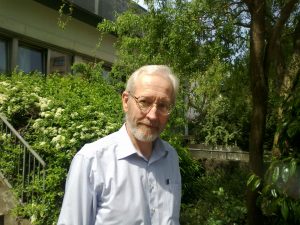
Entropy convexity along dynamics and Bakry-Émery $\Gamma_2$.
- Langevin : $L=\Delta-\nabla V\cdot\nabla$, $\mu\propto\mathrm{e}^{-V}$, $\mu_t=\mu_0P_t$, $f_t=\mathrm{d}\mu_t/\mathrm{d}\mu$.
- We have (mix of Villani Boltzmannian notation and Bakry-Ledoux notation)
\begin{align*}
\mathrm{I}(\nu\mid\mu)
&=\int\Gamma(\log f)\mathrm{d}\nu,
\quad f=\frac{\mathrm{d}\nu}{\mathrm{d}\mu},\\
\partial_t\mathrm{I}(\mu_t\mid\mu)
&=
\partial_t\int\Gamma(\log f_t)\mathrm{d}\mu_t =-2\int\Gamma_2(\log
f_t)\mathrm{d}\mu_t
\end{align*} where
\[
\Gamma(f):=|\nabla f|^2
\quad\text{and}\quad
\Gamma_2(f):=\mathrm{Tr}((\mathrm{Hess}f)^2)+\mathrm{Hess}(V)\nabla
f\cdot\nabla f.
\] We use here reversible IBP to kill all instances of $L$ and replace by $\nabla$ - Decay and convexity along dynamics (deBruijn identity and Stam inequality)
\begin{align*}
\partial_t\mathrm{H}(\mu_t\mid\mu)
&=-\mathrm{I}(\mu_t\mid\mu)\leq0 \forall t\quad(\text{H-theorem})\\
\partial_t^2\mathrm{H}(\mu_t\mid\mu)
&=2\int\Gamma_2(\log f_t)\mathrm{d}\mu_t\geq0\ \forall t
\quad(\text{Cercignany theorem})
\end{align*} $\Gamma_2\geq0$ when $V$ convex ($\mu$ log-concave) including $V$ constant (Lebesgue)
deBruijn and Stam correspond to Lebesgue measure (which is Gaussian) - Bakry-Émery $\Gamma_2$ criterion: $\rho\geq0$,
\[
\Gamma_2(f)\geq\rho\Gamma(f)\ \forall f
\quad\Leftrightarrow\quad
\mathrm{Hess}V(x)\geq\rho\ \forall x.
\] - Grönwall (motivation of $\Gamma_2$, OU and = ref model):
\begin{align*}
\partial_t\mathrm{I}(\mu_t\mid\mu)
&\leq-2\rho\mathrm{I}(\mu_t\mid\mu)\ \forall t\\
\mathrm{I}(\mu_t\mid\mu)
&\leq\mathrm{e}^{-2\rho t}
\mathrm{I}(\mu_0\mid\mu)\ \forall t
\end{align*} - LSI for log-concave, optimal for Gaussians
\begin{align*}
\mathrm{H}(\mu_0\mid\mu)
&=-\int_0^\infty-\mathrm{I}(\mu_t\mid\mu)\mathrm{d}t\\
&\leq\Bigr(\int_0^\infty\mathrm{e}^{-2\rho
t}\mathrm{d}t\Bigr)\mathrm{I}(\mu_0\mid\mu)\\
&=\frac{1}{2\rho}\mathrm{I}(\mu_0\mid\mu).
\end{align*} - Exponential decay
\[
\mathrm{H}(\mu_t\mid\mu)
\leq\mathrm{e}^{-2\rho t}
\mathrm{H}(\mu_0\mid\mu)\ \forall t.
\]
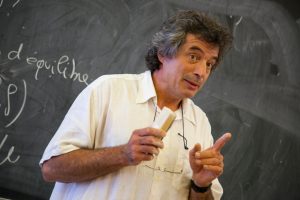
Bakry-Émery : Langevin.
- Let us show for $L=\Delta-\nabla V\cdot\nabla$ how $\Gamma_2$ emerges and gives local PI.
Comes from infinitesimal form at $t=0$, semigroup interpolation, Grönwall:
\begin{align*}
P_t(f^2)-P_t(f)^2
&=\alpha(t)-\alpha(0)=\int_0^t\alpha'(s)\mathrm{d}s\\
\alpha(s)&=P_s((P_{t-s}f)^2)\\
\alpha'(s)&=2P_s(\Gamma P_{t-s}f)\\
\alpha”(s)&=4P_s(\Gamma_2(P_{t-s}f)).
\end{align*} If $\Gamma_2\geq\rho\Gamma$ then $\alpha”\geq2\rho\alpha’$, hence by Grönwall $\alpha'(t)\geq\mathrm{e}^{2\rho t}\alpha'(0)$, and since we have $\alpha(0)=2\Gamma P_tf$, we get (for O.-U. via commutation from Mehler formula)
\[
\Gamma P_tf\leq\mathrm{e}^{-2\rho t}P_t\Gamma f.
\] Used with $t-s$ this gives in turn
\[
\alpha'(t-s)\leq2\mathrm{e}^{-2\rho(t-s)}P_sP_{t-s}\Gamma f=2\mathrm{e}^{-2\rho(t-s)}P_t\Gamma
\] where the semigroup no longer involves $s$, hence
\[
\mathrm{Var}_{P_t}(f) =\alpha(t)-\alpha(0)\leq2\frac{1-\mathrm{e}^{-2\rho t}}{2\rho}P_t\Gamma f.
\] - Bakry-Ledoux interpolation : all these are equivalent for $L=\Delta-\nabla V\cdot\nabla$
- $\mathrm{Ent}^\Phi_{P_t}(f)\leq\frac{1-\mathrm{e}^{-2\rho
t}}{2\rho}P_t(\Phi”(f)|\nabla f|^2)$, $\forall t>0,\forall f$ - $P_t(f^2)-P_t(f)^2\leq\frac{1-\mathrm{e}^{-2\rho
t}}{\rho}P_t(|\nabla f|^2)$ - $P_t(f\log f)-P_t(f)\log P_t(f)\leq\frac{1-\mathrm{e}^{-2\rho
t}}{2\rho}P_t(\frac{|\nabla f|^2}{f})$ - $I(P_tf)\leq P_t\sqrt{I(f)^2+\frac{1-\mathrm{e}^{-2\rho
t}}{2\rho}|\nabla f|^2}$, $I:=F’\circ F^{-1}$, $F:=\mathbb{P}(\mathcal{N}(0,1)\leq\cdot)$ - $|\nabla P_tf|\leq\mathrm{e}^{-\rho t}P_t|\nabla f|$
- $|\nabla P_tf|^2\leq\mathrm{e}^{-2\rho t}P_t(|\nabla f|^2)$
- $\mathrm{Hess}V(x)\geq\rho I_n$, $\forall x$
- $\mathrm{Ent}^\Phi_{P_t}(f)\leq\frac{1-\mathrm{e}^{-2\rho
- Note:
- Interpretation of sub-commutation via curvature/trajectories
For Langevin $\nabla L=L\nabla-\mathrm{Hess}V\nabla=L\nabla-\rho\nabla$
Bochner-Lichnerowicz-Weitzenböck in Riemannian geometry - We speak about the $\Gamma_2$ criterion, or Bakry-Émery criterion
- PI : weak sub-commutation is enough, no diffusion property is needed.
- These equivalences fail beyond diffusions on discrete spaces
Except PI which does not really need the diffusionproperty
Some adaptation can be done for Poisson and Lévy processes - Curvature-Dimension inequality : $\mathrm{CD}(\rho,m)$ $\Gamma_2(f)\geq\rho\Gamma(f)+\frac{1}{m}(L f)^2$.
- The $\Gamma_2$ criterion is $\mathrm{CD}(\rho,\infty)$
- On a Riemannian manifold add $\mathrm{Ric}(\nabla f,\nabla f)$ to $\Gamma_2$
Bakry-Émery tensor, Perelman on Poincaré conjecture
- Interpretation of sub-commutation via curvature/trajectories
- LSI for $P_t$ (local LSI) via diffusion property: $P_t(\cdot)(x)=\mu_t$, $\mu_0=\delta_x$, with $g:=P_{t-s}f$,
\begin{align*}
\mathrm{Ent}^\Phi_{P_t}(f)
&=\int_0^t\partial_sP_s(\Phi(P_{t-s}f))\mathrm{d}s\\
&=\int_0^tP_s(L(\Phi(g))-\Phi'(g)Lg)\mathrm{d}s\\
&=\int_0^tP_s(\Phi”(g)|\nabla g|^2)\mathrm{d}s\\
&\leq\int_0^t\mathrm{e}^{-2\rho s}P_s(\Phi”(P_{t-s}f)|P_{t-s}(|\nabla f|)^2)\mathrm{d}s\\
&\leq\int_0^t\mathrm{e}^{-2\rho s}P_s(P_{t-s}(\Phi”(f)||\nabla
f|^2)\mathrm{d}s\\
&= P_t(\Phi”(f)|\nabla f|^2)\int_0^t\mathrm{e}^{-2\rho s}\mathrm{d}s\\
&=\frac{1-\mathrm{e}^{-2\rho t}}{2\rho}
P_t(\Phi”(f)|\nabla f|^2).
\end{align*} When $t\to\infty$, recover inequality for invariant measure $\mu=P_\infty(\cdot)(x)$, $\forall x$.
Diffusion property plays for $P_t$ the role playbed by IBP for $\mu$
As for IBP, allows to kill $L$ and replace it by $\nabla$ i.e. $\Gamma$ - LSI for $\mu$ via semigroup interpolation (Bakry-Émery method, IBP for diffusion)
\begin{align*}
\mathrm{Ent}^\Phi_\mu(f)
&=-\mathbb{E}_\mu\int_0^\infty\partial_t\Phi(P_tf)\mathrm{d}t\\
&=-\mathbb{E}_\mu\int_0^\infty\Phi'(P_tf)LP_tf\mathrm{d}t\\
&=\int_0^\infty\mathbb{E}_\mu(\Phi”(P_tf)|\nabla P_tf|^2)\mathrm{d}t.
\end{align*} Bakry-Ledoux semigroup interpolation proof of LSI via sub-commutation
\begin{align*}
|\nabla P_tf|&\leq\mathrm{e}^{-\rho t}P_t|\nabla f|\\
\mathrm{Ent}^\Phi_\mu(f)
&=\int_0^\infty\mathbb{E}_\mu(\Phi”(P_tf)|\nabla P_tf|^2)\mathrm{d}t\\
&\leq\int_0^\infty\mathrm{e}^{-2\rho t}\mathbb{E}_\mu(\Phi”(P_tf)P_t(|\nabla f|)^2)\mathrm{d}t\\
&\leq\int_0^\infty\mathrm{e}^{-2\rho t}\mathbb{E}_\mu(P_t(\Phi”(f)|\nabla
f|^2))\mathrm{d}t\\
&=\mathbb{E}_\mu(\Phi”(f)|\nabla
f|^2)\int_0^\infty\mathrm{e}^{-2\rho t}\mathrm{d}t\\
&=\frac{1}{2\rho}\mathbb{E}_\mu(\Phi”(f)|\nabla f|^2).
\end{align*} - PI, spectral gap, integrated $\Gamma_2$ criterion (no diffusion, robust to discrete spaces):
\[
\mathbb{E}_\mu(\Gamma_2f)\geq\rho\mathbb{E}_\mu(\Gamma f)
\quad\Leftrightarrow\quad
c_{\mathrm{PI}}\leq\frac{1}{\rho}
\quad\Leftrightarrow\quad
\mathrm{SpectralGap}(-L)\geq\rho.
\] - Alternative via mass transportation : Caffarelli contraction theorem
\begin{align*}
\mathrm{d}\mu
&=\mathrm{e}^{-V}\mathbb{d}x, \quad\mathrm{d}\nu =\mathrm{e}^{-W}\mathbb{d}\\
\mathrm{Hess}V &\leq AI_n , \quad \mathrm{Hess}W \geq BI_n.
\end{align*} Following Caffarelli, maximum principle for Monge-Ampère implies that Brenier mass transportation map $\nabla \phi$ between $\mu$ and $\nu$ is Lipschitz with $$\|\nabla\phi\|_{\mathrm{Lip}}\leq\sqrt{A/B}$$ Works very well on $\mathbb{R}^n$ to get LSI for uniformly log-concave
Does not work very well on manifolds
Requires knowledge of Gaussian inequalities
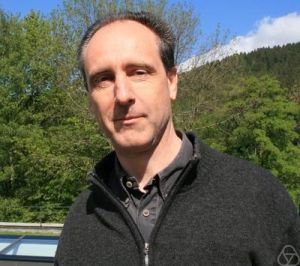
Bakry-Émery : abstract Markov.
- Abstract Markov setting (Bakry-Ledoux)
\begin{align*}
P_t&=\mathrm{e}^{tL}\\
\Gamma(f,g)&=\frac{1}{2}(L(fg)-fLg-gLf)\quad(\text{carré du champ})\\
\Gamma_2(f,g)&=\frac{1}{2}(L\Gamma(f,g)-\Gamma(f,Lg)-\Gamma(g,Lf))\\
-\int fLg\mathrm{d}\mu
&=\int\Gamma(f,g)\mathrm{d}\mu\\
L\phi(f)&=\phi'(f)Lf+\phi”(f)\Gamma(f)\\
\Gamma P_tf&\leq\mathrm{e}^{-2\rho t}P_t\Gamma f\\
\sqrt{\Gamma P_tf}&\leq\mathrm{e}^{-\rho t}P_t\sqrt{\Gamma f}\\
\mathrm{Ent}^\Phi_{P_t}(f)&\leq\frac{1-\mathrm{e}^{-2\rho t}}{2\rho}
P_t(\Phi”(f)\Gamma(f))\\
\Gamma_2(f)&\geq\rho\Gamma(f)+\frac{1}{m}(Lf)^2
\end{align*} - Problem of $\mathcal{A}$ algebra to make things rigorous
- All in all, the Bakry-Émery-Ledoux approach consists in commutations and positivity, the latter coming essentially from squares and convexity. In some sense, it is a rigid or algebraic-geometric side of probabilistic functional analysis and differential calculus.
- The Bakry-Émery approach is still available for time inhomogeneous Markov processes, with $L_t$, $\rho_t$, $\int_0^t\rho(s)\mathrm{d}s$, see for instance Collet-Malrieu.
- There exists a way to interpolate between a log-concave probability measure and a uniformly log-concave probability measure by using a Gaussian position mixture. This was explored from different perspectives by Ronen Eldan and his followers (stochastic localization), Roland Bauerschmidt and Thierry Bodineau and their followers (Polchinski equation or renormalization and multiscale interpretation). Roughly speaking, the idea is to construct a perturbation which is strictly more convex while remaining close to the original object from the covariance perspective. An interesting distant point of view on this topic is provided by Boaz Klartag (monotonicity of spectral gap) and by Yair Shenfeld (Schrödinger bridges). What is called renormalization group is often nothing else but a sort of semigroup interpolation or perturbation or regularization.
Related functional inequalities.
- LSI is linearization of Bobkov functional Gaussian isoperimetry (Beckner)
- LSI is projection of Sobolev on high dimensional spheres (Beckner)
- LSI is connected to Talagrand transportation inequalities
(Bobkov-Götze, Otto-Villani, Bobkov-Gentil-Ledoux, etc) - LSI connected to Nash inequalities and Li-Yau parabolic Harnack inequalities
- LSI for Gaussian is Shannon-Stam inequality for Lebesgue (information theory)
Statistical mechanics and beyond product measures.
- Integrated $\Gamma_2$ criterion: $c_{\mathrm{PI}}(\mu)\leq\frac{1}{\rho}$ $\Leftrightarrow$ $\mathbb{E}_\mu(\Gamma_2(f))\geq\rho\mathbb{E}_\mu(\Gamma(f))$ $\forall f$
- Only sufficient integral criteria for LSI
- $c_{\mathrm{LSI}}(\mu)<\infty$ if $\mathrm{d}\mu(x)=\mathrm{e}^{-V}\mathrm{d}x$ $V$ uniformly convex @ $\infty$ (Bodineau-Helffer)
- PI/LSI for spin systems (discrete or continuous) Glauber or Kawasaki dynamics
\[
\frac{\mathrm{e}^{-V(x)}}{Z}\mathrm{d}x,\quad\mathbb{R}^\Lambda,\quad
V(x)=\sum_iU(x_i)+\sum_{i\sim j}W(x_i,x_j).
\] Control of correlations.
Perturbative approaches.
High dimensional convexification.
Conditionnings (martingale decomposition).
(Lu-)Yau(-Landim), Zegarlinski, Martinelli, Bodineau-Helffer, Ledoux, etc.
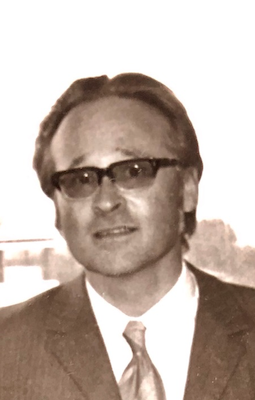
Further reading.
- C. Ané, S. Blachère, D. Chafaï, P. Fougères, I. Gentil, F. Malrieu, C. Roberto, and G. Scheffer.
Sur les inégalités de Sobolev logarithmiques
Panoramas et Synthèses 10 Société Mathématique de France (2000) - D. Bakry and M. Émery
Diffusions hypercontractives
Séminaire de probabilités XIX, Université de Strasbourg 1983/84, Lecture Notes in Mathematics 1123, 177-206 (1985) - D. Bakry, I. Gentil, and M. Ledoux.
Analysis and geometry of Markov diffusion operators
Grundlehren Math. Wiss. 348, Springer (2014) - D. Bakry and M. Ledoux
Lévy-Gromov’s isoperimetric inequality for an infinite dimensional diffusion generator
Invent. Math. 123(2):259-281 (1996) - D. Bakry and M. Ledoux
A logarithmic Sobolev form of the Li-Yau parabolic inequality
Rev. Mat. Iberoam. 22(2):683-702 (2006) - D. Bakry, M. Ledoux, and L. Saloff-Coste
Markov semigroups at Saint-Flour
Reprint (2012) of lectures originally published in the Lecture Notes in Mathematics volumes 1581 (1994), 1648 (1996) and 1665 (1997). - R. Bauerschmidt and T. Bodineau
Log-Sobolev inequality for the continuum sine-Gordon model
Commun. Pure Appl. Math. 74(10):2064-2113 (2021) - D. Chafaï
Binomial-Poisson entropic inequalities and the M/M/$\infty$ queue
ESAIM, Probab. Stat. 10:317–339 (2006) - D. Chafaï
From Boltzmann to random matrices and beyond
Ann. Fac. Sci. Toulouse, Math. 6 24(4):641–689 (2015) - J.-F. Collet and F. Malrieu
Logarithmic Sobolev inequalities for inhomogeneous Markov semigroups
ESAIM, Probab. Stat. 12:492–504 (2008) - E. B. Davies, L. Gross, and B. Simon.
Hypercontractivity: a bibliographic review
Ideas and methods in quantum and statistical physics
Oslo, 1988 370–389, Cambridge Univ. Press (1992) - J.-D. Deuschel and D. W. Stroock
Large deviations
Academic Press, rev. ed. edition (1989) - W. G. Faris
Product spaces and Nelson’s inequality
Helv. Phys. Acta 48(5/6):721–730 (1975) - P. Federbush
Partially alternate derivation of a result of Nelson
J. Math. Phys. 10:50–52 (1969) - L. Gross
Logarithmic Sobolev inequalities
Am. J. Math. 97(4):1061–1083 (1975) - L. Gross
Logarithmic Sobolev inequalities and contractivity properties of semigroups
Dirichlet Forms, Varenna, 1992, Lecture Notes in Math. 1563, 54–88, Springer (1993) - L. Gross
Hypercontractivity, logarithmic Sobolev inequalities, and applications: a survey of surveys.
Diffusion, Quantum Theory, and Radically Elementary Mathematics
Math. Notes 47, 45–73. Princeton Univ. Press (2006) - A. Guionnet and B. Zegarlinski
Lectures on logarithmic Sobolev inequalities
Séminaire de probabilités XXXVI, 1-134. Springer (2003) - B. Helffer
Semiclassical analysis, Witten Laplacians, and statistical mechanics
World Scientific (2002) - E. P. Hsu
Stochastic analysis on manifolds
Grad. Stud. Math. 38 American Mathematical Society (2002) - B. Klartag and Putterman
Spectral monotonicity under Gaussian convolution
arXiv 2107.09496 To appear in Annales de la Faculté des Sciences de Toulouse - B. Klartag
Logarithmic bounds for isoperimetry and slices of convex sets
arXiv:2303.14938 - M. Ledoux
Concentration of measure and logarithmic Sobolev inequalities
Séminaire de probabilités XXXIII, pages 120-216. Springer (1999) - M. Ledoux
The geometry of Markov diffusion generators
Ann. Fac. Sci. Toulouse, Math. (6) 9(2):305–366 (2000) - M. Ledoux
Logarithmic Sobolev inequalities for unbounded spin systems revisited
Séminaire de Probabilités XXXV, 167-194. Springer (2001) - M. Ledoux
Heat flows, geometric and functional inequalities
Proceedings of the International Congress of Mathematicians (ICM 2014), Seoul, Korea, August 13-21 (2014) Vol. IV: Invited lectures pages 117-135. - M. Ledoux
More than fifteen proofs of the logarithmic Sobolev inequality
Historical note available on personal webpage - M. Ledoux
Curvature-Dimension
Historical note available on personal webpage - F. Martinelli
Lectures on Glauber dynamics for discrete spin models.
Lectures on probability theory and statistics. Ecole d’été de Probabilités de Saint-Flour XXVII-1997 July 7–23, 1997}, pages 93-191. Springer (1999) - R. Montenegro and P. Tetali
Mathematical aspects of mixing times in Markov chains
Found. Trends Theor. Comput. Sci. 1(3):237–354 (2005) - G. Royer
An initiation to logarithmic Sobolev inequalities
SMF/AMS Texts Monogr. 14 American Mathematical Society and Société Mathématique de France (2007) - L. Saloff-Coste
Aspects of Sobolev-type inequalities
Lond. Math. Soc. Lect. Note Ser. 289 Cambridge University Press (2002) - Y. Shenfeld
Exact renormalization groups and transportation of measures
arXiv:2205.01642 - A. J. Stam
Some inequalities satisfied by the quantities of information of Fisher and Shannon
Inf. Control 2:101-112 (1959) - C. Villani
Optimal transport. Old and new
Grundlehren Math. Wiss. 338 Springer (2009) - F.-Y. Wang
Analysis for diffusion processes on Riemannian manifolds
Adv. Ser. Stat. Sci. Appl. Probab. 18 World Scientific (2014)
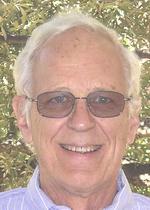