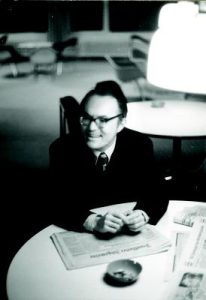
If you know what are Poincaré and log-Sobolev inequalities, you may ask what makes variance and entropy so special. Bloody hell?! This is an old natural question. This tiny post is about $\Phi$-entropies, a subject that was explored two or three decades ago, and which provides an answer based on convexity. Convexity is something important in probabilistic functional analysis, which cannot be reduced to the Cauchy-Schwarz inequality, and which interacts with the integration by parts formula and the Bochner formula. In particular, it is useful for the study of the analysis and geometry of discrete and continuous Markov processes.
Phi-entropy. Let $\Phi:I\to\mathbb{R}\cup\{+\infty\}$ be strictly convex, defined on a closed interval $I\subset\mathbb{R}$ with non-empty interior, finite and $\mathcal{C}^4$ on the interior of $I$. Let $(\Omega,\mathcal{A},\mu)$ be a probability space, and $f:\Omega\to I$ such that $f\in L^1(\mu)$ and $\Phi(f)\in L^1(\mu)$. Following for instance [L], the $\Phi$-entropy (actually relative entropy) of $f$ with respect to $\mu$ is defined by \[ \mathrm{Ent}^\Phi_\mu(f) =\int\Phi(f)\mathrm{d}\mu-\Phi\Bigr(\int f\mathrm{d}\mu\Bigr). \] The Jensen inequality gives $\mathrm{Ent}^\Phi_\mu(f)\geq0$, with equality iff $f$ is constant $\mu$-almost-everywhere. We can recover the convexity of $\Phi$ from the non-negativity of $\mathrm{Ent}^\Phi_\mu$ by considering the probability measure $\mu=(1-t)\delta_u+t\delta_v$ for arbitrary $t\in[0,1]$ and $u,v\in I$.
The $\Phi$-entropy can be seen as a Jensen divergence. It is linear with respect to $\Phi$, and invariant by additive affine perturbations on $\Phi$. Basic examples include the following:
- Variance : $\Phi(u)=u^2$ on $I=\mathbb{R}$
- Entropy : $\Phi(u)=u\log(u)$ on $I=[0,+\infty)$
- In between interpolation : $\Phi(u)=\frac{u^p-u}{p-1}$ on $I=[0,+\infty)$, $1 < p\leq2$.
The case $p > 2$ produces a valid $\Phi$-entropy, however, as explained in the sequel, the $\Phi$-entropy in this case is not convex with respect to its functional argument, and therefore, it does not have a variational formula nor a tensorization property.
A convex functional subset. The following set is convex: \[ L^\Phi(\mu)=\{f\in L^1(\mu):\Phi(f)\in L^1(\mu)\}. \] This suggests to study the convexity of $f\in L^1(\mu)\mapsto\mathrm{Ent}^\Phi_\mu(f)$ (we do it in the sequel).
Indeed, let $f,g\in L^\Phi(\mu)$ and $\lambda\in[0,1]$. Then $\lambda f+(1-\lambda) g\in L^1(\mu)$. Next, since $\Phi$ is convex and since $x\in\mathbb{R}\mapsto x_+=\max(0,x)$ is convex and non-decreasing, we get \[ \Phi(\lambda f+(1-\lambda)g)_+ \leq(\lambda\Phi(f)+(1-\lambda)\Phi(g))_+ \leq\lambda\Phi(f)_++(1-\lambda)\Phi(g)_+. \] Therefore $\Phi(\lambda f+(1-\lambda)g)_+\in L^1(\mu)$. On the other hand, since $\Phi$ is convex, there exists an affine function $\psi$ such that $\Phi\geq\psi$, therefore \[ \Phi(\lambda f+(1-\lambda)g)\geq\psi(\lambda f+(1-\lambda)g)\in L^1(\mu), \] which implies $\Phi(\lambda f+(1-\lambda)g)\in L^1(\mu)$. We have proved that $\lambda f+(1-\lambda)g\in L^\Phi(\mu)$.
Approximation. Let $\mathcal{F}$ be the set of measurable functions $f:\Omega\to I$ such that $f(\Omega)$ is a compact subset of the interior of $I$. In particular, if $f\in\mathcal{F}$ then both $f$ and $\Phi(f)$ are bounded. We have $\mathcal{F}\subset L^\Phi(\mu)$ and $\mathcal{F}$ is convex. If $(\Omega,\mathcal{A})$ is nice enough, such as $\mathbb{R}^d$, then $\mathcal{F}$ is dense in the sense that for all $f\in L^\Phi(\mu)$, there exists a sequence ${(f_n)}$ in $\mathcal{F}$ such that $f_n\to f$ and $\Phi(f_n)\to\Phi(f)$ in $L^1(\mu)$. In particular $\mathrm{Ent}^\Phi_\mu(f_n)\to\mathrm{Ent}^\Phi_\mu(f)$. We always assume that this approximation property is satisfied in the sequel.
Characterization of convexity. The following properties are equivalent:
- $f\mapsto\mathrm{Ent}^\Phi_\mu(f)$ is convex on $L^\Phi(\mu)$, for all $(\Omega,\mathcal{A},\mu)$
- $(u,v)\mapsto C^\Phi(u,v)=\Phi''(u)v^2$ is convex on $J=\{(u,v)\in\mathbb{R}^2:u\in I,u+v\in I\}$.
Indeed, the convexity of $\mathrm{Ent}^\Phi_\mu$ is a univariate property, equivalent to state that \[ t\in[0,1]\mapsto\alpha(t)=\mathrm{Ent}^\Phi_\mu((1-t)f+tg) \] is convex for all $f,g\in\mathcal{F}$. It is in turn equivalent to $\alpha''\geq0$ for all $f,g\in\mathcal{F}$, and since $f$ and $g$ are free, it is equivalent to $\alpha''(0)\geq0$ for all $f,g\in\mathcal{F}$. Now \begin{align*} \alpha'(t) &=\int\Phi'(f+t(g-f))(g-f)\mathrm{d}\mu-\Phi'\Big(\int(f+t(g-f))\mathrm{d}\mu\Bigr)\int(g-f)\mathrm{d}\mu\\ &=\int\Bigr(\Phi'(f+t(g-f))-\Phi'\Big(\int(f+t(g-f))\mathrm{d}\mu\Bigr)\Bigr)(g-f)\mathrm{d}\mu.\\ \alpha''(t) &=\int\Phi''(f+t(g-f))(g-f)^2\mathrm{d}\mu-\Phi''\Big(\int(f+t(g-f))\mathrm{d}\mu\Bigr)\Bigr(\int(g-f)\mathrm{d}\mu\Bigr)^2. \end{align*} Hence $\alpha''(0)$ is actually nothing else but the $C^\Phi$-entropy for bivariate functions: \begin{align*} \alpha''(0) &=\int\Phi''(f)(g-f)^2\mathrm{d}\mu-\Phi''\Big(\int f\mathrm{d}\mu\Bigr)\Bigr(\int(g-f)\mathrm{d}\mu\Bigr)^2\\ &=\mathrm{Ent}^{C^\Phi}_\mu((f,g-f)). \end{align*} Now the convexity of $C^\Phi$ implies $\alpha''(0)\geq0$ and thus the convexity of $\mathrm{Ent}^\Phi_\mu$. Conversely, if $\mathrm{Ent}^\Phi_\mu$ is convex for all $\mu$, then the $C^\Phi$-entropy is $\geq0$ for all $\mu$, Used in particular with $\mu=(1-t)\delta_{(u,v)}+t\delta_{(u',v')}$, $t\in[0,1]$, $(u,v),(u',v')\in J$, this gives the convexity of $C^\Phi$.
More convexity. The following properties are equivalent
- $(u,v)\mapsto A^\Phi(u,v)=\Phi(u+v)-\Phi(u)-\Phi'(u)v$ is convex on $J$.
- $(u,v)\mapsto B^\Phi(u,v)=(\Phi'(u+v)-\Phi'(u))v$ is convex on $J$.
- $(u,v)\mapsto C^\Phi(u,v)=\Phi''(u)v^2$ is convex on $J$.
- Either $\Phi$ is affine on $I$, or $\Phi'' > 0$ and $1/\Phi''$ is concave on $I$.
Indeed, the equivalence between the convexity of $A^\Phi$, $B^\Phi$, and $C^\Phi$ comes from \[ A^\Phi(u,v)=\int_0^1(1-p)C^\Phi(u+pv,v)\mathrm{d}p, \quad B^\Phi(u,v)=\int_0^1C^\Phi(u+pv,v)\mathrm{d}p, \] \[ A^\Phi(u,\varepsilon v)=\tfrac{1}{2}C^\Phi(u,v)\varepsilon^2+o(\varepsilon^2), \quad B^\Phi(u,\varepsilon v)=C^\Phi(u,v)\varepsilon^2+o(\varepsilon^2). \] Finally, for the equivalence between the convexity of $A^\Phi$ and of $-1/\Phi''$, we start from \[ \mathrm{Hess}A^\Phi(u,v) =\begin{pmatrix} A^{\Phi''}(u,v) & \Phi''(u+v)-\Phi''(u) \\ \Phi''(u+v)-\Phi''(u) & \Phi''(u+v) \end{pmatrix}. \] If $A^\Phi$ is convex, then the diagonal elements of $\mathrm{Hess}A^\Phi(u,v)$ are $\geq0$, and thus $\Phi''\geq0$. Moreover the convexity of $A^\Phi$ yields $\det(\mathrm{Hess}A^\Phi(u,v))\geq0$. Now, if $\Phi''(u+v)=0$, then $\det(\mathrm{Hess}A^\Phi(u,v))=-\Phi''(u)^2$, and thus $\Phi''(u)=0$, therefore $\{w:\Phi''(w)=0\}$ is either empty of equal to $I$ ($\Phi$ is the affine). If $\Phi'' > 0$ on $I$, then it turns out that \[ \det(\mathrm{Hess}A^\Phi(u,v))=\Phi''(u+v)\Phi''^2(u)A^{-1/\Phi''}(u,v), \] which is $\geq0$ since $A^\Phi$ is convex. Since $\Phi'' > 0$, we get $A^{-1/\Phi''}\geq0$, thus $-1/\Phi''$ is convex.
Conversely, if $\Phi$ is affine, then $A^\Phi$ is $\equiv0$, hence convex. If $\Phi'' > 0$ and $-1/\Phi''$ is convex, then $(-1/\Phi'')''=(\Phi''''\Phi''-2\Phi'''^2)/\Phi''^3$ hence $\Phi''''\Phi''\geq2\Phi'''^2$, thus $\Phi''''\geq0$, which gives $A^{\Phi''}\geq0$, therefore the diagonal elements of $\mathrm{Hess}A^\Phi(u,v)$ are $\geq0$. On the other hand, $\det(\mathrm{Hess}A^\Phi(u,v))=\Phi''(u+v)\Phi''^2(u)A^{-1/\Phi''}(u,v)\geq0$, therefore $A^\Phi$ is convex.
By moving only along the $u$ variable, we see that the convexity of $C^\Phi$ implies the one of $\Phi''$. However the converse is false : the convexity of $\Phi''$ does not imply the one of $C^\Phi$. For instance if $\Phi(u)=u^4$ and $I=[0,+\infty)$, then $1/\Phi''(u)=1/(12u^2)$ is not concave.
Variational formula. The map $f\mapsto\mathrm{Ent}^\Phi_\mu(f)$ is convex iff for all $f\in\mathcal{F}$, \[ \mathrm{Ent}^\Phi_\mu(f) =\sup_{g\in\mathcal{F}}\Bigr(\mathrm{Ent}^\Phi_\mu(g)+\int\Bigr(\Phi'(g)-\Phi'\Bigr(\int g\mathrm{d}\mu\Bigr)\Bigr)(f-g)\mathrm{d}\mu\Bigr), \] and equality is achieved when $f=g$. Indeed, this formula expresses $\mathrm{Ent}^\Phi_\mu$ as a supremum of affine functions, showing that $\mathrm{Ent}^\Phi_\mu$ is convex. The convexity on $\mathcal{F}$ and on $L^\Phi(\mu)$ are equivalent by approximation. Conversely, if $\mathrm{Ent}^\Phi_\mu$ is convex, then for all $f,g\in\mathcal{F}$, \[ \alpha:t\in[0,1]\mapsto\alpha(t)=\mathrm{Ent}^\Phi_\mu((1-t)f+tg) \] is convex and differentiable, equal to the envelope of its affine tangents. In particular, \[ \alpha(0)=\sup_{t\in[0,1]}(\alpha(t)+\alpha'(t)(0-t)), \] and equality is achieved when $t=0$, as well as when $t=1$ if $f=g$. But recall that \begin{align*} \alpha'(t) &=\int\Phi'(f+t(g-f))(g-f)\mathrm{d}\mu-\Phi'\Big(\int(f+t(g-f))\mathrm{d}\mu\Bigr)\int(g-f)\mathrm{d}\mu\\ &=\int\Bigr(\Phi'(f+t(g-f))-\Phi'\Big(\int(f+t(g-f))\mathrm{d}\mu\Bigr)\Bigr)(g-f)\mathrm{d}\mu. \end{align*} Finally, the desired variational formula comes from \[ \mathrm{Ent}^\Phi_\mu(f) =\alpha(0) =\sup_{g\in\mathcal{F}}(\alpha(1)+\alpha'(1)(0-1)). \]
Tensorization inequality. If $f\mapsto\mathrm{Ent}^\Phi_\mu(f)$ is convex for all $(\Omega,\mathcal{A},\mu)$, then for all $n\geq1$, all $\mu=\mu_1\otimes\cdots\otimes\mu_n$ on a product space $(\Omega_1\times\cdots\times\Omega_n,\mathcal{A}_1\otimes\cdots\otimes\mathcal{A}_n)$, all $f\in\mathcal{F}$, denoting $\mathrm{Ent}^\Phi_{\mu_i}(f)$ the partial $\Phi$-entropy of $f$ with respect to the $i$-th variable, \[ \mathrm{Ent}^\Phi_{\mu}(f)\leq\sum_{i=1}^n\int\mathrm{Ent}^\Phi_{\mu_i}(f)\mathrm{d}\mu. \] Indeed, it suffices to prove the case $n=2$. Now, denoting $g_1=\int g\mathrm{d}\mu_1$, we note that \[ \int\mathrm{Ent}^\Phi_{\mu_1}(g)\mathrm{d}\mu_2 +\int\mathrm{Ent}^\Phi_{\mu_2}(g_1)\mathrm{d}\mu_1 =\mathrm{Ent}^\Phi_\mu(g). \] Therefore, by using the variational formula for $\mu_1,g$ and $\mu_2,g_1$, we get \begin{align*} \int\mathrm{Ent}^\Phi_{\mu_1}(f)\mathrm{d}\mu_2 +\int\mathrm{Ent}^\Phi_{\mu_2}(f)\mathrm{d}\mu_1 &\geq\int\mathrm{Ent}^\Phi_{\mu_1}(g)\mathrm{d}\mu_2\\ &\quad+\int\Bigr(\Phi'(g)-\Phi'\Bigr(\int g\mathrm{d}\mu_1\Bigr)\Bigr)(f-g)\mathrm{d}\mu_1\mathrm{d}\mu_2\\ &\quad+\int\mathrm{Ent}^\Phi_{\mu_2}(g_1)\mathrm{d}\mu_1\\ &\quad+\int\Bigr(\Phi'(g_1)-\Phi'\Bigr(\int g_1\mathrm{d}\mu_2\Bigr)\Bigr)(f-g_1)\mathrm{d}\mu_2\mathrm{d}\mu_1\\ &=\mathrm{Ent}^\Phi_{\mu}(g)\\ &\quad+\int\Bigr(\Phi'(g)-\Phi'(g_1)\Bigr)(f-g)\mathrm{d}\mu_1\mathrm{d}\mu_2\\ &\quad+\int\Bigr(\Phi'(g_1)-\Phi'\Bigr(\int g\mathrm{d}\mu\Bigr)\Bigr)(f-g)\mathrm{d}\mu_2\mathrm{d}\mu_1\\ &=\mathrm{Ent}^\Phi_\mu(g)\\ &\quad+\int\Bigr(\Phi'(g)-\Phi'\Bigr(\int g\mathrm{d}\mu\Bigr)\Bigr)(f-g)\mathrm{d}\mu. \end{align*} It remains to take the supremum over $g$ and use the variational formula for $\mu$.
The tensorization inequality is actually equivalent to the convexity of $\mathrm{Ent}^\Phi_\mu$. More precisely, on the product space $\{0,1\}\times\Omega$ equipped with $((1-p)\delta_0+p\delta_1)\otimes\mu$, the tensorization for $f$ defined by $f(i,y)=g_i(y)$ gives, after rearrangement, \[ \mathrm{Ent}^\Phi_\mu((1-p)g_1+pg_2) \leq(1-p)\mathrm{Ent}^\Phi_\mu(g_1)+p\mathrm{Ent}^\Phi_\mu(g_2). \]
Variance and entropy.
- If $\Phi(u)=u^2$ and $I=\mathbb{R}$, then $1/\Phi''(u)=2$, which is concave.
- If $\Phi(u)=u\log(u)$ and $I=[0,+\infty)$, then $1/\Phi''(u)=u$, which is concave.
- If $\Phi(u)=\frac{u^p-u}{p-1}$, $p > 1$, $I=[0,+\infty)$, then $1/\Phi''(u)=u^{2-p}/p$, concave iff $p\leq2$.
This shows that the entropy and variance cases are actually critical or extremal : $p=1^+$ and $p=2$ respectively. Even if it is not apparent on $1/\Phi''$, the linearity of $A^\Phi,B^\Phi,C^\Phi$ with respect to $\Phi$ implies that the set of convex $\Phi$ for which they are convex is a convex non-negative cone, in other words stable by taking finite or infinite linear combinations on $\Phi$ with non-negative coefficients (a sum or more generally an integral with respect to a positive measure). In particular $\Phi(u)=au^2+bu\log(u)$, $a,b\geq0$ works.
Phi-Sobolev inequalities for diffusions. Let ${(X_t)}_{t\geq0}$ be the Markov process solving the stochastic differential equation (also known as an overdamped Langevin diffusion) \[ \mathrm{d}X_t=\sqrt{2}\mathrm{d}B_t-\nabla V(X_t)\mathrm{d}t \] where $B$ is a Brownian motion on $\mathbb{R}^d$ and $V:\mathbb{R}^d\to\mathbb{R}$ is $\mathcal{C}^2$ with $V-\frac{\rho}{2}\left|\cdot\right|^2$ convex for some $\rho\in\mathbb{R}$. This ensures that there is no explosion in finite time, and the process ${(X_t)}_{t\geq0}$ is well defined. In the special case in which $V=\frac{\rho}{2}\left|\cdot\right|^2$ for a constant $\rho\geq0$, we get the Ornstein-Uhlenbeck process, for which we have the explicit (Mehler) formula \[ \mathrm{Law}(X_t\mid X_0=x)=\mathcal{N}(x\mathrm{e}^{-\rho t},(1-\mathrm{e}^{-2\rho})I_d). \] For any bounded and measurable $f:\mathbb{R}^d\to\mathbb{R}$ and $x\in\mathbb{R}^d$, we set \[ \mathrm{P}_t(f)(x)=\mathbb{E}(f(X_t)\mid X_0=x), \quad\text{namely}\quad \mathrm{P}_t(\cdot)(x)=\mathrm{Law}(X_t\mid X_0=x). \] This also defines a linear operator on bounded measurable functions $\mathrm{P}_t:f\mapsto\mathrm{P}_t(f)$. We have $\mathrm{P}_0=\mathrm{Id}$, and the Markov nature of $X$ translates into a semigroup property: \[ \mathrm{P}_{t+s}=\mathrm{P}_t\mathrm{P}_s=\mathrm{P}_s\mathrm{P}_t,\quad s,t\geq0. \] The semigroup acts on functions (right) and on measures (left): \[ \mathbb{E}(f(X_t))=\int \mathrm{P}_t(f)\mathrm{d}\nu=\nu \mathrm{P}_tf\quad\text{when}\quad X_0\sim\nu. \] Since the stochastic differential equation involves only the gradient of $V$, we can add to it a constant to make $\mu=\mathrm{e}^{-V}\mathrm{d}x$ a probability measure on $\mathbb{R}^d$. This probability measure is invariant: if $X_0\sim\mu$ then $X_t\sim\mu$ for all $t\geq0$, in other words $\mu\mathrm{P}_t=\mu$ for all $t\geq0$. The semigroup ${(\mathrm{P}_t)}_{t\geq0}$ leaves invariant $L^p(\mu)$ for all $p\in[1,\infty]$. The infinitesimal generator of this semigroup is the linear differential operator $\mathrm{L}=\Delta-\nabla V\cdot\nabla$, namely \[ \partial_t\mathrm{P}_tf=\mathrm{L}\mathrm{P}_tf=\mathrm{P}_t\mathrm{L}f. \] The integration by parts gives, for all rapidly decaying $\mathcal{C}^2$ functions $f$ and $g$, \[ \int f\mathrm{L}g\mathrm{d}\mu =-\int\nabla f\cdot\nabla g\mathrm{d}\mu =\int g\mathrm{L}f\mathrm{d}\mu. \] We recover the invariance, $\partial_t\int \mathrm{P}_t(f)\mathrm{d}\mu=0$, and moreover \begin{align*} \partial_t\mathrm{Ent}^\Phi_\mu(\mathrm{P}_tf) &=\int\Phi'(\mathrm{P}_tf)\mathrm{L}\mathrm{P}_tf\mathrm{d}\mu\\ &=-\int\Phi''(\mathrm{P}_tf)|\nabla \mathrm{P}_tf|^2\mathrm{d}\mu\\ &=-\int C^\Phi(\mathrm{P}_tf,|\nabla \mathrm{P}_tf|)\mathrm{d}\mu\leq0. \end{align*} This can be seen as a sort of Boltzmann H-theorem for the evolution equation $\partial_tf_t=\mathrm{L}f_t$ where $f_t=\mathrm{P}_tf$ is the density of $X_t$ with respect to $\mu$. Now, following Dominique Bakry, the Bochner commutation formula $\nabla\mathrm{L}=\mathrm{L}\nabla-(\mathrm{Hess}V)\nabla$ gives \[ |\nabla\mathrm{P}_tf|\leq\mathrm{e}^{-\rho t}\mathrm{P}_t|\nabla f|, \] hence, by the bivariate Jensen inequality for the convex function $C^\Phi$ and the law $\mathrm{P}_t(\cdot)(x)$, \[ C^\Phi(\mathrm{P}_tf,|\nabla\mathrm{P}_tf|) \leq \mathrm{e}^{-2\rho t}C^\Phi(\mathrm{P}_tf,\mathrm{P}_t|\nabla f|) \leq \mathrm{e}^{-2\rho t}\mathrm{P}_tC^\Phi(f,|\nabla f|). \] This gives, using again the invariance $\mu \mathrm{P}_t=\mu$ for the last equality, \begin{align*} \mathrm{Ent}^\Phi_\mu(f)-\mathrm{Ent}^\Phi_\mu(\mathrm{P}_Tf) &=-\int_0^T\partial_t\mathrm{Ent}^\Phi_\mu(\mathrm{P}_tf)\mathrm{d}t\\ &=\int_0^T\int C^\Phi(\mathrm{P}_tf,|\nabla \mathrm{P}_tf|)\mathrm{d}\mu\mathrm{d}t\\ &\leq\Bigr(\int_0^T\mathrm{e}^{-2\rho t}\mathrm{d}t\Bigr)\Bigr(\int \mathrm{P}_t(C^\Phi(f,|\nabla f|))\mathrm{d}\mu\Bigr)\\ &=\frac{1-\mathrm{e}^{-2\rho T}}{2\rho}\int C^\Phi(f,|\nabla f|)\mathrm{d}\mu. \end{align*} Alternatively, instead of using the Jensen inequality with $C^\Phi$, we could use the Cauchy-Schwarz inequality and the Jensen inequality for the concave function $1/\Phi''$ as \[ (\mathrm{P}_t|\nabla f|)^2 \leq \mathrm{P}_t(\Phi''(f)|\nabla f|^2)\mathrm{P}_t\Bigr(\frac{1}{\Phi''(f)}\Bigr) \leq \frac{\mathrm{P}_t(\Phi''(f)|\nabla f|^2)}{\mathrm{P}_t(\Phi''(f))}. \] Now when $\rho > 0$, the process is ergodic : $\mathrm{Law}(X_t)\to\mu$ as $t\to\infty$, regardless of $X_0$. In other words $\mathrm{P}_t(\cdot)(x)\to\mu$ as $t\to\infty$, for all $x$. In particular, $\mathrm{P}_Tf\to\int f\mathrm{d}\mu$, which is constant, as $T\to\infty$, giving the following $\Phi$-Sobolev inequality for $\mu$: \[ \mathrm{Ent}^\Phi_\mu(f) \leq\frac{1}{2\rho}\int C^\Phi(f,|\nabla f|)\mathrm{d}\mu. \] This is a Poincaré inequality when $\Phi(u)=u^2$, a logarithmic Sobolev inequality when $\Phi(u)=u\log(u)$, and a Beckner inequality when $\Phi(u)=\frac{u^p-u}{p-1}$, $1 < p\leq2$.
Local inequalities for diffusions. It is also possible to get similar inequalities, even when $\rho=0$, for $\mathrm{P}_t(\cdot)(x)$ instead of $\mu=\mathrm{P}_\infty(\cdot)(x)$, by using the interpolation $\mathrm{P}_{t-s}\Phi(\mathrm{P}_{s}f)$, and replacing the integration by parts formula by the diffusion property \[ \mathrm{L}(\Phi(f))-\Phi'(f)\mathrm{L}f =\Phi''(f)|\nabla f|^2=C^\Phi(f,|\nabla f|). \] Namely, for all $t\in\mathbb{R}_+$, all $x\in\mathbb{R}^d$, and all $f:\mathbb{R}^d\to I$, \[ \mathrm{Ent}^\Phi_{\mathrm{P}_t(\cdot)(x)}(f) =\mathrm{P}_t(\Phi(f))(x)-\Phi(\mathrm{P}_t(f)(x)) =\int_0^t\partial_s\mathrm{P}_s(\Phi(\mathrm{P}_{t-s}f))(x)\mathrm{d}s. \] Dropping the notation $(x)$, we get, from the diffusion property, denoting $g=\mathrm{P}_{t-s}f$, \[ \partial_s\mathrm{P}_s\Phi(\mathrm{P}_{t-s}f) =\mathrm{P}_s(\mathrm{L}\Phi(g)-\Phi'(g)\mathrm{L}g) =\mathrm{P}_s(\Phi''(f)|\nabla g|^2) =\mathrm{P}_sC^\Phi(g,|\nabla g|). \] Now recall that the Bochner formula gives $|\nabla g|\leq\mathrm{e}^{-\rho(t-s)}\mathrm{P}_{t-s}|\nabla f|$, hence, by the Jensen inequality for the bivariate convex function $C^\Phi$, and the semigroup property, \begin{align*} \mathrm{P}_sC^\Phi(g,|\nabla g|) &\leq\mathrm{e}^{-\rho(t-s)}\mathrm{P}_sC^\Phi(\mathrm{P}_{t-s}f,\mathrm{P}_{t-s}|\nabla f|)\\ &\leq\mathrm{e}^{-\rho(t-s)}\mathrm{P}_s\mathrm{P}_{t-s}C^\Phi(f,|\nabla f|)\\ &=\mathrm{P}_tC^\Phi(f,|\nabla f|). \end{align*} This gives finally the following local $\Phi$-Sobolev inequality: \[ \mathrm{Ent}^\Phi_{\mathrm{P}_t(\cdot)}(f) \leq\Bigr(\int_0^t\mathrm{e}^{-\rho(t-s)}\mathrm{d}s\Bigr)\mathrm{P}_tC^\Phi(f,|\nabla f|) =\frac{1-\mathrm{e}^{-\rho t}}{\rho}\mathrm{P}_tC^\Phi(f,|\nabla f|). \] The formula is still valid when $\rho=0$ as soon as we use the natural convention $\frac{1-\mathrm{e}^{-\rho t}}{\rho}=t$. When $\rho > 0$ and $t\to\infty$, we recover the inequality for the invariant law $\mu=\mathrm{P}_\infty(\cdot)(x)$. Moreover it can be checked that the constants in front of the right hand side are optimal (smallest) in the case of Brownian motion and Ornstein-Uhlenbeck processes.
Modified inequalities for Poisson. Fix any $\lambda\geq0$, we consider the Poisson law \[ \pi_\lambda=\mathrm{e}^{-\lambda}\sum_{x\in\mathbb{N}}\frac{\lambda^x}{x!}\delta_x \] Then for all convex $\Phi$ on $I\subset\mathbb{R}$ with $\Phi''$ convex as before, and all $f:\mathbb{N}\to I$ bounded, \[ \mathrm{Ent}^\Phi_{\pi_\lambda}(f)\leq\lambda\mathbb{E}_{\pi_\lambda}(A^\Phi(f,\mathrm{D}f)) \] where $\mathrm{D}(f)(x)=f(x+1)-f(x)$. Following [C1, C2], let us give a simple proof melting the semigroups of Dominique Bakry and the bivariate convexity of Liming Wu. Let ${(X_t)}_{t\in\mathbb{R}_+}$ be the simple Poisson process on $\mathbb{N}$ with intensity $\lambda > 0$. Then \[ \mathrm{P}_t(\cdot)(x)=\mathrm{Law}(X_t\mid X_0=x)=\delta_x*\pi_{\lambda t}. \] In other words $\mathrm{P}_t(f)(x)=\mathbb{E}(f(X_t)\mid X_0=x)=\mathbb{E}(f(x+Z_t))$ where $Z_t\sim\pi_{\lambda t}$. The infinitesimal generator is the difference operator given for $f:\mathbb{N}\to\mathbb{R}$ and $x\in\mathbb{N}$ by \[ \mathrm{L}(f)(x)=\lambda f(x+1)-f(x)=\lambda(\mathrm{D}f)(x). \] Now \[ \mathrm{Ent}^\Phi_{\pi_{\lambda t}} =\mathrm{P}_t(\Phi(f))(x)-\Phi(\mathrm{P}_t(f)(x)) =\int_0^t\partial_s\mathrm{P}_s(\Phi(\mathrm{P}_{t-s}f))(x)\mathrm{d}s. \] Dropping the notation $(x)$ and setting $g=\mathrm{P}_{t-s}f$, we get \[ \partial_s\mathrm{P}_s(\Phi(\mathrm{P}_{t-s}f)) =\mathrm{P}_s(\mathrm{L}\Phi(g)-\Phi'(g)\mathrm{L}g) =\lambda\mathrm{P}_s(A^\Phi(g,\mathrm{D}g)). \] The commutation $\mathrm{D}\mathrm{L}=\mathrm{L}\mathrm{D}$ gives $\mathrm{D}\mathrm{P}_t=\mathrm{P}_t\mathrm{D}$, in particular $\mathrm{D}g=\mathrm{P}_{t-s}\mathrm{D}f$, hence, by the Jensen inequality for the bivariate convex function $A^\Phi$ and the semigroup property, \[ \mathrm{P}_sA(g,\mathrm{D}g) =\mathrm{P}_s(A(\mathrm{P}_{t-s}f,\mathrm{P}_{t-s}\mathrm{D}f)) \leq\mathrm{P}_s(\mathrm{P}_{t-s}A(f,\mathrm{D}f)) =\mathrm{P}_tA(f,\mathrm{D}f). \] Finally we obtain a local $\Phi$-Sobolev inequality for the simple Poisson process: \[ \mathrm{Ent}^\Phi_{\mathrm{P}_t(\cdot)}(f) \leq\Big(\int_0^t\lambda\mathrm{d}s\Bigr)\mathrm{P}_tA(f,\mathrm{D}f) =\lambda t\mathrm{P}_tA(f,\mathrm{D}f). \] In particular for $x=0$ and $t=1$ we get a $\Phi$-Sobolev inequality for the Poisson law: \[ \mathrm{Ent}^\Phi_{\pi_{\lambda}}(f) \leq\lambda\mathbb{E}_{\pi_{\lambda}}(A(f,\mathrm{D}f)). \] It can be checked that the constant $\lambda$ in the right hand side is optimal (smallest).
The lack of chain rule in discrete space produces a lack of diffusion property, which is circumvented here by using $A^\Phi$ and convexity. This $A^\Phi$-based modified inequality can be generalized to Poisson point processes by using stochastic calculus, see [W1].
Modified inequalities for Poisson again. We have seen above that $C^\Phi$- based $\Phi$-Sobolev inequalities for the Gaussian law can be obtained by semigroup interpolation and the Bochner formula via two distinct methods:
- See the Gaussian law as the invariant law of the Ornstein-Uhlenbeck process, the overdamped Langevin with $V=\frac{1}{2}\left|\cdot\right|^2$. This approach involves an integration by parts of the generator. The obtained inequality is at equilibrium for the process.
- See the Gaussian law as the law at time $t$ of a Brownian motion, the overdamped Langevin with $V\equiv0$. This approach involves the diffusion property of the generator. The obtained inequality is a local inequality for the process.
The simple Poisson process plays for the Poisson law the role played by Brownian motion for the Gaussian law. As we have seen, this gives an $A^\Phi$-based $\Phi$-Sobolev inequality for the Poisson law via a local $A^\Phi$-based $\Phi$-Sobolev inequality for the simple Poisson process. Is there an analogue of the Ornstein-Uhlenbeck process for the Poisson law ? Following [C2], it turns out that the answer is positive. It is the M/M/$\infty$ queue, for which the Poisson law is invariant. It allows to get a $B^\Phi$-based $\Phi$-Sobolev inequality for the Poisson law.
More precisely, the M/M/$\infty$ queue is a Markov process ${(X_t)}_{t\in\mathbb{R}_+}$ with state space $\mathbb{N}$ and infinitesimal generator given for $f:\mathbb{N}\to\mathbb{R}$ and $x\in\mathbb{N}$ by \[ \mathrm{L}(f)(x)=\lambda\mathrm{D}(f)(x)+x\mu\mathrm{D}^*(f)(x) \] where $\mathrm{D}(f)(x)=f(x+1)-f(x)$, $\mathrm{D}^*(f)(x)=f(x-1)-f(x)$, and where $\lambda > 0$ and $\mu > 0$ are parameters. We have a discrete analogue of the Mehler formula: \[ \mathrm{P}_t(\cdot)(x) =\mathrm{Law}(X_t\mid X_0=x) =\mathrm{Binomial}(x,\mathrm{e}^{-\mu t})*\mathrm{Poisson}(\rho(1-\mathrm{e}^{-\mu t})) \] where $\rho=\lambda/\mu$. In particular the Poisson law $\pi_\rho=\mathrm{Poisson}(\rho)$ is invariant. Moreover it is reversible and we have the integration by parts formula \[ \int f\mathrm{L}g\mathrm{d}\pi_\rho =-\lambda\int(\mathrm{D}f)(\mathrm{D}g)\mathrm{d}\pi_\rho =\int g\mathrm{L}f\mathrm{d}\pi_\rho. \] Furthermore, we have the following discrete Bochner formula \[ \mathrm{D}\mathrm{L}=\mathrm{L}\mathrm{D}-\mu\mathrm{D} \quad\text{and}\quad \mathrm{D}\mathrm{P}_t=\mathrm{e}^{-\mu t}\mathrm{P}_t\mathrm{D} \] Now, denoting $f_t=\mathrm{P}_tf$, and using the invariance and integration by parts, \[ -\partial_t\mathrm{Ent}^\Phi_{\pi_\rho}(f_t) =\int\Phi'(f_t)\mathrm{L}f_t\mathrm{d}\pi_\rho =\lambda\int\mathrm{D}(\Phi'(f_t))\mathrm{D}f_t)\mathrm{d}\pi_\rho =\int B^\Phi(f_t,\mathrm{D}f_t)\mathrm{d}\pi_\rho. \] Next, by using the discrete Bochner formula, the inequality $B^\Phi(u,pv)\leq p B^\Phi(u,v)$ for $p\in[0,1]$, and the Jensen inequality for $\mathrm{P}_t(\cdot)(x)$ and the convex function $B^\Phi$, we get \[ B^\Phi(f_t,\mathrm{D}f_t) =B^\Phi(f_t,\mathrm{e}^{-\mu t}(\mathrm{D}f)_t) \leq\mathrm{e}^{-\mu t}\mathrm{P}_tB^\Phi(f,\mathrm{D}f). \] Therefore, by using $\mathrm{Ent}^\Phi_{\pi_\rho}(f_\infty)=0$ and the invariance of $\pi_\rho$, we get (recall that $f_0=f$) \[ \mathrm{Ent}^\Phi_{\pi_\rho}(f) =-\int_0^\infty\partial_t\mathrm{Ent}^\Phi_{\pi_\rho}(f_t)\mathrm{d}\pi_\rho \leq\lambda\Bigr(\int_0^\infty\mathrm{e}^{-\mu t}\mathrm{d}t\Bigr)\int B^\Phi(f,\mathrm{D}f)\mathrm{d}\pi_\rho =\rho\int B^\Phi(f,\mathrm{D}f)\mathrm{d}\pi_\rho. \] We have thus obtained a $B^\Phi$-based $\Phi$-Sobolev inequality for the Poisson law $\pi_\rho$: \[ \mathrm{Ent}^\Phi_{\pi_\rho}(f)\leq\rho\mathbb{E}_{\pi_\rho}B^\Phi(f,\mathrm{D}f). \] It can be checked that the constant in front of the right hand side is optimal (smallest).
Convex $\Phi$-transforms and modified $\Phi$-Sobolev inequalities.
\begin{align*} A^\Phi(f,\mathrm{D} f) &=\mathrm{D}\Phi(f)-\Phi'(f)\mathrm{D} f\\ B^\Phi(f,\mathrm{D} f) &=\mathrm{D}(\Phi'(f))\mathrm{D} f\\ C^\Phi(f,\mathrm{D} f) &=\Phi''(f)(\mathrm{D} f)^2 \end{align*}
$$ \begin{array}{lll} \boldsymbol{\Phi(u)} & \boldsymbol{A^\Phi(u,v)} & \boldsymbol{A^\Phi(f,\mathrm{D} f)} \\ u\log(u) & \displaystyle{(u+v)(\log(u+v)-\log(u))-v} & (f+\mathrm{D} f)\mathrm{D}(\log f)-\mathrm{D} f\\ u^2 & v^2 & (\mathrm{D} f)^2 \\ u^p & \displaystyle{(u+v)^p-u^p-p u^{p-1}v} & \mathrm{D}(f^p)-p f^{p-1}\mathrm{D} f \\ & \boldsymbol{B^\Phi(u,v)} & \boldsymbol{B^\Phi(f,\mathrm{D} f)} \\ u\log(u) & \displaystyle{v(\log(u+v)-\log(u))} & \mathrm{D}(f)\mathrm{D}(\log f) \\ u^2 & 2v^2 & 2(\mathrm{D} f)^2 \\ u^p & \displaystyle{p v((u+v)^{p-1}-u^{p-1})} & p \mathrm{D}(f)\mathrm{D}(f^{p-1}) \\ & \boldsymbol{C^\Phi(u,v)} & \boldsymbol{C^\Phi(f,\mathrm{D} f)} \\ u\log(u) & \displaystyle{v^2 u^{-1}} & (\mathrm{D} f)^2 f^{-1} \\ u^2 & 2v^2 & 2(\mathrm{D} f)^2 \\ u^p & \displaystyle{p(p-1)v^2u^{p-2}} & p(p-1)(\mathrm{D} f)^2 f^{p-2} \end{array} $$
The function $A^\Phi$ is known in convex analysis as a Bregman divergence.
The tensorization can be used to get $\Phi$-entropy inequalities for Gauss and Poisson laws from two-point space, as Gross did for the log-Sobolev inequality in [G], see [C2]. This can be pushed further to infinite dimension (Wiener measure and Poisson space).
Further comments. This post is a revival of an article written in 2010 for an online encyclopedia of functional inequalities, a MoinMoin wiki run by the former EVOL ANR research project. Well, this blog will also disappear at some point! The content of this post is taken from [C1-C2], with corrections and simplifications. The main motivation of [C1] was to explore a unification and generalization, incorporating as much as possible [H], [LO], [W1], among other works, using convexity and the semigroup approach of Dominique Bakry. Unfortunately, the main results in [C2] are buried in Section 4, never do that! The writing of [C1] and [C2] was done in parallel of [BRC] and [M], and without being aware of [AMTU] and [BT] respectively. The variational formula and the tensorization of $\Phi$-entropies is also considered or mentioned to some extent in [L] and [BT]. There are now many works on the topic, including for instance [Co] and [LRS].
Further reading.
- [ABD] Arnold, A. and Bartier, J.-Ph. and Dolbeault, J.
Interpolation between logarithmic Sobolev and Poincaré inequalities.
Commun. Math. Sci. 5 (2007), no. 4, 971--979. - [AMTU] Arnold, A. and Markowich, P. and Toscani, G. and Unterreiter, A.
On convex Sobolev inequalities and the rate of convergence to equilibrium for Fokker-Planck type equations.
Comm. Partial Differential Equations 26 (2001), no. 1-2, 43--100. - [B] Beckner, W.
A generalized Poincaré inequality for Gaussian measures.
Proceedings of the American Mathematical Society, pages 397--400, 1989. - [BCR] Barthe, F. and Cattiaux, P. and Roberto, C.
Interpolated inequalities between exponential and Gaussian Orlicz hypercontractivity and isoperimetry.
Rev. Mat. Iberoam. 22 (2006), no. 3, 993--1067. - [BE] Bakry, D. and Émery, M.
Hypercontractive diffusions
Lecture Notes in Math., 1123 Springer, 1985, 177--206. - [BGL] Bakry, D. and Gentil, I. and Ledoux, M.
Analysis and geometry of Markov diffusion operators
Springer, 2014, xx+552 pp. - [BaL] Bakry, D. and Ledoux, M.
Lévy-Gromov's isoperimetric inequality for an infinite-dimensional diffusion generator
Invent. Math. 123 (1996), no. 2, 259-281. - [BoL] Bobkov, S. G. and Ledoux, M.
On modified logarithmic Sobolev inequalities for Bernoulli and Poisson measures.
J. Funct. Anal. 156 (1998), no. 2, 347--365 - [BT] Bobkov, S. and Tetali, P.
Modified logarithmic Sobolev inequalities in discrete settings
J. Theoret. Probab. 19 (2006), no. 2, 289--336. - [Br] Brègman, L. M.
A relaxation method of finding a common point of convex sets and its application to the solution of problems in convex programming.
Z. Vyčisl. Mat. i Mat. Fiz. 7 1967 620--631. - [C1] Chafaï, D.
Entropies, convexity, and functional inequalities: on $\Phi$-entropies and $\Phi$-Sobolev inequalities.
J. Math. Kyoto Univ., 44(2):325–363, 2004. - [C2] Chafaï, D.
Binomial-Poisson entropic inequalities and the $M/M/ \infty$ queue.
ESAIM Probab. Stat. 10 (2006), 317--339. - [CL] Chafaï, D. and Lehec, J.
Logarithmic Sobolev Inequalities Essentials
Master 2 Lecture Notes (2017) Available online - [Co] Conforti, G.
A probabilistic approach to convex Phi-entropy decay for Markov chains.
Ann. Appl. Probab. 32 (2022), no. 2, 932-973. - [Cs] Csiszár, I.
A class of measures of informativity of observation channels. Collection of articles dedicated to the memory of Alfréd Rényi
I. Period. Math. Hungar. 2 (1972), 191--213. - [G] Gross, L.
Logarithmic Sobolev inequalities.
Amer. J. Math. 97 (1975), no. 4, 1061--1083. - [H] Hu, Y.-Z.
A unified approach to several inequalities for Gaussian and diffusion measures.
Séminaire de Probabilités, XXXIV, 329--335, Lecture Notes in Math., 1729, Springer, Berlin, 2000. - [L] Ledoux, M.
On Talagrand's deviation inequalities for product measures.
ESAIM Probab. Statist. 1 (1995/97), 63--87. - [LO] Latała, R. and Oleszkiewicz, K.
Between Sobolev and Poincaré.
Geometric aspects of functional analysis, 147--168, Lecture Notes in Math., 1745, Springer, Berlin, 2000. - [LRS] López-Rivera, P. and Shenfeld Y.
The Poisson transport map.
Preprint arXiv:2407.02359 (2024) - [M] Massart, P. Concentration inequalities and model selection. Lectures from the 33rd Summer School on Probability Theory held in Saint-Flour, July 6-23, 2003. With a foreword by Jean Picard.
Lecture Notes in Mathematics, 1896. Springer, Berlin, 2007. xiv+337 pp. - [MC] Malrieu, F. and Collet, J.-F.
Logarithmic Sobolev Inequalities for Inhomogeneous Semigroups
ESAIM PS, 12 (2008), pp 492--504. - [W1] Wu, L.
A new modified logarithmic Sobolev inequality for Poisson point processes and several applications.
Probab. Theory Related Fields 118 (2000), no. 3, 427--438. - [W2] Wu, L.
A Phi-entropy contraction inequality for Gaussian vectors.
J. Theoret. Probab. 22 (2009), no. 4, 983--991.