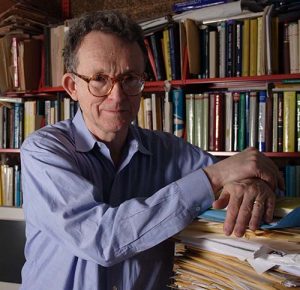
This post is about some few distances or divergences between probability measures on the same space, by default $\mathbb{R}^n$, or even $\mathbb{R}$. They generate useful but different topologies.
The first one is familiar in Statistics:
$$
\chi^2(\nu\mid\mu)
=\mathrm{Var}_\mu\Bigr(\frac{\mathrm{d}\nu}{\mathrm{d}\mu}\Bigr)
=\Bigr\|\frac{\mathrm{d}\nu}{\mathrm{d}\mu}-1\Bigr\|^2_{L^2(\mu)}.
$$ The second one is also known as the Kullback-Leibler information divergence or relative entropy:
$$
\mathrm{Kullback}(\nu\mid\mu)
=\int\log\frac{\mathrm{d}\nu}{\mathrm{d}\mu}\mathrm{d}\nu
=\int\frac{\mathrm{d}\nu}{\mathrm{d}\mu}\log\frac{\mathrm{d}\nu}{\mathrm{d}\mu}\mathrm{d}\mu.
$$ The third one is the Fisher information, which can be seen as a logarithmic Sobolev norm:
$$
\mathrm{Fisher}(\nu\mid\mu)
=\int\Bigr|\nabla\log\frac{\mathrm{d}\nu}{\mathrm{d}\mu}\Bigr|^2\mathrm{d}\nu
=4\int\Bigr|\nabla\sqrt{\frac{\mathrm{d}\nu}{\mathrm{d}\mu}}\Bigr|^2\mathrm{d}\mu
$$ The fourth one is the order $2$ Monge-Kantorovich-Wasserstein Euclidean coupling distance
$$
\mathrm{Wasserstein}^2(\mu,\nu)
=\inf_{(X_\mu,X_\nu)}\mathbb{E}(\tfrac{1}{2}|X_\mu-X_\nu|^2)
=\sup_{f,g}\Bigr(\int f\mathrm{d}\mu-\int g\mathrm{d}\nu\Bigr)
$$ where the infimum runs over all couples $(X_\mu,X_\nu)$ of random variables on the product space with marginal laws $\mu$ and $\nu$, and where the supremum runs over all bounded and Lipschitz $f$ and $g$ such that $f(x)-g(y)\leq\frac{1}{2}|x-y|^2$. The optimal $f$ is given by the infimum convolution $f(x)=\inf_y(g(y)+\frac{1}{2}|x-y|^2$ for all $x,y$, which is the Hopf-Lax solution at unit time of the Hamilton-Jacobi equation $\partial f_t+\frac{1}{2}|\nabla_x f_t|^2=0$ with $f_0=g$. The passage from an infimum formulation to a supremum formulation is an instance of the Kantorovich-Rubinstein duality.
The fifth one is the total variation distance:
$$\begin{align*}
\|\mu-\nu\|_{\mathrm{TV}}
&=\inf_{(X_\mu,X_\nu)}\mathbb{E}(\mathbf{1}_{X_\mu\neq X_\nu}) \\
&=\sup_{\|f\|_\infty\leq\frac{1}{2}}\Bigr(\int f\mathrm{d}\mu-\int f\mathrm{d}\nu\Bigr) \\
&=\sup_A|\nu(A)-\mu(A)|=\tfrac{1}{2}\|\varphi_\mu-\varphi_\nu\|_{L^1(\lambda)}
\end{align*}$$ where $\varphi_\mu$ and $\varphi_\nu$ are the densities of $\mu$ and $\nu$ with respect to any common reference measure $\lambda$ when such a measure exists. The first expression states that it can be seen as a Monge-Kantorovich-Wasserstein coupling distance of order $1$ for the atomic distance on the underlying space. The total variation distance makes no difference between small differences and big differences, in contrast with the Euclidean Wasserstein distance introduced previously. The sixth and last one is the Hellinger distance:
$$
\mathrm{Hellinger}^2(\mu,\nu)
=\tfrac{1}{2}\|\sqrt{\varphi_\mu}-\sqrt{\varphi_\nu}\|^2_{L^2(\lambda)}
$$ which turns out to be essentially equivalent with total variation, while allowing explicit formulas for tensor products and Gaussians.
Some universal comparisons.
$$
\begin{align*}
\left\Vert\mu-\nu\right\Vert_{\mathrm{TV}}^2
&\leq2\mathrm{Kullback}(\nu\mid\mu)\\
2\mathrm{Hellinger}^2(\mu,\nu)
&\leq\mathrm{Kullback}(\nu\mid\mu)\\
\mathrm{Kullback}(\nu\mid\mu)
&\leq 2\chi(\nu\mid\mu)+\chi^2(\nu\mid\mu)\\
\mathrm{Hellinger}^2(\mu,\nu)\leq\|\mu-\nu\|_{\mathrm{TV}}
&\leq \mathrm{Hellinger}(\mu,\nu)\sqrt{2-\mathrm{Hellinger}^2(\mu,\nu)}
\end{align*}
$$
Contraction. From the variational formulas, if $\mathrm{dist}\in\{\mathrm{TV}, \mathrm{Kullback}, \chi^2\}$ then
$$
\mathrm{dist}(\nu \circ f^{-1}\mid \mu \circ f^{-1})
\leq \mathrm{dist}(\nu \mid \mu).
$$In the same spirit, if $f:\mathbb{R}^n\to\mathbb{R}^k$, then
$$
\mathrm{Wasserstein}(\mu\circ f^{-1},\nu\circ f^{-1})
\leq\left\|f\right\|_{\mathrm{Lip}}\mathrm{Wasserstein}(\mu,\nu)
$$
Tensorisation.
$$
\begin{align*}
\mathrm{Hellinger}^2(\otimes_{i=1}^n\mu_i,\otimes_{i=1}^n\nu_i)
&=1-\prod_{i=1}^n\Bigr(1-\mathrm{Hellinger}^2(\mu_i,\nu_i)\Bigr)\\
\mathrm{Kullback}(\otimes_{i=1}^n\nu_i\mid\otimes_{i=1}^n\mu_i)
&=\sum_{i=1}^n\mathrm{Kullback}(\nu_i\mid\mu_i)\\
\chi^2(\otimes_{i=1}^n\mu_i\mid\otimes_{i=1}^n\nu_i)
&=-1+\prod_{i=1}^n(\chi^2(\mu_i,\nu_i)+1)\\
\mathrm{Fisher}(\otimes_{i=1}^n\nu_i\mid\otimes_{i=1}^n\mu_i)
&=\sum_{i=1}^n\mathrm{Fisher}(\nu_i\mid\mu_i)\\
\mathrm{Wasserstein}^2(\otimes_{i=1}^n\mu_i,\otimes_{i=1}^n\nu_i)
&=\sum_{i=1}^n\mathrm{Wasserstein}^2(\mu_i,\nu_i)\\
\max_{1\leq i\leq n}\Vert\mu_i-\nu_i\Vert_{\mathrm{TV}}
\leq\Vert\otimes_{i=1}^n\mu_i&-\otimes_{i=1}^n\nu_i\Vert_{\mathrm{TV}}
\leq\sum_{i=1}^n\Vert\mu_i-\nu_i\Vert_{\mathrm{TV}}
\end{align*}
$$
Monotonicity. If ${(X_t)}_{t\geq0}$ is a continuous time Markov process, ergodic with unique invariant probability measure $\mu$, then, denoting $\mu_t=\mathrm{Law}(X_t)$,
$$
\mathrm{dist}(\mu_t\mid\mu)\underset{t\to\infty}{\searrow}0
$$provided that $\mathrm{dist}$ is convex. Actually, if $\nu\ll\mu$, then
$$
\begin{align*}
\mathrm{dist}(\nu\mid\mu)
&=\int\Phi(\tfrac{\mathrm{d}\nu}{\mathrm{d}\mu})\mathrm{d}\mu\\
\Phi(u)
&=\begin{cases}
u^2-1 & \mathrm{if }\mathrm{dist}=\chi^2\\
u\log(u) & \mathrm{if }\mathrm{dist}=\mathrm{Kullback}\\
\frac{1}{2}|u-1| & \mathrm{if }\mathrm{dist}=\mathrm{TV}\\
\frac{1}{2}(1-\sqrt{u}) & \mathrm{if }\mathrm{dist}=\mathrm{Hellinger}^2
\end{cases}
\end{align*}
$$
In the case of Fisher and Wasserstein, the monotonicity along the time is not always true, but it always holds for the overdamped Langevin diffusion solving the stochastic differential equation $$\mathrm{d}X_t=\sqrt{2}\mathrm{d}B_t-\nabla V(X_t)\mathrm{d}t$$provided that the potential $V:\mathbb{R}^d\to\mathbb{R}$ is convex, but this is not obvious at all!
Further reading. There are of course plenty of other distances and divergences between probability measures, such as the Lévy-Prokhorov metric, the Fortet-Mourier or Dudley bounded-Lipschitz metric, the Kolmogorov-Smirnov metric, etc.
Further reading.
- Gibbs, Alison L. and Su, Francis Edward
On choosing and bounding probability metrics
International Statistical Review / Revue Internationale de Statistique 70(3) 419-435 (2002) - Pollard, David
A user's guide to measure theoretic probability
Cambridge University Press (2002) - Rachev, Svetlozar Todorov
Probability Metrics and the Stability of Stochastic Models
Wiley (1991)