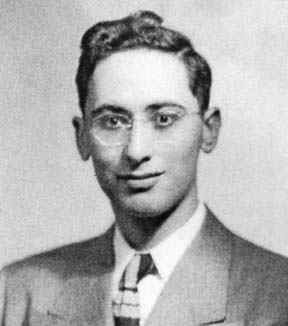
This post is devoted to a sub-Gaussian tail bound and exponential square integrability for local martingales, taken from my master course on stochastic calculus.
Sub-Gaussian tail bound and exponential square integrability for local martingales. Let \( {M={(M_t)}_{t\geq0}} \) be a continuous local martingale issued from the origin. Then for all \( {t,K,r\geq0} \),
\[ \mathbb{P}\Bigr(\sup_{s\in[0,t]}|M_s|\geq r, \langle M\rangle_t\leq K\Bigr) \leq2\mathrm{e}^{-\frac{r^2}{2K}}, \]
and in particular, if \( {\langle M\rangle_t\leq Ct} \) then
\[ \mathbb{P}\Bigr(\sup_{s\in[0,t]}|M_s|\geq r\Bigr) \leq2\mathrm{e}^{-\frac{r^2}{2Ct}} \]
and, for all \( {\alpha<\frac{1}{2Ct}} \),
\[ \mathbb{E}\Bigr(\mathrm{e}^{\alpha\sup_{s\in[0,t]}|M_s|^2}\Bigr)<\infty. \]
The condition \( {\langle M\rangle_t\leq Ct} \) is a comparison to Brownian motion for which equality holds.
Proof. For all \( {\lambda,t\geq0} \), the Doléans-Dade exponential
\[ X^\lambda ={\Bigr(\mathrm{e}^{\lambda M_t-\frac{\lambda^2}{2}\langle M\rangle_t}\Bigr)}_{t\geq0} \]
is a positive super-martingale with \( {X^\lambda_0=1} \) and \( {\mathbb{E}X^\lambda_t\leq1} \) for all \( {t\geq0} \). For all \( {t,\lambda,r,K\geq0} \), by using the maximal inequality for the super-martingale \( {X^\lambda} \) in the last step,
\[ \begin{array}{rcl} \mathbb{P}\Bigr(\langle M\rangle_t\leq K,\sup_{0\leq s\leq t}M_s\geq r\Bigr) &\leq&\mathbb{P}\Bigr(\langle M\rangle_t\leq K,\sup_{0\leq s\leq t}X^\lambda_s\geq\mathrm{e}^{\lambda r-\frac{\lambda^2}{2}K}\Bigr) \\ &\leq&\mathbb{P}\Bigr(\sup_{0\leq s\leq t}X^\lambda_s\geq\mathrm{e}^{\lambda r-\frac{\lambda^2}{2}K}\Bigr)\\ &\leq&\mathbb{E}(X^\lambda_0)\mathrm{e}^{-\lambda r+\frac{\lambda^2}{2}K} =\mathrm{e}^{-\lambda r+\frac{\lambda^2}{2}K}. \end{array} \]
Taking \( {\lambda=r/K} \) gives
\[ \mathbb{P}\Bigr(\langle M\rangle_t\leq K,\sup_{0\leq s\leq t}M_s\geq r\Bigr) \leq\mathrm{e}^{-\frac{r^2}{2K}}. \]
The same reasoning for \( {-M} \) instead of \( {M} \) provides (note that \( {\langle -M\rangle=\langle M\rangle} \) obviously)
\[ \mathbb{P}\Bigr(\langle M\rangle_t\leq K,\sup_{0\leq s\leq t}(-M_s)\geq r\Bigr) \leq\mathrm{e}^{-\frac{r^2}{2K}}. \]
The union bound (hence the prefactor \( {2} \)) gives then the first desired inequality. The exponential square integrability comes from the usual link between tail bound and integrability, namely if \( {X=\sup_{s\in[0,t]}|M_s|} \), \( {U(x)=\mathrm{e}^{\alpha x^2}} \), \( {\alpha<\frac{1}{2Kt}} \), then, by Fubini-Tonelli,
\[ \begin{array}{rcl} \mathbb{E}(U(X)) &=&\mathbb{E}\Bigr(\int_0^XU'(x)\mathrm{d}x\Bigr)\\ &=&\mathbb{E}\Bigr(\int_0^\infty\mathbf{1}_{x\leq X}U'(x)\mathrm{d}x\Bigr)\\ &=&\int_0^\infty U'(x)\mathbb{P}(X\geq x)\mathrm{d}x\ &\leq&\int_0^\infty2\alpha x\mathrm{e}^{\alpha x^2}\mathrm{e}^{-\frac{x^2}{2Kt}}\mathrm{d}x <\infty. \end{array} \]
Doob maximal inequality for super-martingales. If \( {M} \) is a continuous super-martingale, then for all \( {t\geq0} \) and \( {\lambda>0} \), denoting \( {M^-=\max(0,-M)} \),
\[ \mathbb{P}\Bigr(\max_{s\in[0,t]}|M_s|\geq\lambda\Bigr) \leq\frac{\mathbb{E}(M_0)+2\mathbb{E}(M^-_t)}{\lambda}. \]
In particular when \( {M} \) is non-negative then \( {\mathbb{E}(M^-)=0} \) and the upper bound is \( {\frac{\mathbb{E}(M_0)}{\lambda}} \).
Proof. Let us define the bounded stopping time
\[ T=t\wedge \inf\{s\in[0,t]:M_s\geq \lambda\}. \]
We have \( {M_T\in\mathrm{L}^1} \) since \( {|M_T|\leq\max(|M_0|,|M_t|,\lambda)} \). By the Doob stopping theorem for the sub-martingale \( {-M} \) and the bounded stopping times \( {0} \) and \( {T} \) that satisfy \( {M_0\in\mathrm{L}^1} \) and \( {M_T\in\mathrm{L}^1} \), we get
\[ \mathbb{E}(M_0) \geq\mathbb{E}(M_T) \geq \lambda\mathbb{P}(\max_{s\in[0,t]}M_s\geq \lambda) +\mathbb{E}(M_t\mathbf{1}_{\max_{s\in[0,t]}M_s<\lambda}) \]
hence, recalling that \( {M^-=\max(-M,0)} \),
\[ \lambda\mathbb{P}(\max_{s\in[0,t]}M_s\geq \lambda) \leq \mathbb{E}(M_0)+\mathbb{E}(M^-_t). \]
This produces the desired inequality when \( {M} \) is non-negative. For the general case, we observe that the Jensen inequality for the nondecreasing convex function \( {u\in\mathbb{R}\mapsto\max(u,0)} \) and the sub-martingale \( {-M} \) shows that \( {M^-} \) is a sub-martingale. Thus, by the Doop maximal inequality for non-negative sub-martingales,
\[ \lambda\mathbb{P}(\max_{s\in[0,t]}M^-_s\geq \lambda) \leq\mathbb{E}(M^-_t). \]
Finally, putting both inequalities together gives
\[ \lambda\mathbb{P}(\max_{s\in[0,t]}|M_s|\geq \lambda) \leq \lambda\mathbb{P}(\max_{s\in[0,t]}M_s\geq \lambda) +\lambda\mathbb{P}(\max_{s\in[0,t]}M^-_s\geq \lambda) \leq\mathbb{E}(M_0)+2\mathbb{E}(M^-_t). \]
Doob maximal inequalities. Let \( {M} \) be a continuous process.
- If \( {M} \) is a martingale or a non-negative sub-martingale then for all \( {p\geq1} \), \( {t\geq0} \), \( {\lambda>0} \),
\[ \mathbb{P}\Bigr(\max_{s\in[0,t]}|M_s|\geq\lambda\Bigr) \leq\frac{\mathbb{E}(|M_t|^p)}{\lambda^p}. \]
- If \( {M} \) is a martingale then for all \( {p>1} \) and \( {t\geq0} \),
\[ \mathbb{E}\Bigr(\max_{s\in[0,t]}|M_s|^p\Bigr) \leq\Bigr(\frac{p}{p-1}\Bigr)^p\mathbb{E}(|M_t|^p) \]
in other words
\[ \Bigr\|\max_{s\in[0,t]}|M_s|\Bigr\|_p\leq\frac{p}{p-1}\|M_t\|_p. \]
In particular if \( {M_t\in\mathrm{L}^p} \) then \( {M^*_t=\max_{s\in[0,t]}M_s\in\mathrm{L}^p} \).
Comments. This inequality allows to control the tail of the supremum by the moment at the terminal time. It is a continuous time martingale version of the simpler Kolmogorov maximal inequality for sums of independent and identically distributed random variables. Note that \( {q=1/(1-1/p)=p/(p-1)} \) is the Hölder conjugate of \( {p} \) namely \( {1/p+1/q=1} \). The inequality is often used with \( {p=2} \), for which \( {(p/(p-1))^p=4} \).
Proof. We can always assume that the right hand side is finite, otherwise the inequalities are trivial.
- If \( {M} \) is a martingale, then by the Jensen inequality for the convex function \( {u\in\mathbb{R}\mapsto |u|^p} \), the process \( {|M|^p} \) is a sub-martingale. Similarly, If \( {M} \) is a non-negative sub-martingale then, since \( {u\in[0,+\infty)\mapsto u^p} \) is convex and non-decreasing it follows that \( {M^p=|M|^p} \) is a sub-martingale. Therefore in all cases \( {{(|M_s|^p)}_{s\in[0,t]}} \) is a sub-martingale. Let us define the bounded stopping time
\[ T=t\wedge \inf\{s\geq0:|M_s|\geq\lambda\}. \]
Note that \( {|M_T|\leq\max(|M_0|,\lambda)} \) and thus \( {M_T\in\mathrm{L}^1} \). The Doob stopping theorem for the sub-martingale \( {|M|^p} \) and the bounded stopping times \( {T} \) and \( {t} \) that satisfy \( {T\leq t} \) gives
\[ \mathbb{E}(|M_T|^p)\leq\mathbb{E}(|M_t|^p). \]
On the other hand the definition of \( {T} \) gives
\[ |M_T|^p \geq\lambda^p\mathbf{1}_{\max_{s\in[0,t]}|M_s|\geq\lambda} +|M_t|^p\mathbf{1}_{\max_{s\in[0,t]}|M_s|<\lambda}\\ \geq\lambda^p\mathbf{1}_{\max_{s\in[0,t]}|M_s|\geq\lambda}. \]
It remains to combine these inequalities to get the desired result.
- If we introduce for all \( {n\geq1} \) the “localization” stopping time
\[ T_n=t\wedge\inf\{s\geq0:|M_s|\geq n\}, \]
then the desired inequality for the bounded sub-martingale \( {{(|M_{s\wedge T_n}|)}_{s\in[0,t]}} \) would give
\[ \mathbb{E}(\max_{s\in[0,t]}|M_{s\wedge T_n}|^p) \leq\left(\frac{p}{p-1}\right)^p\mathbb{E}(|M_t|^p), \]
and the desired result for \( {{(M_s)}_{s\in[0,t]}} \) would then follow by monotone convergence theorem. Thus this shows that we can assume without loss of generality that \( {{(|M_s|)}_{s\in[0,t]}} \) is bounded, in particular that \( {\mathbb{E}(\max_{s\in[0,t]}|M_s|^p)<\infty} \). This a martingale localization argument! The previous proof gives
\[ \mathbb{P}(\max_{s\in[0,t]}|M_s|\geq\lambda) \leq\frac{\mathbb{E}(|M_t|\mathbf{1}_{\max_{s\in[0,t]}|M_s|\geq\lambda})}{\lambda} \]
for all \( {\lambda>0} \), and thus
\[ \int_0^\infty\lambda^{p-1} \mathbb{P}(\max_{s\in[0,t]}|M_s|\geq\lambda)\mathrm{d}\lambda \leq \int_0^\infty\lambda^{p-2} \mathbb{E}(|M_t|\mathbf{1}_{\max_{s\in[0,t]}|M_s|\geq\lambda})\mathrm{d}\lambda. \]
Now the Fubini-Tonelli theorem gives
\[ \int_0^\infty\lambda^{p-1}\mathbb{P}(\max_{s\in[0,t]}|M_s|\geq\lambda)\mathrm{d}\lambda =\mathbb{E}\int_0^{\max_{s\in[0,t]}|M_s|}\lambda^{p-1}\mathrm{d}\lambda =\frac{1}{p}\mathbb{E}(\max_{s\in[0,t]}|M_s|^p). \]
and similarly (here we need \( {p>1} \))
\[ \int_0^\infty\lambda^{p-2}\mathbb{E}(|M_t|\mathbf{1}_{\max_{s\in[0,t]}|M_s|\geq\lambda)})\mathrm{d}\lambda =\frac{1}{p-1}\mathbb{E}(|M_t|\max_{s\in[0,t]}|M_s|^{p-1}). \]
Combining all this gives
\[ \mathbb{E}(\max_{s\in[0,t]}|M_s|^p) \leq\frac{p}{p-1} \mathbb{E}(M_t\max_{s\in[0,t]}|M_s|^{p-1}). \]
But since the Hölder inequality gives
\[ \mathbb{E}(|M_t|\max_{s\in[0,t]}|M_s|^{p-1}) \leq\mathbb{E}(|M_t|^p)^{1/p}\mathbb{E}(\max_{s\in[0,t]}|M_s|^p)^{\frac{p-1}{p}}, \]
we obtain
\[ \mathbb{E}(\max_{s\in[0,t]}|M_s|^p) \leq\frac{p}{p-1}\mathbb{E}(|M_t|^p)^{1/p}\mathbb{E}(\max_{s\in[0,t]}|M_s|^p)^{\frac{p-1}{p}}. \]
Consequently, since \( {\mathbb{E}(\max_{s\in[0,t]}|M_s|^p)<\infty} \), we obtain the desired inequality.
Doob stopping theorem for sub-martingales. If \( {M} \) is a continuous sub-martingale and \( {S} \) and \( {T} \) are bounded stopping times such that \( {S\leq T,\quad M_S\in\mathrm{L}^1,\quad M_T\in\mathrm{L}^1} \), then
\[ \mathbb{E}(M_S)\leq\mathbb{E}(M_T). \]
Proof. We proceed as in the proof of the Doob stopping theorem for martingales, by assuming first that \( {S} \) and \( {T} \) take their values in the finite set \( {\{t_1,\ldots,t_n\}} \) where \( {t_1<\cdots<t_n} \). In this case \( {M_T} \) and \( {M_S} \) are in \( {\mathrm{L}^1} \) automatically. The inequality \( {S\leq T} \) gives \( {\mathbf{1}_{S\geq t}\leq\mathbf{1}_{T\geq t}} \) for all \( {t} \). Using this fact and the sub-martingale property of \( {M} \), we get
\[ \begin{array}{rcl} \mathbb{E}(M_S) &=&\mathbb{E}(M_0) +\mathbb{E}\Big(\sum_{k=1}^n\overbrace{\mathbb{E}(M_{t_k}-M_{t_{k-1}}\mid\mathcal{F}_{t_{k-1}})}^{\geq0}\mathbf{1}_{S\geq t_k}\Bigr)\\ &\leq&\mathbb{E}(M_0) +\mathbb{E}\Big(\sum_{k=1}^n\mathbb{E}(M_{t_k}-M_{t_{k-1}}\mid\mathcal{F}_{t_{k-1}})\mathbf{1}_{T\geq t_k}\Bigr)\\ &=&\mathbb{E}(M_T). \end{array} \]
More generally, when \( {S} \) and \( {T} \) are arbitrary bounded stopping times satisfying \( {S\leq T} \), we proceed by approximation as in the proof of the Doob stopping for martingales, using again the sub-martingale nature of \( {M} \) to get uniform integrability.
Doob stopping theorem for martingales. If \( {M} \) is a continuous martingale and \( {T:\Omega\rightarrow[0,+\infty]} \) is a stopping time then \( {{(M_{t\wedge T})}_{t\geq0}} \) is a martingale: for all \( {t\geq0} \) and \( {s\in[0,t]} \), we have
\[ M_{t\wedge T}\in\mathrm{L}^1 \quad\text{and}\quad \mathbb{E}(M_{t\wedge T}\mid\mathcal{F}_s)=M_{s\wedge T}. \]
Moreover, if \( {T} \) is bounded, or if \( {T} \) is almost surely finite and \( {{(M_{t\wedge T})}_{t\geq0}} \) is uniformly integrable (for instance dominated by an integrable random variable), then
\[ M_T\in\mathrm{L}^1 \quad\text{and}\quad \mathbb{E}(M_T)=\mathbb{E}(M_0). \]
Comments. The most important is that \( {{(M_{t\wedge T})}_{t\geq0}} \) is a martingale. We have always \( {\lim_{t\rightarrow\infty}M_{T\wedge t}\mathbf{1}_{T<\infty}=M_T\mathbf{1}_{T<\infty}} \) almost surely. When \( {T<\infty} \) almost surely we could use what we know on \( {M} \) and \( {T} \) to deduce by monotone or dominated convergence that this holds in \( {\mathrm{L}^1} \), giving \( {\mathbb{E}(M_T)=\mathbb{E}(\lim_{t\rightarrow\infty}M_{t\wedge T})=\lim_{t\rightarrow\infty}\mathbb{E}(M_{t\wedge T})=\mathbb{E}(M_0)} \).
The theorem states that this is automatically the case when \( {T} \) is bounded or when \( {M^T} \) is uniformly integrable. Furthermore, if \( {M^T} \) is uniformly integrable then it can be shown that \( {M_\infty} \) exists, giving a sense to \( {M_T} \) even on \( {\{T=\infty\}} \), and then \( {\mathbb{E}(M_T)=\mathbb{E}(M_0)} \).
Proof. Let assume first that \( {T} \) takes a finite number of values \( {t_1<\cdots<t_n} \). Let us show that \( {M_T\in\mathrm{L}^1} \) and \( {\mathbb{E}(M_T)=\mathbb{E}(M_0)} \). We have \( {M_T=\sum_{k=1}^nM_{t_k}\mathbf{1}_{T=t_k}\in\mathrm{L}^1} \), and moreover, using
\[ \{T\geq t_k\}=(\cup_{i=1}^{k-1}\{T=t_i\})^c\in\mathcal{F}_{t_{k-1}}, \]
and the martingale property \( {\mathbb{E}(M_{t_k}-M_{t_{k-1}}\mid\mathcal{F}_{t_{k-1}})=0} \), for all \( {k} \), we get
\[ \mathbb{E}(M_T) =\mathbb{E}(M_0) +\mathbb{E}\Big(\sum_{k=1}^n\mathbb{E}(M_{t_k}-M_{t_{k-1}}\mid\mathcal{F}_{t_{k-1}})\mathbf{1}_{T\geq t_k}\Bigr) =\mathbb{E}(M_0). \]
Suppose now that \( {T} \) takes an infinite number of values but is bounded by some constant \( {C} \). For all \( {n\geq0} \), we approximate \( {T} \) by the piecewise constant random variable (discretization of \( {[0,C]} \)).
\[ T_n=C\mathbf{1}_{T=C}+\sum_{k=1}^{n}t_{k}\mathbf{1}_{t_{k-1}\leq T<t_{k}} \quad\text{where}\quad t_k=t_{n,k}=C\frac{k}{n}. \]
This is a stopping time since for all \( {t\geq0} \), \( {\{T_n\leq t\}=\{T_n\leq\lfloor t\rfloor\}\in\mathcal{F}_{\lfloor t\rfloor}} \), which reduces the problem to a discrete \( {t} \), and then for all integer \( {m\geq0} \), we have that \( {\{T_n=m\} =\varnothing\in\mathcal{F}_0} \) if \( {m\not\in\{t_{k}:1\leq k\leq n\}} \), while \( {\{T_n=m\}=\{T=C\}\in\mathcal{F}_C} \) if \( {m=C} \), and
\[ \{T_n=m\} =\{T<t_{k-1}\}^c\cap\{T<t_{k}\}\in\mathcal{F}_{t_{k}} \]
if \( {m=t_{k}} \), \( {1\leq k\leq n} \), where we used the fact that for all \( {t\geq0} \),
\[ \{T=t\}=\{T\leq t\}\cap\{T<t\}^c=\{T\leq t\}\cap\cap_{r=1}^\infty\{T>t-1/r\}\in\mathcal{F}_t. \]
Since \( {T_n} \) takes a finite number of values, the previous step gives \( {\mathbb{E}(M_{T_n})=\mathbb{E}(M_0)} \). On the other hand, almost surely, \( {T_n\rightarrow T} \) as \( {n\rightarrow\infty} \). Since \( {M} \) is continuous, it follows that almost surely \( {M_{T_n}\rightarrow M_T} \) as \( {n\rightarrow\infty} \). Let us show now that \( {{(M_{T_n})}_{n\geq1}} \) is uniformly integrable. Since for all \( {n\geq0} \), \( {T_n} \) takes its values in a finite set \( {t_1<\cdots<t_{m_n}\leq C} \), the martingale property and the Jensen inequality give, for all \( {R>0} \),
\[ \begin{array}{rcl} \mathbb{E}(|M_C|\mathbf{1}_{|M_{T_n}|\geq R}) &=&\sum_k\mathbb{E}(|M_C|\mathbf{1}_{|M_{t_k}|\geq R,T_n=t_k})\\ &=&\sum_k\mathbb{E}(\mathbb{E}(|M_C|\mid\mathcal{F}_{t_k})\mathbf{1}_{|M_{t_k}|\geq R,T_n=t_k})\\ &\geq&\sum_k\mathbb{E}(|\mathbb{E}(M_C\mid\mathcal{F}_{t_k})|\mathbf{1}_{|M_{t_k}|\geq R,T_n=t_k})\\ &=&\sum_k\mathbb{E}(|M_{t_k}|\mathbf{1}_{|M_{t_k}|\geq R,T_n=t_k})\\ &=&\mathbb{E}(|M_{T_n}|\mathbf{1}_{|M_{T_n}|\geq R}). \end{array} \]
Now \( {M} \) is continuous and thus locally bounded, and \( {M_C\in\mathrm{L}^1} \), thus, by dominated convergence,
\[ \sup_n\mathbb{E}(|M_{T_n}|\mathbf{1}_{|M_{T_n}|>R}) \leq\mathbb{E}(|M_C|\mathbf{1}_{\sup_{s\in[0,C]}|M_s|\geq R}) \underset{R\rightarrow\infty}{\longrightarrow}0. \]
Therefore \( {{(M_{T_n})}_{n\geq0}} \) is uniformly integrable. As a consequence
\[ \overset{\mathrm{a.s.}}{\lim_{n\rightarrow\infty}}M_{T_n}=M_T\in\mathrm{L}^1 \quad\text{and}\quad \mathbb{E}(M_T)=\lim_{n\rightarrow\infty}\mathbb{E}(M_{T_n})=\mathbb{E}(M_0). \]
Let us suppose now that \( {T} \) is an arbitrary stopping time. For all \( {0\leq s\leq t} \) and \( {A\in\mathcal{F}_s} \), the random variable \( {S=s\mathbf{1}_A+t\mathbf{1}_{A^c}} \) is a (finite) stopping time, and what precedes for the finite stopping time \( {t\wedge T\wedge S} \) gives \( {M_{t\wedge T\wedge S}\in\mathrm{L}^1} \) and \( {\mathbb{E}(M_{t\wedge T\wedge S})=\mathbb{E}(M_0)} \). Now, using the definition of \( {S} \), we have
\[ \mathbb{E}(M_0) =\mathbb{E}(M_{t\wedge T\wedge S}) =\mathbb{E}(\mathbf{1}_AM_{s\wedge T}) +\mathbb{E}(\mathbf{1}_{A^c}M_{t\wedge T}) =\mathbb{E}(\mathbf{1}_A(M_{s\wedge T}-M_{t\wedge T}))+\mathbb{E}(M_{t\wedge T}). \]
Since \( {\mathbb{E}(M_{t\wedge T})=\mathbb{E}(M_0)} \), we get the martingale property for \( {{(M_{t\wedge T})}_{t\geq0}} \), namely
\[ \mathbb{E}((M_{t\wedge T}-M_{s\wedge T})\mathbf{1}_A)=0. \]
Finally, suppose that \( {T<\infty} \) almost surely and \( {{(M_{t\wedge T})}_{t\geq0}} \) is uniformly integrable. The random variable \( {M_T} \) is well defined and \( {\lim_{t\rightarrow\infty}M_{t\wedge T}=M_T} \) almost surely because \( {M} \) is continuous. Furthermore, since \( {{(M_{t\wedge T})}_{t\geq0}} \) is uniformly integrable, it follows that \( {M_T\in\mathrm{L}^1} \) and \( {\lim_{t\rightarrow\infty}M_{t\wedge T}=M_T} \) in \( {\mathrm{L}^1} \). In particular \( {\mathbb{E}(M_0)\underset{\forall t}{=}\mathbb{E}(M_{t\wedge T})=\lim_{t\rightarrow\infty}\mathbb{E}(M_{t\wedge T})=\mathbb{E}(M_T)} \). Further reading in the same spirit.
- Concentration Inequalities for Sums and Martingales
Bernard Bercu, Bernard Delyon, and Emmanuel Rio, Springer (2015).