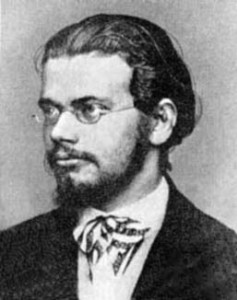
Recently a friend of mine asked about finding a good reason to explain the presence of the Boltzmann-Shannon entropy here and there in mathematics. Well, a vague answer is to simply say that the logarithm is already in many places, waiting for a nice interpretation. A bit less vaguely, here are some concrete fundamental formulas involving the Boltzmann-Shannon entropy \( {\mathcal{S}} \) also denoted \( {-H} \).
Combinatorics. If \( {n=n_1+\cdots+n_r} \) and \( {\lim_{n\rightarrow\infty}\frac{(n_1,\ldots,n_r)}{n}=(p_1,\ldots,p_r)} \) then
\[ \frac{1}{n}\log\binom{n}{n_1,\ldots,n_r} =\frac{1}{n}\log\frac{n!}{n_1!\cdots n_r!} \underset{n\rightarrow\infty}{\longrightarrow} -\sum_{k=1}^r p_k\log(p_k)=:\mathcal{S}(p_1,\ldots,p_r). \]
Also \( {\binom{n}{n_1,\ldots,n_r}\approx e^{-nH(p_1,\ldots,p_r)}} \) when \( {n\gg1} \) and \( {\frac{(n_1,\ldots,n_r)}{n}\approx (p_1,\ldots,p_r)} \). Wonderful!
Volumetrics. In terms of microstates and macrostate we also have
\[ \inf_{\varepsilon>0} \varlimsup_{n\rightarrow\infty} \frac{1}{n} \log\left| \left\{ f:\{1,\ldots,n\}\rightarrow\{1,\ldots,r\}: \max_{1\leq k\leq r}\left|\frac{f^{-1}(k)}{n}-p_k\right|<\varepsilon \right\}\right| =\mathcal{S}(p_1,\ldots,p_r). \]
This formula can be related to the Sanov Large Deviations Principle, some sort of refinement of the strong Law of Large Numbers.
Maximization. If \( {\displaystyle\int\!V(x)\,f(x)\,dx=\int\!V(x)f_\beta(x)\,dx} \) with \( {f_\beta(x)=\frac{e^{-\beta V(x)}}{Z_\beta}} \) then
\[ \mathcal{S}(f_\beta) - \mathcal{S}(f) =\int\!\frac{f}{f_\beta}\log\frac{f}{f_\beta}f_\beta\,dx \geq\left(\int\!\frac{f}{f_\beta}f_\beta\,dx\right)\log\left(\int\!\frac{f}{f_\beta}f_\beta\,dx\right)=0. \]
This formula plays an important role in statistical physics and in Bayesian statistics.
Legendre transform is log-Laplace. If \( {\mu} \) and \( {\nu} \) are probability measures then
\[ \sup_{\substack{g\geq0\\\int g\mathrm{d}\mu=1}} \Bigr\{\int fg\mathrm{d}\mu-\int f\log f\mathrm{d}\mu\Bigr\} =\log\int\mathrm{e}^f\mathrm{d}\mu \quad\text{where}\quad f:=\frac{\mathrm{d}\nu}{\mathrm{d}\mu}. \]
and the reverse identity by convex duality
\[ \int f\log f\mathrm{d}\mu =\sup_{\int g\mathrm{d}\mu=1} \Bigr\{\int fg\mathrm{d}\mu-\log\int\mathrm{e}^g\mathrm{d}\mu\Bigr\}. \]
Involved notably in large deviations theory and functional inequalities.
Likelihood. If \( {X_1,X_2,\ldots} \) are i.i.d. r.v. on \( {\mathbb{R}^d} \) with density \( {f} \) then
\[ L(f;X_1,\ldots,X_n) =\frac{1}{n}\log(f(X_1,\ldots,X_n)) \overset{a.s.}{\underset{n\rightarrow\infty}{\longrightarrow}} \int\!f\log(f)\,dx=:-\mathcal{S}(f). \]
This formula allows to reinterpret the maximum likelihood estimator as a minimum contrast estimator for the Kullback-Leibler divergence or relative entropy. It is also at the heart of Shannon coding theorems in information theory.
\( {L^p} \) norms. If \( {f\geq0} \) then
\[ \partial_{p=1}\left\Vert f\right\Vert_p^p =\partial_{p=1}\int\!e^{p\log(f)}\,dx =\int\!f\log(f)\,dx =-\mathcal{S}(f). \]
This formula is at the heart of a famous theorem of Leonard Gross which relates the hypercontractivity of ergodic Markov semigroups with a logarithmic Sobolev inequality for the invariant measure of the semigroup.
Fisher information. If \( {\partial_t f_t(x)=\Delta f_t(x)} \) then by integration by parts
\[ \partial_t\mathcal{S}(f_t) =-\int\!\log(f_t)\,\Delta f_t\,dx =\int\!\frac{\left|\nabla f_t\right|^2}{f_t}\,dx =\mathcal{F}(f_t). \]
This formula, attribued to de Bruijn, is at the heart of the analysis and geometry of heat kernels, diffusion processes, and gradient flows in partial differential equations.
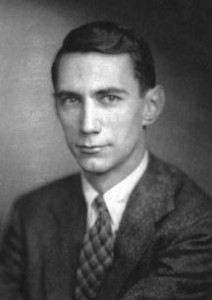