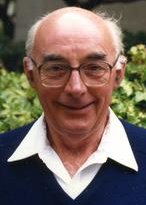
This post concerns an inequality regarding Poisson approximation, due to Lucien Le Cam (1924 - 2000). The total variation distance between two probability measures \( {\mu} \) and \( {\nu} \) on \( {\mathbb{N}} \) is given by
\[ d_{TV}(\mu,\nu) =\sup_{A\subset\mathbb{N}}|\mu(A)-\nu(A)| =\frac{1}{2}\sum_{k\in\mathbb{N}}|\mu_k-\nu_k|. \]
Weak convergence of probability measures on \( {\mathbb{N}} \) is equivalent to convergence in total variation. On non countable spaces, the convergence in total variation is in general stronger. If \( {\mu_1,\ldots,\mu_n} \) and \( {\nu_1,\ldots,\nu_n} \) are probability measures on \( {\mathbb{N}} \) then by induction we get the sub-additive bound
\[ d_{TV}(\mu_1*\cdots*\mu_n,\nu_1*\cdots*\nu_n) \leq d_{TV}(\mu_1,\nu_1)+\cdots+d_{TV}(\mu_n,\nu_n). \]
Le Cam's inequality allows to bound the total variation distance between the law of the sum of independent Bernoulli random variables and the Poisson law of the same mean. In all the sequel, \( {\beta_p} \) is the Bernoulli distribution \( {p\delta_1+(1-p)\delta_0} \) with \( {0\leq p\leq 1} \). Let us denote by \( {\pi_{\lambda}} \) the Poisson law of mean \( {\lambda} \). The elementary bound \( {d_{TV}(\beta_p,\pi_p)\leq p^2} \) used with the sub-additive bound above leads to Le Cam's inequality:
Theorem 1 (Le Cam's inequality)
\[ d_{TV}(\beta_{p_1}*\cdots*\beta_{p_n},\pi_{p_1+\cdots+p_n}) \leq p_1^2+\cdots+p_n^2. \]
Le Cam's inequality generalizes the Poisson approximation of the binomial distribution since if \( {p_1=\cdots=p_n=p} \) then \( {\beta_{p_1}*\cdots*\beta_{p_n}=\beta_p^{*n}=\mathrm{Binom}(n,p)} \). More generally, using Le Cam's inequality with the \( {L^2-L^1-L^\infty} \) bound \( {p_1^2+\cdots+p_n^2\leq (p_1+\cdots+p_n)\max_{1\leq i\leq n}p_i} \) provides immediately the so called law of small numbers: if we have
- convergence of the mean: \( {\lambda:=\sum_{n=1}^\infty p_n<\infty} \)
- dispersion of the bits: \( {\lim_{n\rightarrow\infty}\max_{1\leq i\leq n}p_i=0} \)
then \( {\beta_{p_1}*\cdots*\beta_{p_n}} \) converges weakly as \( {n\rightarrow\infty} \) to \( {\pi_\lambda} \). The convergence holds also in the Wasserstein distance \( {W_1} \) i.e. weak convergence + convergence of the first moment. In the same spirit, we have Chen's inequality:
Theorem 2 (Chen's inequality)
\[ d_{TV}(\beta_{p_1}*\cdots*\beta_{p_n},\pi_{p_1+\cdots+p_n}) \leq (1-e^{-(p_1+\cdots+p_n)})\frac{p_1^2+\cdots+p_n^2}{p_1+\cdots+p_n}. \]
This inequality can be found in the works of Barbour and Eagleson, based on the work of Louis Chen, a student of Charles Stein, who should not be confused with Elias Stein. The proof relies on Poisson integration by parts, following the method developped by Stein for the Gaussian in the central limit theorem. For more details, one may take a look at the paper by Steele and the book by Barbour, Holst, and Janson. You may also read the recent article by Chen and Roellin. Chen's inequality is stronger than Le Cam's inequality since \( {1-e^{-u} \leq u} \) for all \( {u\geq0} \).
Chen's inequality is useful even in situations where the mean \( {p_1+\cdots+p_n} \) of \( {\beta_{p_1}*\cdots*\beta_{p_n}} \) does not converge as \( {n\rightarrow\infty} \). Namely, if for instance \( {p_n=1/n} \) for every \( {n\geq1} \) then the right hand side in Chen's inequality is \( {O(1/\log(n))} \) and thus \( {\beta_{p_1}*\cdots*\beta_{p_n}} \) gets closer and closer to a Poisson law of mean \( {O(\log(n))} \) as \( {n} \) increases. This is helpful for instance for the study of various aspects of large random graphs, and for the derivation of the asymptotic normality of the number of occupied tables in the chineese restaurant process, a famous model of random partitions.
Various versions of Chen's inequality are available, where the total variation is replaced by the relative entropy or by a Wasserstein distance. The presence of a norm ratio in the right hand side of Chen's inequality is not surprising. Norms ratios allow to quantify sparsity or localization, as for instance in Berry-Esseen theorems.
Leave a Comment